Multiagent Multimodal Trajectory Prediction in Urban Traffic Scenarios Using a Neural Network-Based Solution
MATHEMATICS(2023)
摘要
Trajectory prediction in urban scenarios is critical for high-level automated driving systems. However, this task is associated with many challenges. On the one hand, a scene typically includes different traffic participants, such as vehicles, buses, pedestrians, and cyclists, which may behave differently. On the other hand, an agent may have multiple plausible future trajectories based on complex interactions with the other agents. To address these challenges, we propose a multiagent, multimodal trajectory prediction method based on neural networks, which encodes past motion information, group context, and road context to estimate future trajectories by learning from the interactions of the agents. At inference time, multiple realistic future trajectories are predicted. Our solution is based on an encoder-decoder architecture that can handle a variable number of traffic participants. It uses vectors of agent features as inputs rather than images, and it is designed to run on a physical autonomous car, addressing the real-time operation requirements. We evaluate the method using the inD dataset for each type of traffic participant and provide information about its integration into an actual self-driving car.
更多查看译文
关键词
urban traffic scenarios,network-based
AI 理解论文
溯源树
样例
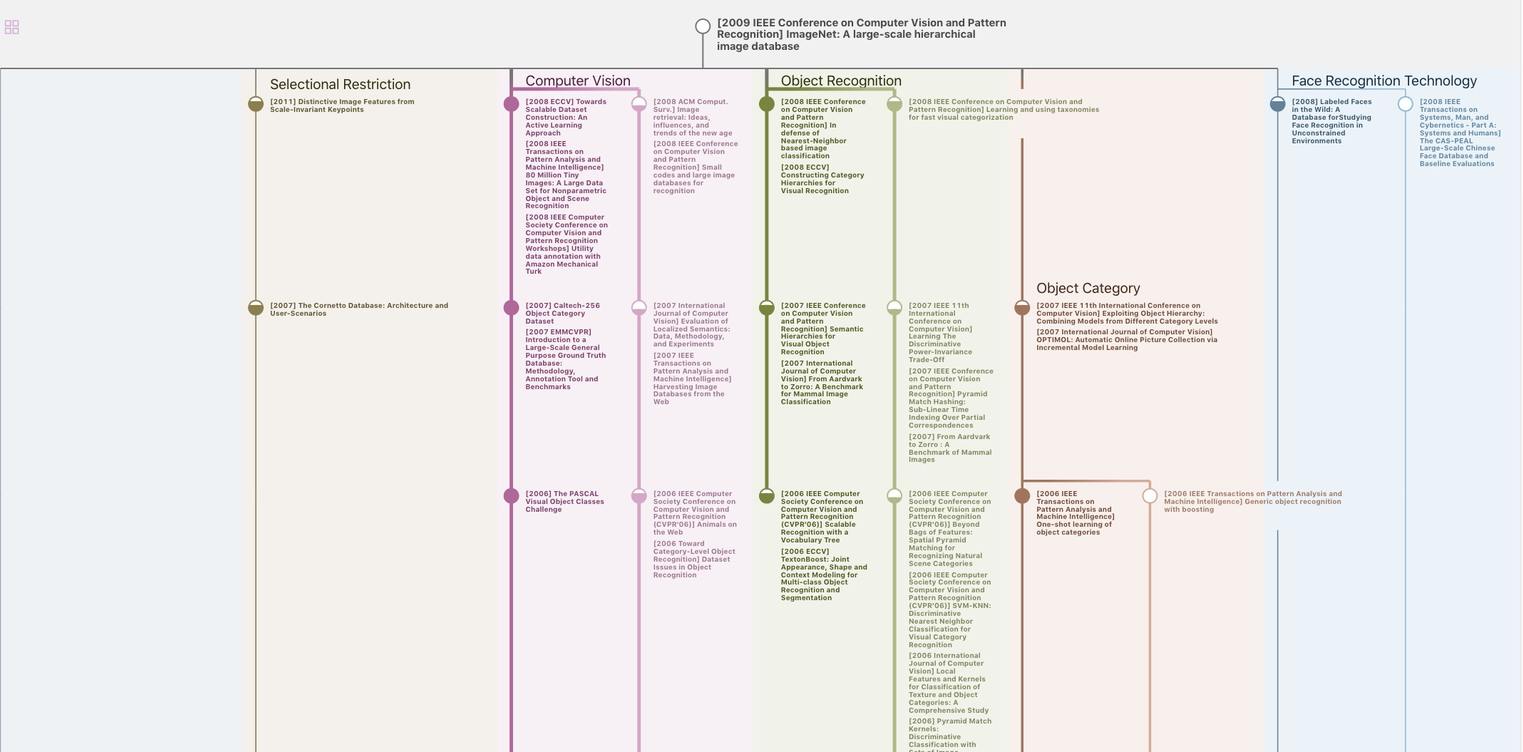
生成溯源树,研究论文发展脉络
Chat Paper
正在生成论文摘要