Deep learning in the marking of medical student short answer question examinations: Student perceptions and pilot accuracy assessment
FOCUS ON HEALTH PROFESSIONAL EDUCATION-A MULTIDISCIPLINARY JOURNAL(2023)
摘要
Introduction: Machine learning has previously been applied to text analysis. There is limited data regarding the acceptability or accuracy of such applications in medical education. This project examined medical student opinion regarding computer-based marking and evaluated the accuracy of deep learning (DL), a subtype of machine learning, in the scoring of medical short answer questions (SAQs). Methods: Fourth- and fifth-year medical students undertook an anonymised online examination. Prior to the examination, students completed a survey gauging their opinion on computer-based marking. Questions were marked by humans, and then a DL analysis was conducted using convolutional neural networks. In the DL analysis, following preprocessing, data were split into a training dataset (on which models were developed using 10-fold cross-validation) and a test dataset (on which performance analysis was conducted). Results: One hundred and eighty-one students completed the examination (participation rate 59.0%). While students expressed concern regarding the accuracy of computer-based marking, the majority of students agreed that computer marking would be more objective than human marking (67.0%) and reported they would not object to computer-based marking (55.5%). Regarding automated marking of SAQs, for 1-mark questions, there were consistently high classification accuracies (mean accuracy 0.98). For more complex 2-mark and 3-mark SAQs, in which multiclass classification was required, accuracy was lower (mean 0.65 and 0.59, respectively). Conclusions: Medical students may be supportive of computer-based marking due to its objectivity. DL has the potential to provide accurate marking of written questions, however further research into DL marking of medical examinations is required.
更多查看译文
关键词
deep learning, natural language processing, automation, medical education
AI 理解论文
溯源树
样例
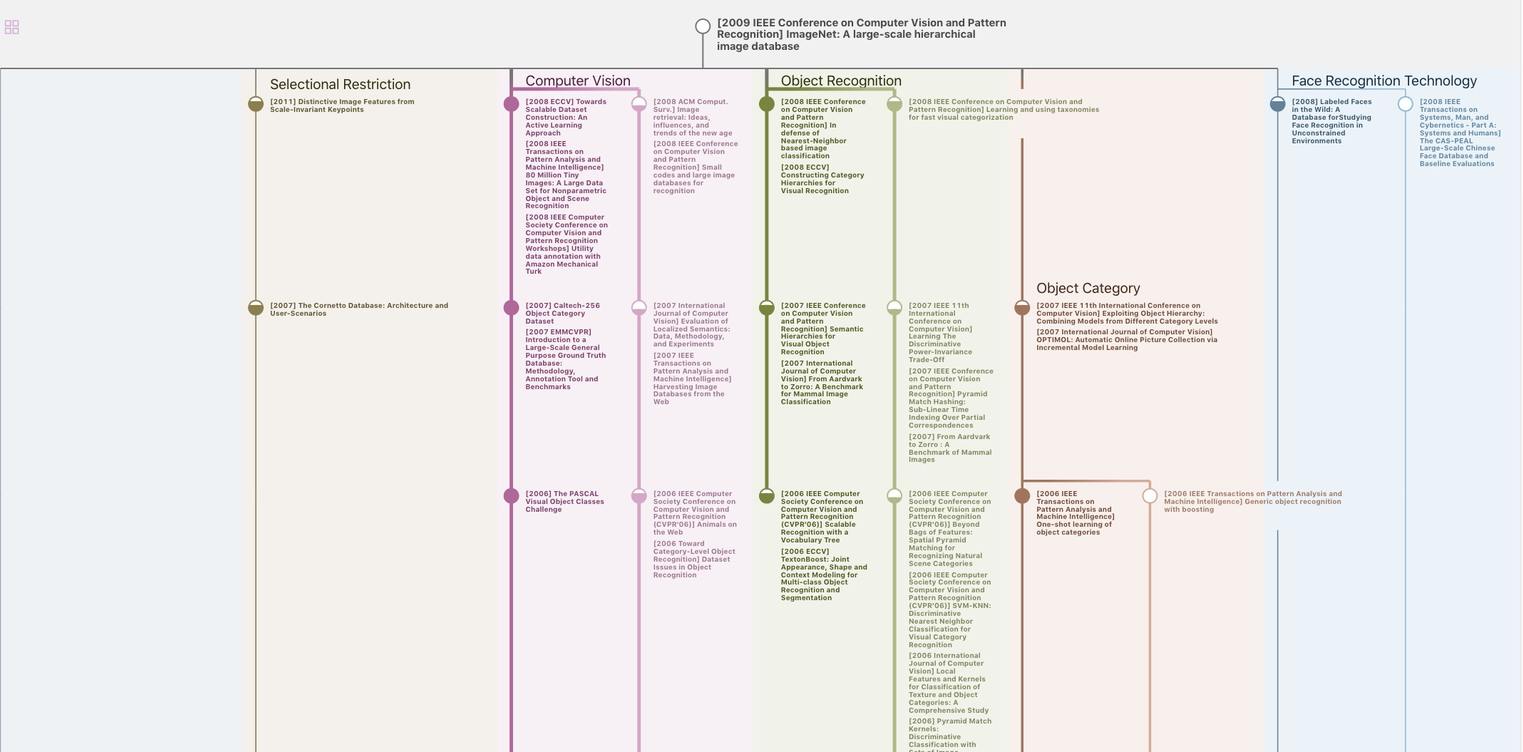
生成溯源树,研究论文发展脉络
Chat Paper
正在生成论文摘要