A sparse lightweight attention network for image super-resolution
VISUAL COMPUTER(2024)
摘要
Recently, deep learning methods have been widely applied to single image super-resolution reconstruction tasks and achieved great improvement in both quantitative and qualitative evaluations. However, most of the existing convolutional neural network-based methods commonly reduce the number of layers or channels to obtain lightweight model. These strategies may reduce the representation ability of informative features and degrade the network performance. To address this issue, we propose a sparse lightweight attention network (SLAN), a novel SISR algorithm to keep informative features between layers. Specially, a sparse attention feature fusion module with lightweight attention and sparse extracting modules is developed to expand feature extracting receptive field and enhance the informative feature extracting ability. To take advantage of the multi-level features and keep fewer multi-adds, cross fusion is used and demonstrated usefully. Extensive experimental results on public datasets demonstrate the superior performance of our proposed SLAN. The average PSNR(dB)/SSIM values of SLAN are about 0.04/0.0004, 0.06/0.0009, and 0.09/0.0018 larger than the competitors under scaling factors of x 2, x 3 and x 4, respectively. Our SLAN benefits from its model size and low computation cost and may be deployed on mobile platforms.
更多查看译文
关键词
Image super-resolution,Lightweight,Attention mechanism,Convolutional neural network
AI 理解论文
溯源树
样例
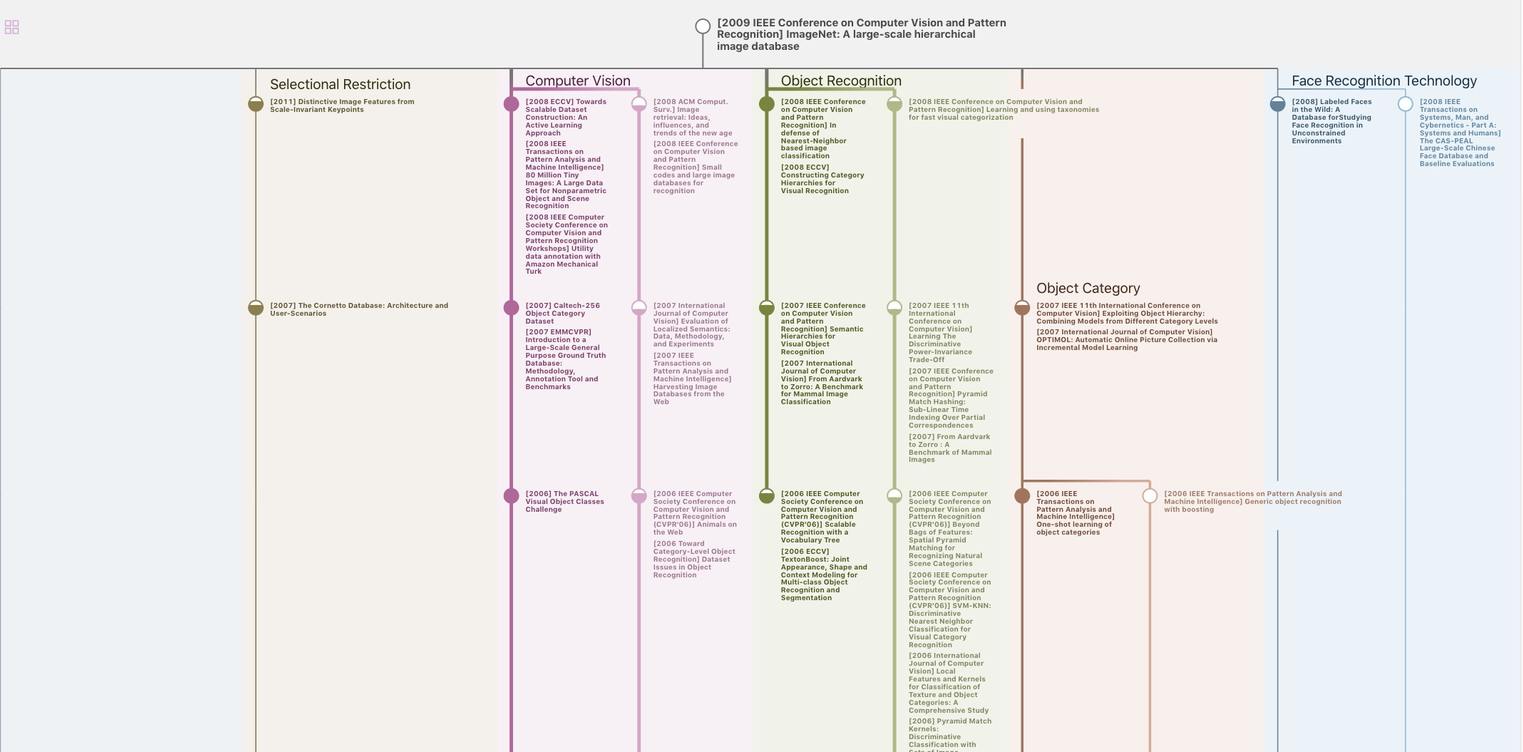
生成溯源树,研究论文发展脉络
Chat Paper
正在生成论文摘要