SFCNet: Deep Learning-based Lightweight Separable Factorized Convolution Network for Landslide Detection
JOURNAL OF THE INDIAN SOCIETY OF REMOTE SENSING(2023)
摘要
It is challenging to detect landslides automatically and accurately in today’s environment, yet these are essential in preventing and minimizing natural landslide threats. Despite developing numerous deep learning models for landslide detection, a lightweight framework for embedding landslide detection in mobile devices or hardware is yet to be devised. This paper proposes a lightweight deep learning model for pixel-based landslide detection called Separable Factorized Convolution Network (SFCNet). The proposed SFCNet's efficiency is observed in the study area, located on the eastern side of the Western Ghats in the Kodagu district, India, which is known for its landslide endeavor. SFCNet is a lightweight convolutional network that incorporates separable and factorized convolution. The separable convolution and factorized convolution are employed in the proposed SFCNet to lower the number of parameters and the computational complexity. In addition, the SFCNet's performance is examined under various scaling and folding instances. The results prove that the proposed SFCNet minimizes the number of parameters by over 95% compared to the existing deep CNN model. The proposed SFCNet's F1 score and accuracy on landslide detection are 98.34 and 98.36%, respectively. The susceptibility map of landslides for the study area is mapped from the probability of prediction of landslides by the SFCNet. From the SFCNet's performance on landslide detection and mapping, it is observed that it has significant scope for various applications in large area landslide identification. Therefore, it can be suggested for risk analysis and emergency rescue.
更多查看译文
关键词
Separable convolution,Factorized convolution,Kodagu landslide detection,Lightweight CNN,LandSat-8(OLI) satellite image
AI 理解论文
溯源树
样例
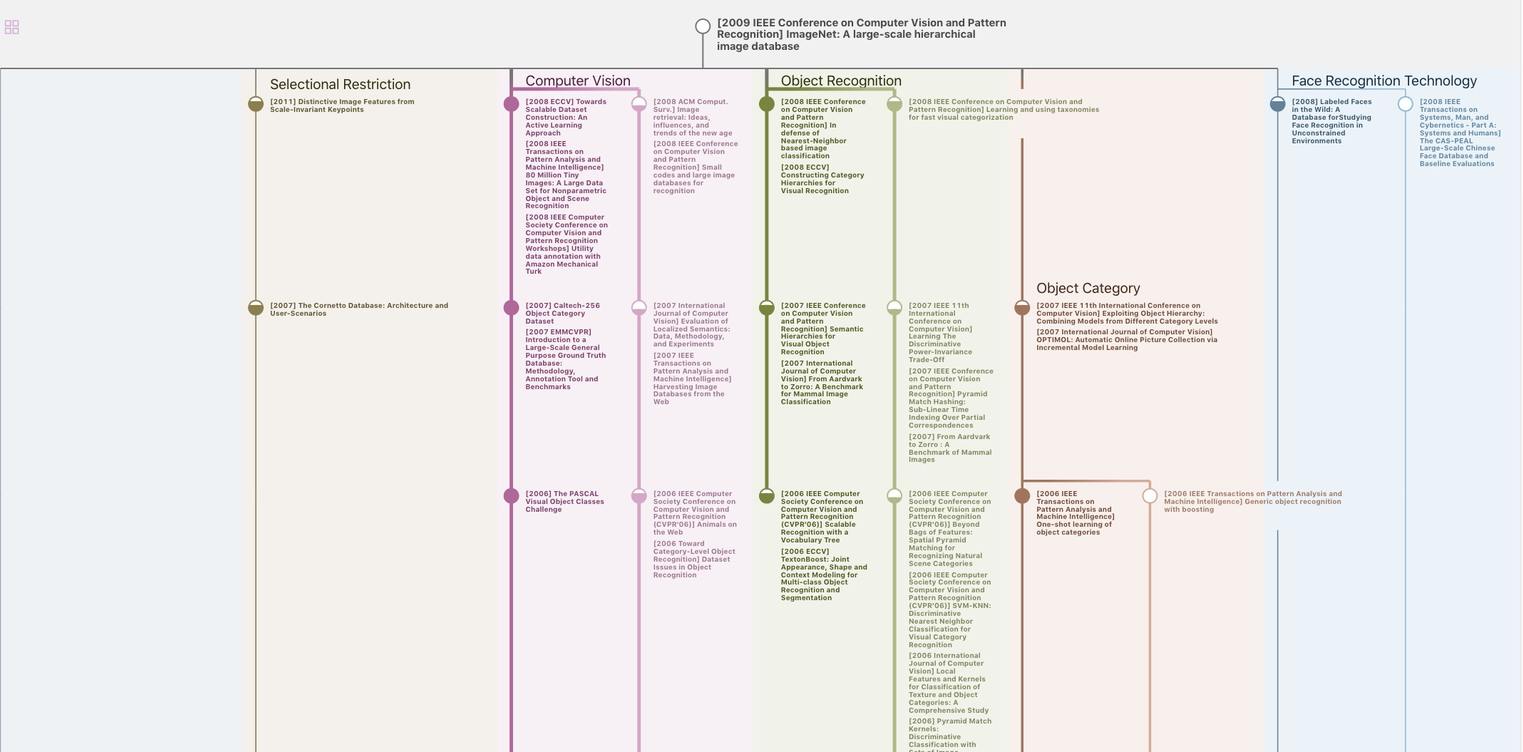
生成溯源树,研究论文发展脉络
Chat Paper
正在生成论文摘要