Prediction of prostate tumour hypoxia using pre-treatment MRI-derived radiomics: preliminary findings
RADIOLOGIA MEDICA(2023)
摘要
Purpose To develop a machine learning (ML) model based on radiomic features (RF) extracted from whole prostate gland magnetic resonance imaging (MRI) for prediction of tumour hypoxia pre-radiotherapy. Material and methods Consecutive patients with high-grade prostate cancer and pre-treatment MRI treated with radiotherapy between 01/12/2007 and 1/08/2013 at two cancer centres were included. Cancers were dichotomised as normoxic or hypoxic using a biopsy-based 32-gene hypoxia signature (Ragnum signature). Prostate segmentation was performed on axial T2-weighted (T2w) sequences using RayStation (v9.1). Histogram standardisation was applied prior to RF extraction. PyRadiomics (v3.0.1) was used to extract RFs for analysis. The cohort was split 80:20 into training and test sets. Six different ML classifiers for distinguishing hypoxia were trained and tuned using five different feature selection models and fivefold cross-validation with 20 repeats. The model with the highest mean validation area under the curve (AUC) receiver operating characteristic (ROC) curve was tested on the unseen set, and AUCs were compared via DeLong test with 95% confidence interval (CI). Results 195 patients were included with 97 (49.7%) having hypoxic tumours. The hypoxia prediction model with best performance was derived using ridge regression and had a test AUC of 0.69 (95% CI: 0.14). The test AUC for the clinical-only model was lower (0.57), but this was not statistically significant ( p = 0.35). The five selected RFs included textural and wavelet-transformed features. Conclusion Whole prostate MRI-radiomics has the potential to non-invasively predict tumour hypoxia prior to radiotherapy which may be helpful for individualised treatment optimisation.
更多查看译文
关键词
Prostate cancer,Hypoxia,MRI,Radiomics,Radiogenomics,Machine learning
AI 理解论文
溯源树
样例
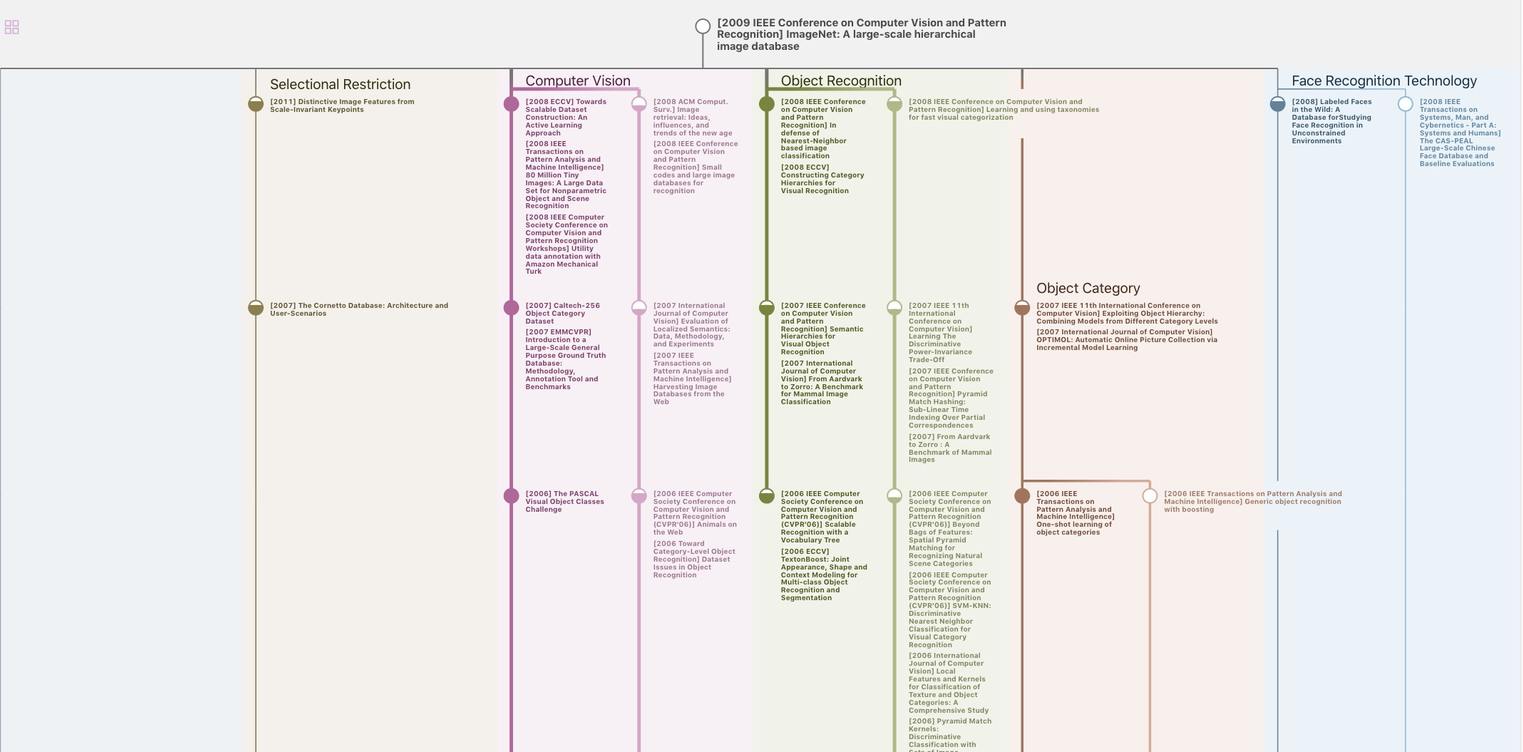
生成溯源树,研究论文发展脉络
Chat Paper
正在生成论文摘要