FedUA: An Uncertainty-Aware Distillation-Based Federated Learning Scheme for Image Classification.
Inf.(2023)
Abstract
Recently, federated learning (FL) has gradually become an important research topic in machine learning and information theory. FL emphasizes that clients jointly engage in solving learning tasks. In addition to data security issues, fundamental challenges in this type of learning include the imbalance and non-IID among clients' data and the unreliable connections between devices due to limited communication bandwidths. The above issues are intractable to FL. This study starts from the uncertainty analysis of deep neural networks (DNNs) to evaluate the effectiveness of FL, and proposes a new architecture for model aggregation. Our scheme improves FL's performance by applying knowledge distillation and the DNN's uncertainty quantification methods. A series of experiments on the image classification task confirms that our proposed model aggregation scheme can effectively solve the problem of non-IID data, especially when affordable transmission costs are limited.
MoreTranslated text
Key words
federated learning,model aggregation,knowledge distillation,uncertainty in deep neural networks
AI Read Science
Must-Reading Tree
Example
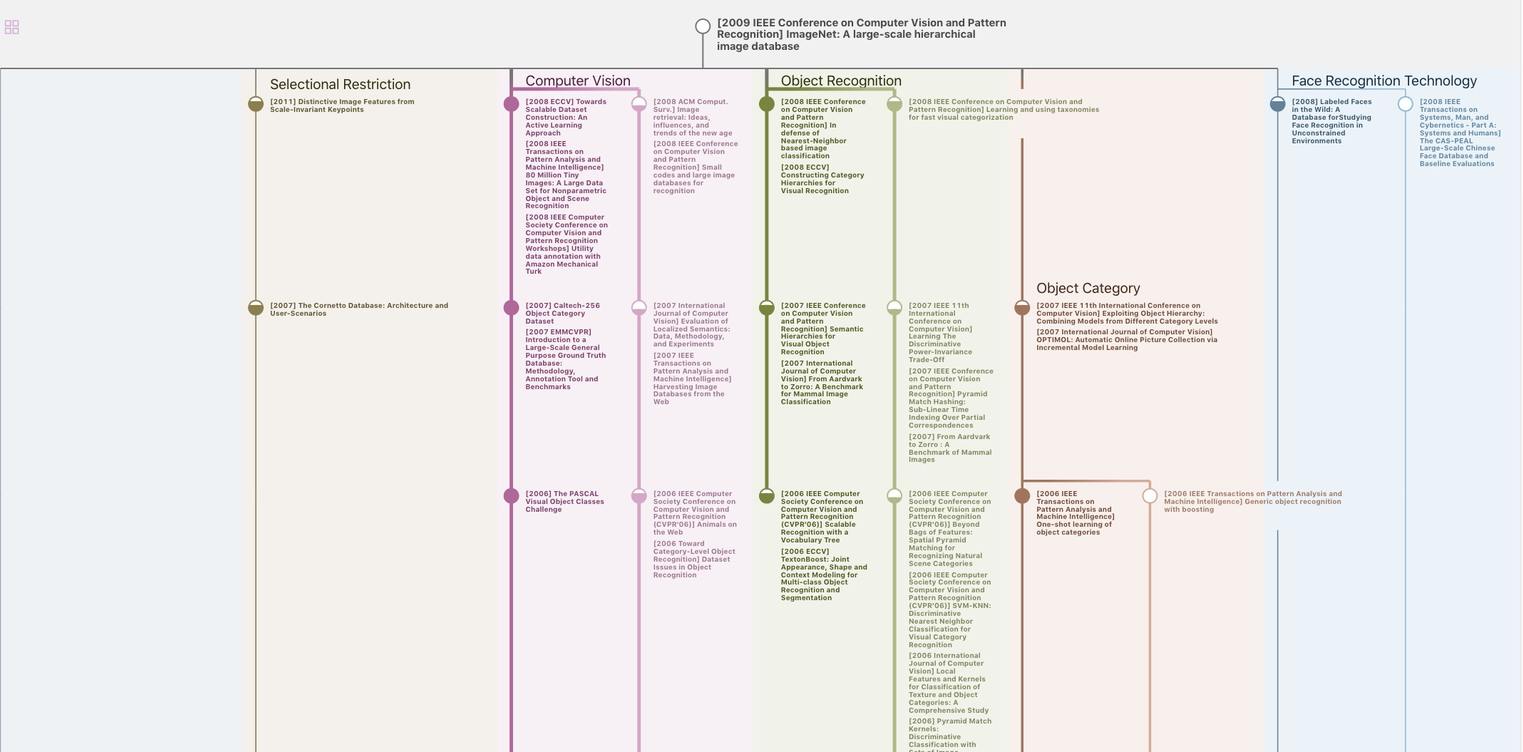
Generate MRT to find the research sequence of this paper
Chat Paper
Summary is being generated by the instructions you defined