SPL-Net: Spatial-Semantic Patch Learning Network for Facial Attribute Recognition with Limited Labeled Data
INTERNATIONAL JOURNAL OF COMPUTER VISION(2023)
摘要
Existing deep learning-based facial attribute recognition (FAR) methods rely heavily on large-scale labeled training data. Unfortunately, in many real-world applications, only limited labeled data are available, resulting in the performance deterioration of these methods. To address this issue, we propose a novel spatial-semantic patch learning network (SPL-Net), consisting of a multi-branch shared subnetwork (MSS), three auxiliary task subnetworks (ATS), and an FAR subnetwork, for attribute classification with limited labeled data. Considering the diversity of facial attributes, MSS includes a task-shared branch and four region branches, each of which contains cascaded dual cross attention modules to extract region-specific features. SPL-Net involves a two-stage learning procedure. In the first stage, MSS and ATS are jointly trained to perform three auxiliary tasks (i.e., a patch rotation task (PRT), a patch segmentation task (PST), and a patch classification task (PCT)), which exploit the spatial-semantic relationship on large-scale unlabeled facial data from various perspectives. Specifically, PRT encodes the spatial information of facial images based on self-supervised learning. PST and PCT respectively capture the pixel-level and image-level semantic information of facial images by leveraging a facial parsing model. Thus, a well-pretrained MSS is obtained. In the second stage, based on the pre-trained MSS, an FAR model is easily fine-tuned to predict facial attributes by requiring only a small amount of labeled data. Experimental results on challenging facial attribute datasets (including CelebA, LFWA, and MAAD) show the superiority of SPL-Net over several state-of-the-art methods in the case of limited labeled data.
更多查看译文
关键词
facial attribute recognition,learning,spl-net,spatial-semantic
AI 理解论文
溯源树
样例
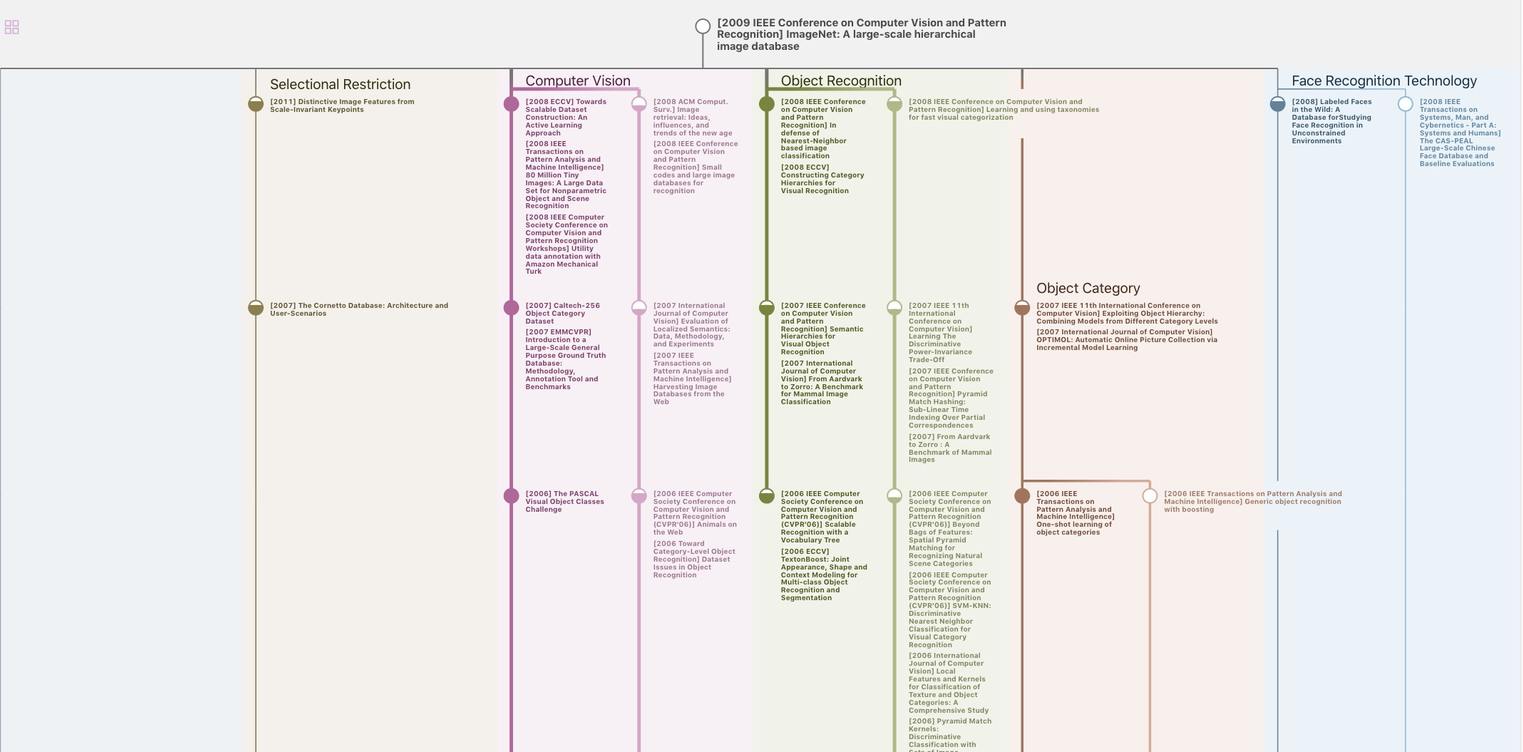
生成溯源树,研究论文发展脉络
Chat Paper
正在生成论文摘要