Deep Cross-Network Alignment with Anchor Node Pair Diverse Local Structure
ALGORITHMS(2023)
摘要
Network alignment (NA) offers a comprehensive way to build associations between different networks by identifying shared nodes. While the majority of current NA methods rely on the topological consistency assumption, which posits that shared nodes across different networks typically have similar local structures or neighbors, we argue that anchor nodes, which play a pivotal role in NA, face a more challenging scenario that is often overlooked. In this paper, we conduct extensive statistical analysis across networks to investigate the connection status of labeled anchor node pairs and categorize them into four situations. Based on our analysis, we propose an end-to-end network alignment framework that uses node representations as a distribution rather than a point vector to better handle the structural diversity of networks. To mitigate the influence of specific nodes, we introduce a mask mechanism during the representation learning process. In addition, we utilize meta-learning to generalize the learned information on labeled anchor node pairs to other node pairs. Finally, we perform comprehensive experiments on both real-world and synthetic datasets to confirm the efficacy of our proposed method. The experimental results demonstrate that the proposed model outperforms the state-of-the-art methods significantly.
更多查看译文
关键词
deep,cross-network
AI 理解论文
溯源树
样例
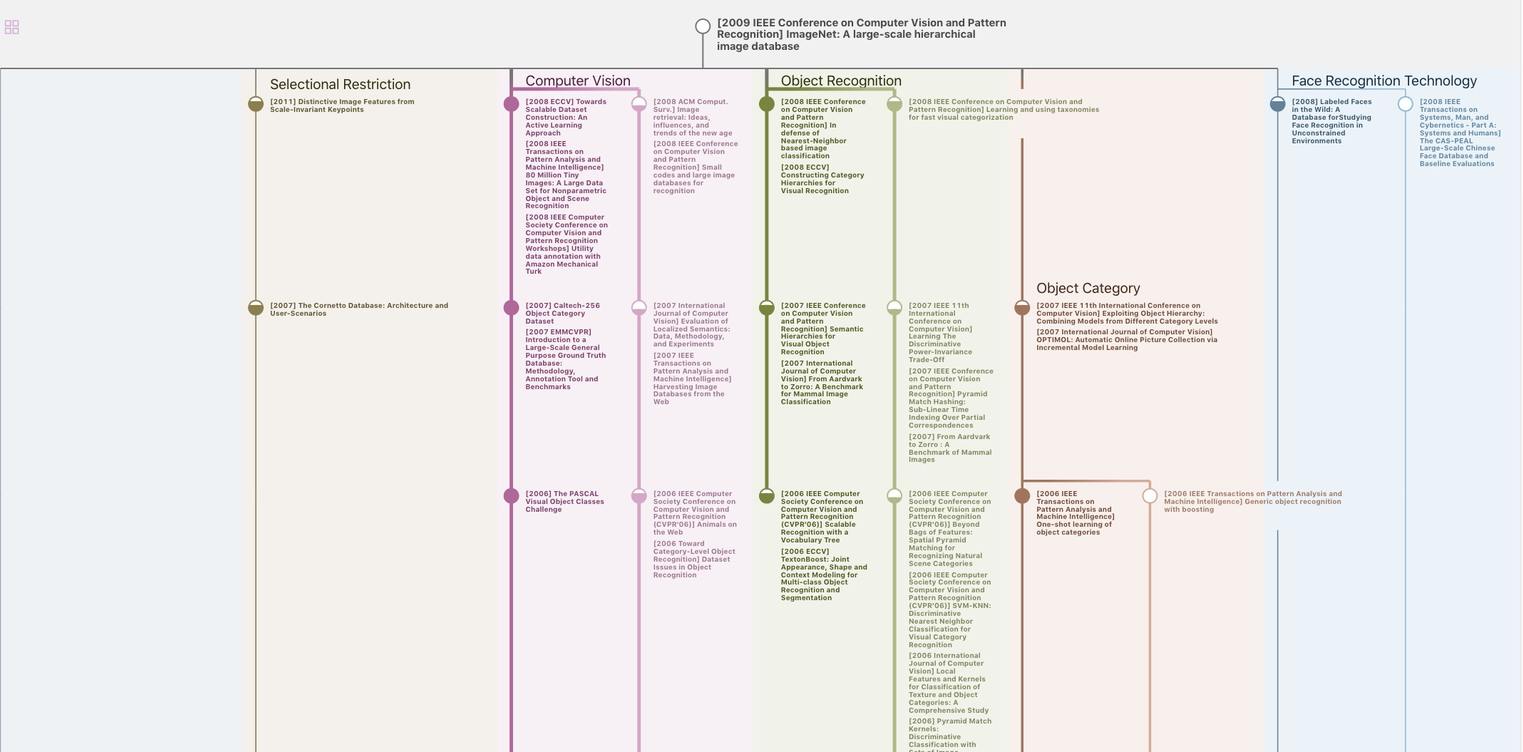
生成溯源树,研究论文发展脉络
Chat Paper
正在生成论文摘要