Improving semantic information retrieval by combining possibilistic networks, vector space model and pseudo-relevance feedback
JOURNAL OF INFORMATION SCIENCE(2023)
摘要
To improve the performance of information retrieval systems (IRSs), we propose in this article a novel approach that enriches the user's queries with new concepts. Indeed, query expansion is one of the best methods that plays an important role in improving searches for a better semantic information retrieval. The proposed approach in this study combines possibilistic networks (PNs), the vector space model (VSM) and pseudo-relevance feedback (PRF) to evaluate and add relevant concepts to the initial index of the user's query. First, query expansion is performed using PN, VSM and domain knowledge. PRF is then exploited to enrich, in a second round, the user's query by applying the same approach used in the first expansion step. To evaluate the performance of the developed system, denoted conceptual information retrieval model (CIRM), several experiments of query expansion are performed. The experiments carried out on the OHSUMED and Clinical Trials corpora showed that using the two measures of possibility and necessity combined the cosinus similarity and PRF improves the query expansion process. Indeed, the improvement rate of our approach compared with the baseline is +28, 49% in terms of P@5.
更多查看译文
关键词
Biomedical domain, information retrieval, possibilistic networks, pseudo-relevance feedback, query enrichment, query expansion, VSM
AI 理解论文
溯源树
样例
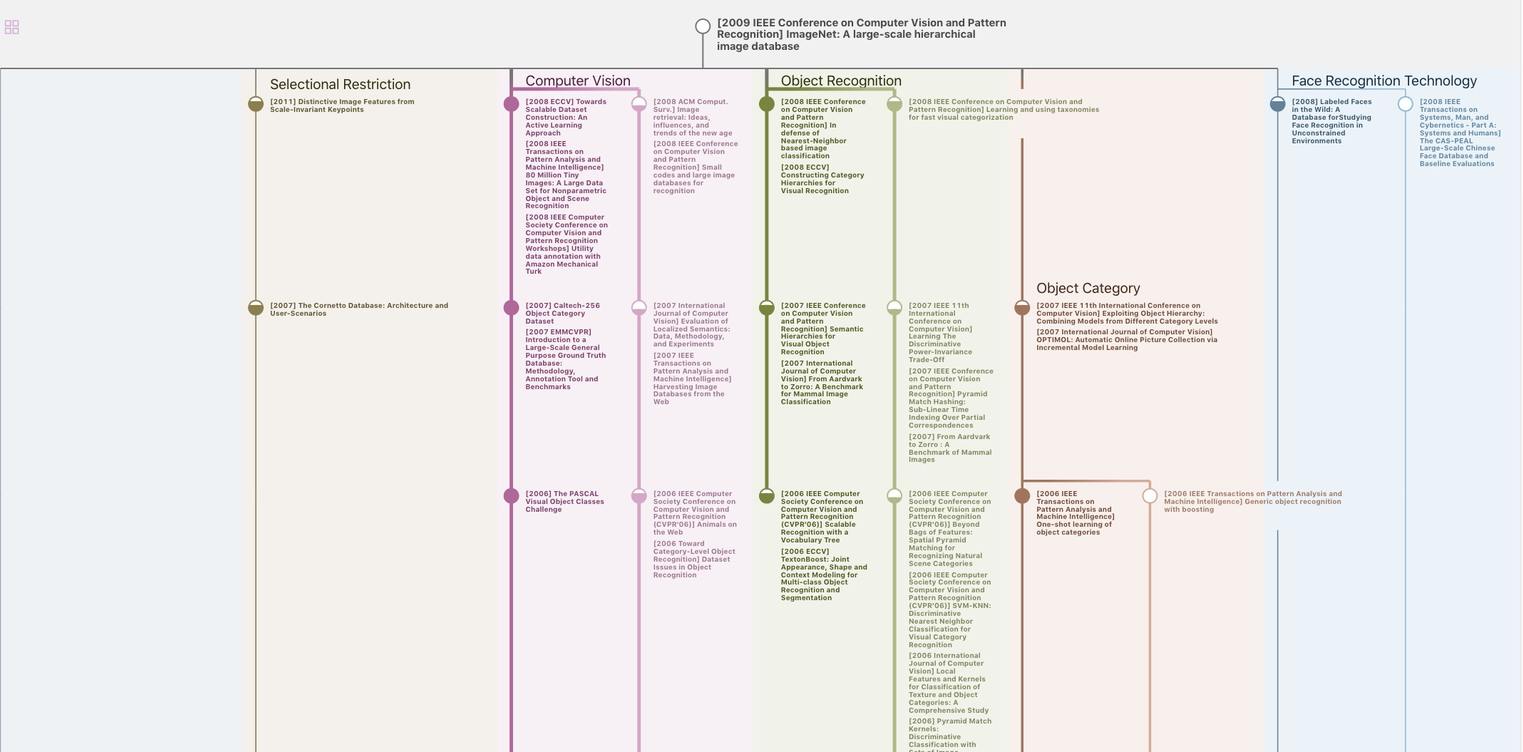
生成溯源树,研究论文发展脉络
Chat Paper
正在生成论文摘要