Music Performance Assessment: Noise in Judgments and Reliability of Measurements
PSYCHOLOGY OF AESTHETICS CREATIVITY AND THE ARTS(2023)
摘要
Individual taste plays an important role in assessing music performances. Aggregating ratings from multiple evaluators is commonly applied to overcome individual biases and to obtain near-objective judgments. However, high degrees of subjectivity in the evaluation of music performances inevitability lowers the agreement between music evaluators and the reliability of aggregated ratings. The present project aims at contributing to the research on music performance assessment by designing and implementing a novel procedure, the Multi-Perspective Assessment Protocol (MPAP) in music, for reliably measuring performance quality. The approach is inspired by the Noise Framework (Kahneman et al., 2021) and Generalizability Theory (G Theory; Brennan, 2001) and results show how interrater agreement can be conceptualized and operationalized efficiently in terms of rater-related variability in numerical judgments. In particular, the Generalizability and Dependability coefficients from G Theory seem well suited for providing useful information about the necessary sample size to ensure reliable performance score estimates. Moreover, the MPAP allowed to collect and compare data from different cohorts of evaluators, assessing performance quality from complementary viewpoints: aggregate judgments from expert evaluators were strongly correlated to those from a general sample of raters without expert experience, r = .786, p < .001. The latter sample achieved similar levels of reliability but with a larger sample size. In contrast, self-assessment scores from the performing musicians were very much unrelated to any peer-evaluation ratings.. In conclusion, the present study shows how recent advancements in theoretical frameworks and psychometric methodology provide a principled approach for increasing the quality and reliability of performance evaluation data.
更多查看译文
关键词
music performance assessment,noise,G theory,reliability,practice
AI 理解论文
溯源树
样例
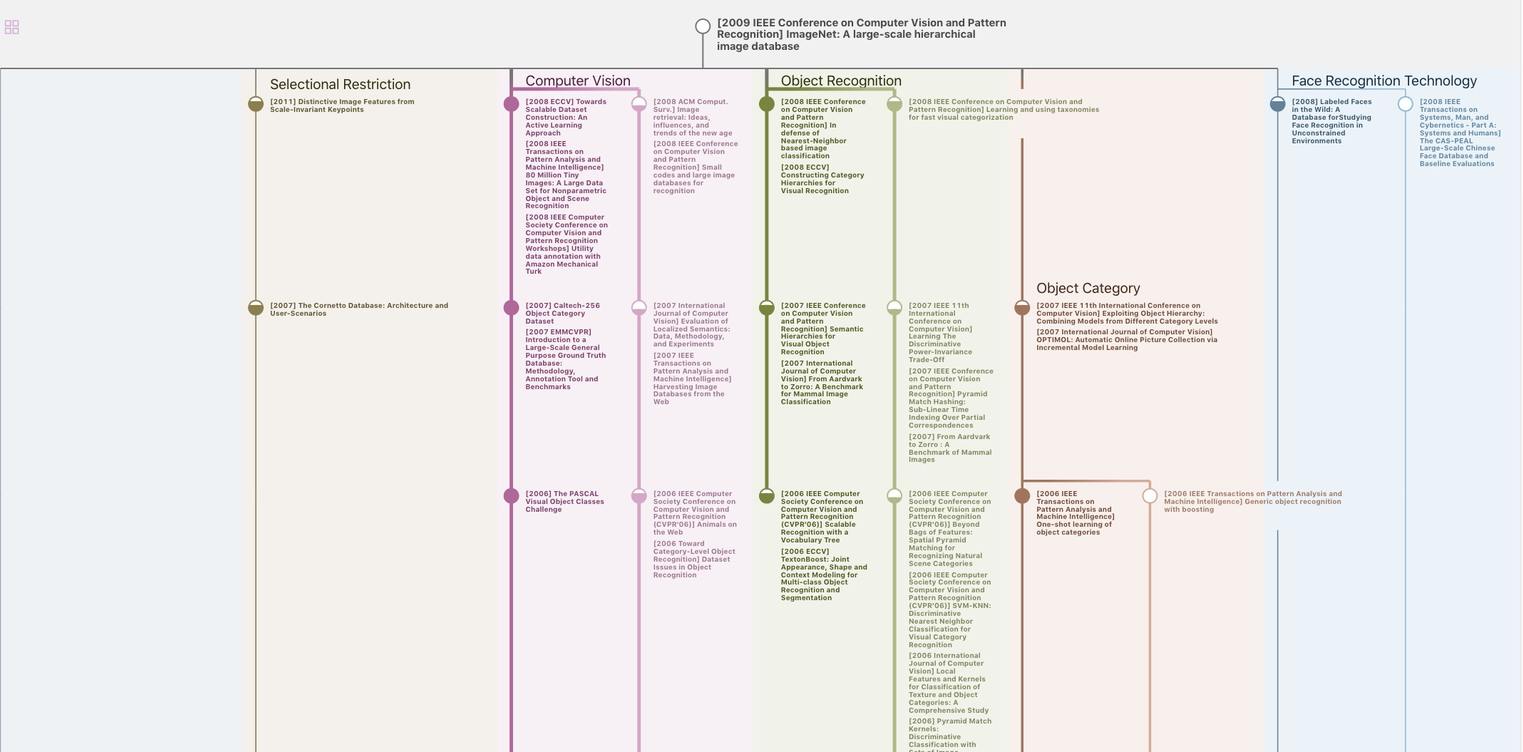
生成溯源树,研究论文发展脉络
Chat Paper
正在生成论文摘要