Zero-shot stance detection via multi-perspective contrastive with unlabeled data
INFORMATION PROCESSING & MANAGEMENT(2023)
摘要
Stance detection is to distinguish whether the text's author supports, opposes, or maintains a neutral stance towards a given target. In most real-world scenarios, stance detection needs to work in a zero-shot manner, i.e., predicting stances for unseen targets without labeled data. One critical challenge of zero-shot stance detection is the absence of contextual information on the targets. Current works mostly concentrate on introducing external knowledge to supplement information about targets, but the noisy schema-linking process hinders their performance in practice. To combat this issue, we argue that previous studies have ignored the extensive target -related information inhabited in the unlabeled data during the training phase, and propose a simple yet efficient Multi-Perspective Contrastive Learning Framework for zero-shot stance detection. Our framework is capable of leveraging information not only from labeled data but also from extensive unlabeled data. To this end, we design target-oriented contrastive learning and label-oriented contrastive learning to capture more comprehensive target representation and more distinguishable stance features. We conduct extensive experiments on three widely adopted datasets (from 4870 to 33,090 instances), namely SemEval-2016, WT-WT, and VAST. Our framework achieves 53.6%, 77.1%, and 72.4% macro-average F1 scores on these three datasets, showing 2.71% and 0.25% improvements over state-of-the-art baselines on the SemEval-2016 and WT-WT datasets and comparable results on the more challenging VAST dataset.
更多查看译文
关键词
Stance detection,Contrastive learning,Unlabeled data,Zero-shot
AI 理解论文
溯源树
样例
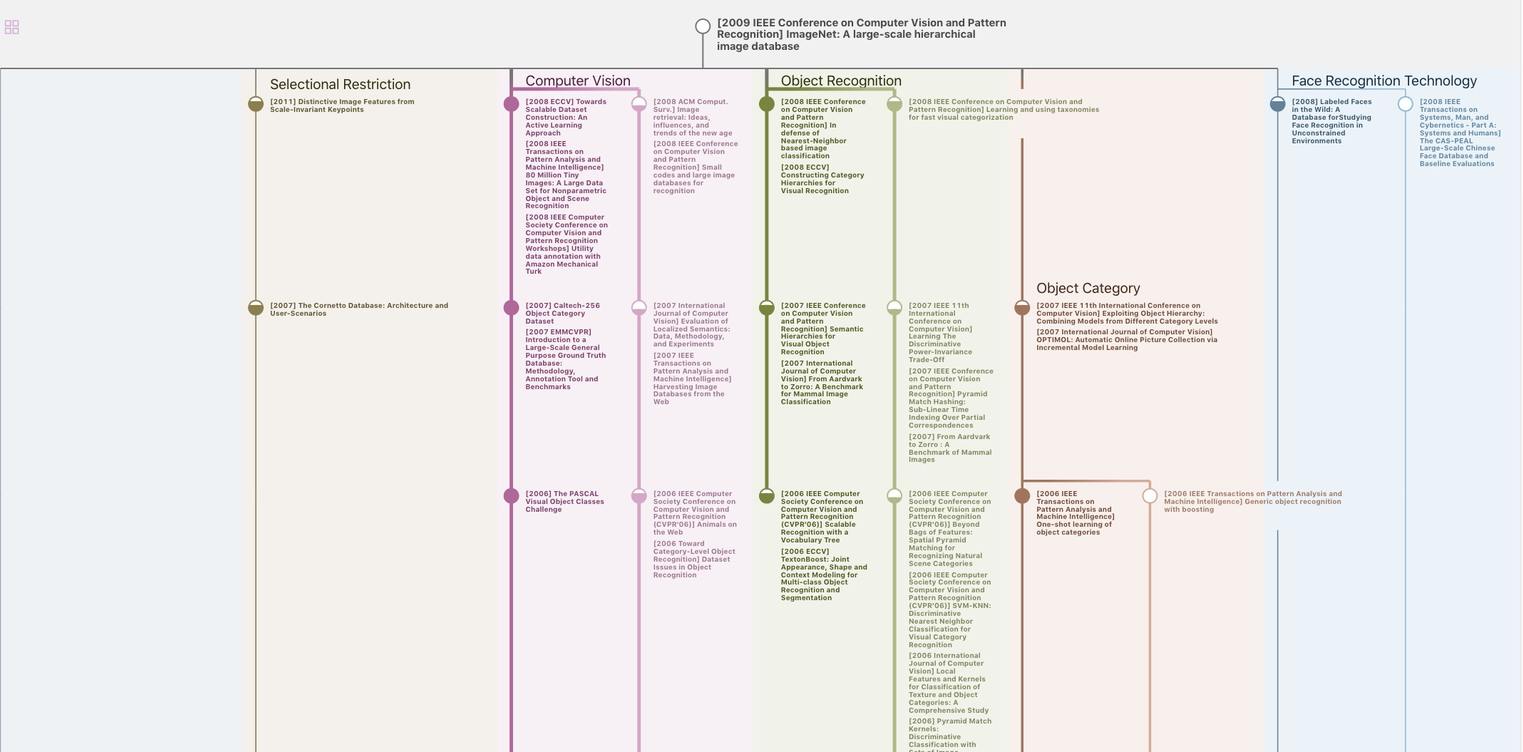
生成溯源树,研究论文发展脉络
Chat Paper
正在生成论文摘要