A novel tool condition monitoring based on Gramian angular field and comparative learning
INTERNATIONAL JOURNAL OF HYDROMECHATRONICS(2023)
摘要
Accurate tool condition monitoring (TCM) is an important part for ensuring milling quality. However, due to the cost of TCM experiment, there are few labelled and a lot of unlabelled samples in the training set that significantly affect the accuracy of many machine learning models. A novel method based on comparative learning (CL) and Gramian angular field (GAF) is proposed for improving the performance of TCM. The cutting force signals of each channel of all samples (including labelled and unlabelled) collected in TCM experiment are expanded to grey images by GAF, and combined with other channels to a colour image. Then, these colour images are input to the CL pre-training model to learn features. Finally, the extracted features and the few labelled samples are applied to train the ResNet18 model to obtain excellent classification results. The milling TCM experiments show that the classification precision of the proposed GAF-CL model is above 95% with small labelled samples, which is more than 19% higher than the ImageNet pre-training model.
更多查看译文
关键词
tool condition monitoring, TCM, comparative learning, CL, Gramian angular field, residual network
AI 理解论文
溯源树
样例
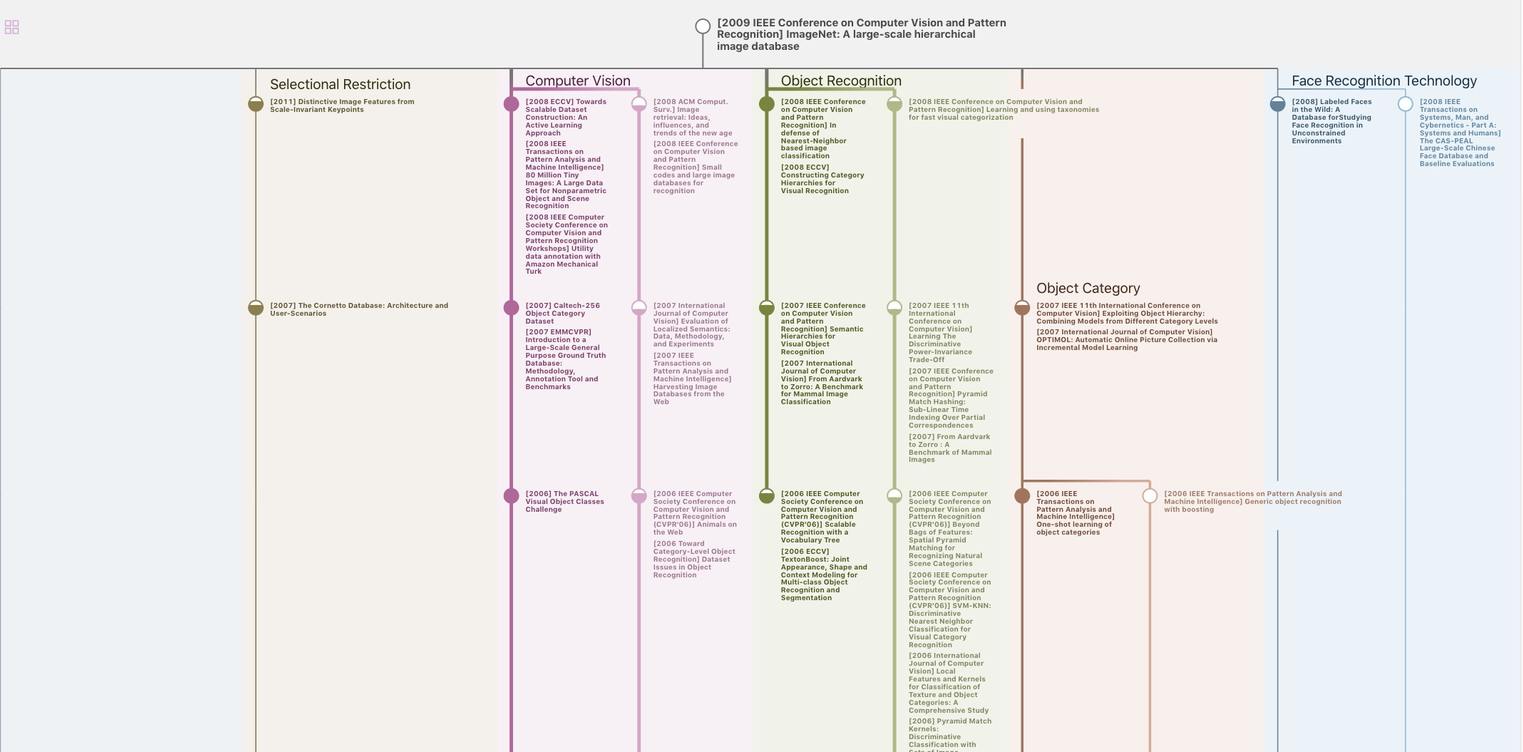
生成溯源树,研究论文发展脉络
Chat Paper
正在生成论文摘要