A comparison of synthetic data approaches using utility and disclosure risk measures
KOREAN JOURNAL OF APPLIED STATISTICS(2023)
摘要
This paper investigates synthetic data generation methods and their evaluation measures. There have been increasing demands for releasing various types of data to the public for different purposes. At the same time, there are also unavoidable concerns about leaking critical or sensitive information. Many synthetic data gener-ation methods have been proposed over the years in order to address these concerns and implemented in some countries, including Korea. The current study aims to introduce and compare three representative synthetic data generation approaches: Sequential regression, nonparametric Bayesian multiple imputations, and deep generative models. Several evaluation metrics that measure the utility and disclosure risk of synthetic data are also reviewed. We provide empirical comparisons of the three synthetic data generation approaches with respect to various eval-uation measures. The findings of this work will help practitioners to have a better understanding of the advantages and disadvantages of those synthetic data methods.
更多查看译文
关键词
synthetic data approaches,risk,utility
AI 理解论文
溯源树
样例
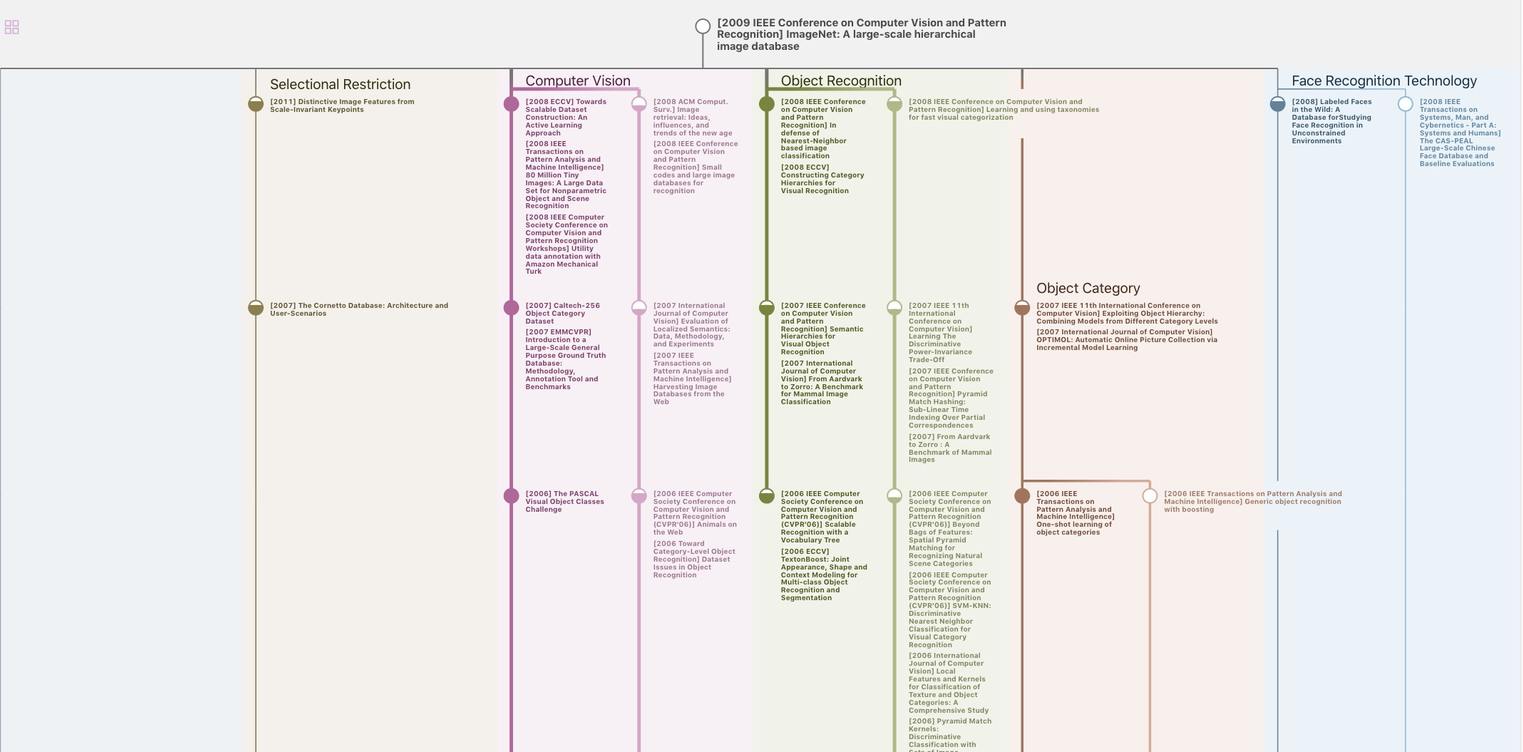
生成溯源树,研究论文发展脉络
Chat Paper
正在生成论文摘要