Improving PLMs for Graph-to-Text Generation by Relational Orientation Attention
NEURAL PROCESSING LETTERS(2023)
摘要
Pretrained language models (PLMs) with impressive performances in graph-to-text generation have recently been employed. However, linearized graph data will lead to the loss of triplet structure information and the problem of insufficient syntactic information when graph data are converted into sequence data by PLMs. These defects prevent PLMs from absorbing all the information that knowledge graphs hold and exerting their full potential in graph-to-text generation. To address these issues, we propose two targeted solutions. First, a relational orientation attention (ROA) module is proposed to incorporate triplet structure information into knowledge graph representations. During graph encoding, ROA establishes structural associations between entities and relations by fusing relevant relation information into entity representations. Second, the (knowledge subgraph, text) pairs are used to pretrain PLMs in text and triplet reconstruction tasks. Pretraining tasks with linearized graph data will enable PLMs to transfer learning more seamlessly between graphs and texts. The experiments with the WebNLG, WebQuestions, and PathQuestions datasets demonstrate that relational orientation attention and pretraining tasks (text and triplet reconstruction) can be implemented to capture triplet structure information and boost the learning ability of PLMs on structured data. Additional research reveals that PLMs equipped with designed approaches have superior few-shot learning capability.
更多查看译文
关键词
Graph-to-text generation,Pretrained language model,Relational orientation attention,Pretraining task
AI 理解论文
溯源树
样例
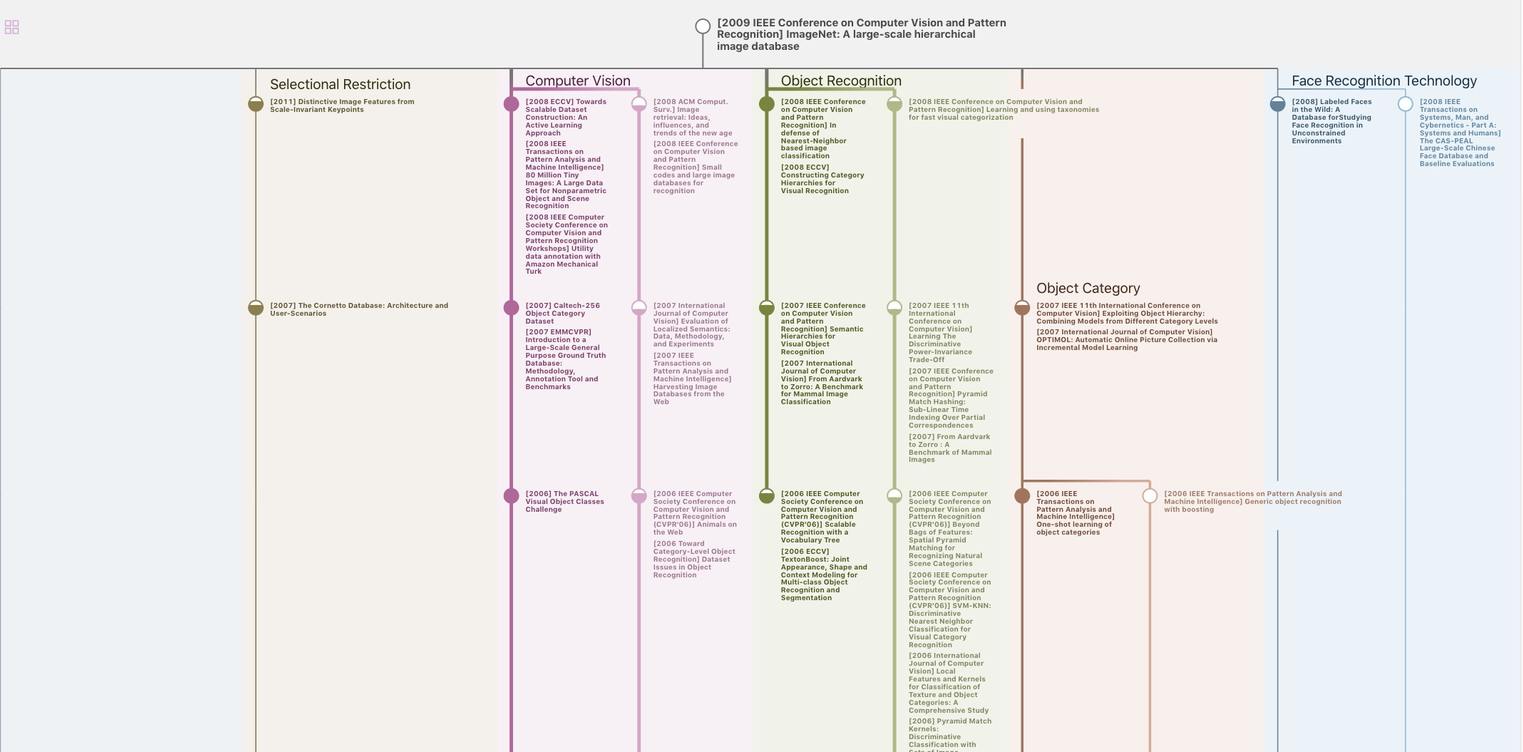
生成溯源树,研究论文发展脉络
Chat Paper
正在生成论文摘要