Observer studies of image quality of denoising reduced-count cardiac single photon emission computed tomography myocardial perfusion imaging by three-dimensional Gaussian post-reconstruction filtering and deep learning
JOURNAL OF NUCLEAR CARDIOLOGY(2023)
摘要
BackgroundThe aim of this research was to asses perfusion-defect detection-accuracy by human observers as a function of reduced-counts for 3D Gaussian post-reconstruction filtering vs deep learning (DL) denoising to determine if there was improved performance with DL.MethodsSPECT projection data of 156 normally interpreted patients were used for these studies. Half were altered to include hybrid perfusion defects with defect presence and location known. Ordered-subset expectation-maximization (OSEM) reconstruction was employed with the optional correction of attenuation (AC) and scatter (SC) in addition to distance-dependent resolution (RC). Count levels varied from full-counts (100%) to 6.25% of full-counts. The denoising strategies were previously optimized for defect detection using total perfusion deficit (TPD). Four medical physicist (PhD) and six physician (MD) observers rated the slices using a graphical user interface. Observer ratings were analyzed using the LABMRMC multi-reader, multi-case receiver-operating-characteristic (ROC) software to calculate and compare statistically the area-under-the-ROC-curves (AUCs).ResultsFor the same count-level no statistically significant increase in AUCs for DL over Gaussian denoising was determined when counts were reduced to either the 25% or 12.5% of full-counts. The average AUC for full-count OSEM with solely RC and Gaussian filtering was lower than for the strategies with AC and SC, except for a reduction to 6.25% of full-counts, thus verifying the utility of employing AC and SC with RC.ConclusionWe did not find any indication that at the dose levels investigated and with the DL network employed, that DL denoising was superior in AUC to optimized 3D post-reconstruction Gaussian filtering.
更多查看译文
关键词
SPECT,MPI,denoising,deep learning,observer study
AI 理解论文
溯源树
样例
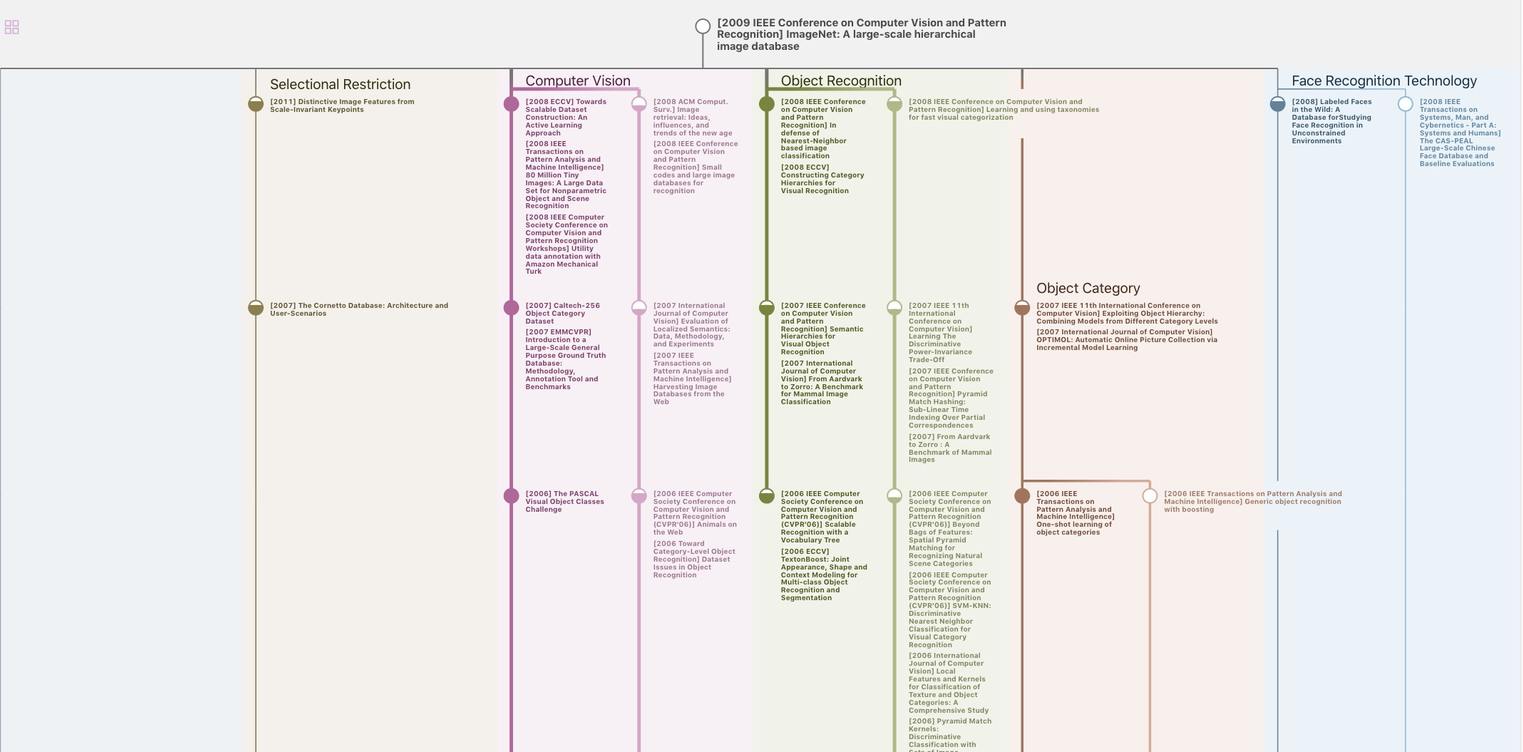
生成溯源树,研究论文发展脉络
Chat Paper
正在生成论文摘要