Machine learning prediction of groundwater heights from passive seismic wavefield
GEOPHYSICAL JOURNAL INTERNATIONAL(2023)
摘要
Most of water reservoirs are underground and therefore challenging to monitor. This is particularly the case of karst aquifers which knowledge is mostly based on sparse spatial and temporal observations. In this study, we propose a new approach, based on a supervised machine learning algorithm, the Random Forests, and continuous seismic noise records, that allows the prediction of the underground river water height. The study site is a karst aquifer in the Jura Mountains (France). An underground river is accessible through an artificial shaft and is instrumented by a hydrological probe. The seismic noise generated by the river is recorded by two broadband seismometers, located underground (20 m depth) and at the surface. The algorithm succeeds in predicting water height thanks to signal energy features. Even weak river-induced noise such as recorded at the surface can be detected and used by the algorithm. Its efficiency, expressed by the Nash-Sutcliffe criterion, is above 95 per cent and 53 per cent for data from the underground and surface seismic stations, respectively.
更多查看译文
关键词
Hydrogeophysics,Seismic noise,Machine learning,Time-series analysis
AI 理解论文
溯源树
样例
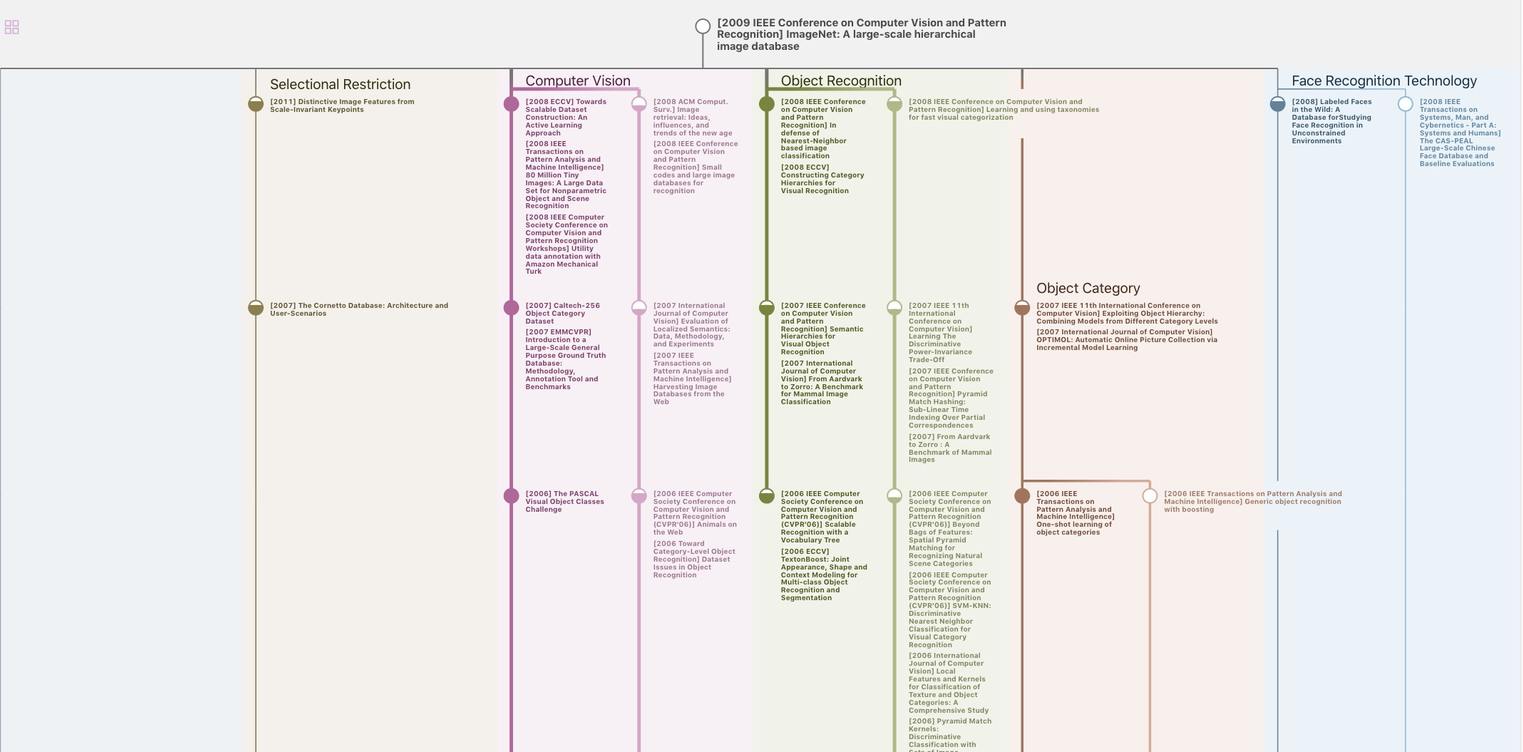
生成溯源树,研究论文发展脉络
Chat Paper
正在生成论文摘要