Striving for Sparsity: On Exact and Approximate Solutions in Regularized Structural Equation Models
STRUCTURAL EQUATION MODELING-A MULTIDISCIPLINARY JOURNAL(2023)
摘要
Regularized structural equation models have gained considerable traction in the social sciences. They promise to reduce overfitting by focusing on out-of-sample predictions and sparsity. To this end, a set of increasingly constrained models is fitted to the data. Subsequently, one of the models is selected, usually by means of information criteria. Current implementations of regularized structural equation models differ in their optimizers: Some use general purpose optimizers whereas others use specialized optimization routines. While both approaches often perform similarly, we show that they can produce very different results. We argue that in particular, the interaction between optimizer and selection criterion (e.g., BIC) contributes to these differences. We substantiate our arguments with an empirical demonstration and a simulation study. Based on these findings, we conclude that researchers should consider specialized optimizers whenever possible. To facilitate the implementation of such optimizers, we provide the R package lessSEM.
更多查看译文
关键词
Lasso,optimization,regularization,structural equation model
AI 理解论文
溯源树
样例
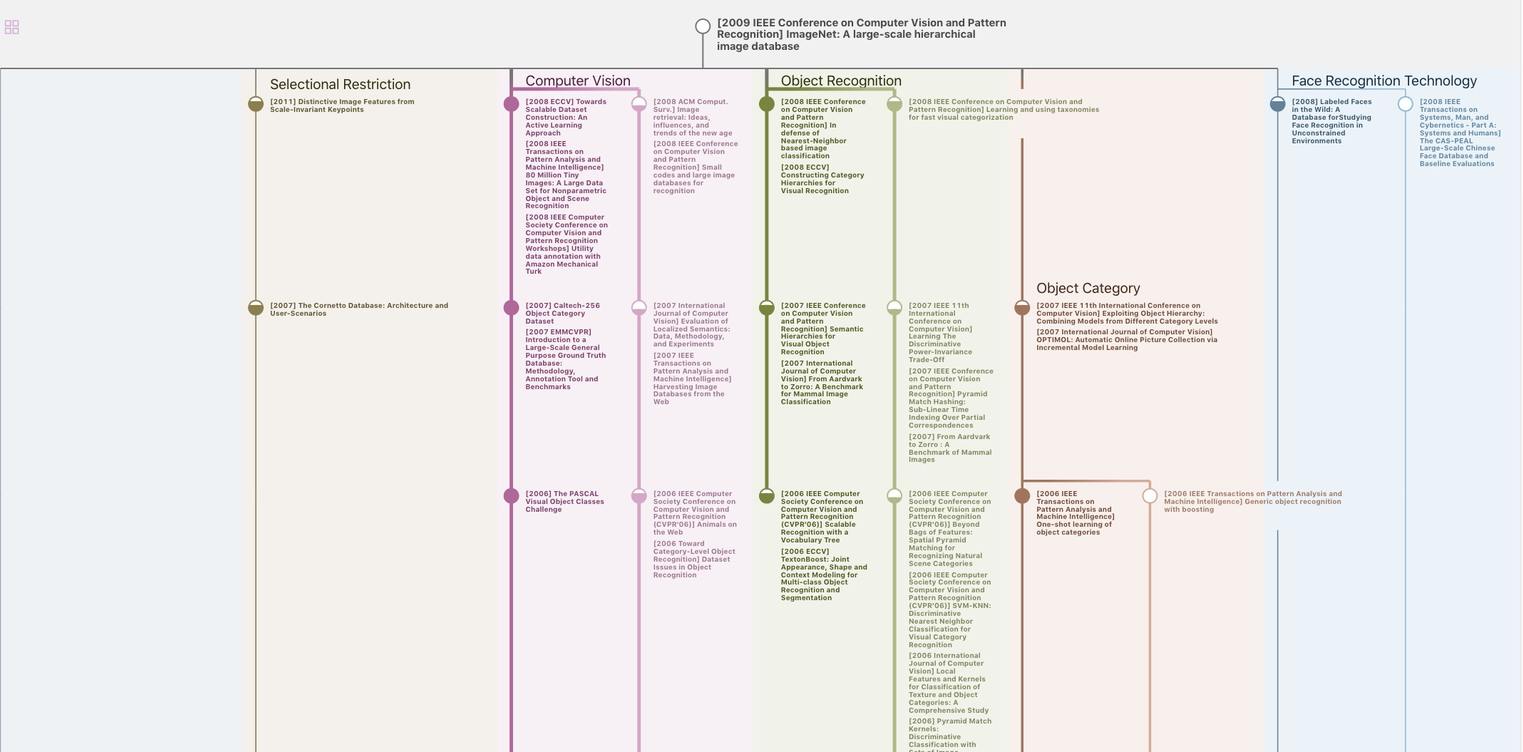
生成溯源树,研究论文发展脉络
Chat Paper
正在生成论文摘要