QSLRS-CNN: Qur'anic sign language recognition system based on convolutional neural networks
IMAGING SCIENCE JOURNAL(2024)
摘要
Deaf and dumb Muslims face educational barriers. They can't read, recite, or comprehend the Holy Qur'an, hence they can't practise Islamic ceremonies. This study proposes a CNN-based Qur'anic sign language recognition methodology. First, photos are used to train for dynamic and static gesture recognition. Second, preparing images diversifies datasets. Finally, CNN-based deep learning models extract and classify features. To teach the deaf and dumb Islamic ceremonies, the programme recognises Arabic sign language hand motions referring to dashed Qur'anic letters. Only 24,137 photos of the Holy Qur'an's 14 dashed letters were used in the trials from ArSL2018, a huge Arabic sign language collection. SMOTE raises training and testing accuracy to 98.31% and 97.67%, respectively, whereas the proposed model reaches 98.05% and 97.13%. RMU obtains 98.66% and 97.52% training and testing accuracy, whereas RMO achieves 98.37% and 97.36%.
更多查看译文
关键词
Holy Qur'an,Qur'anic sign language,RMO,convolutional neural network,SMOTE,sign language,deep Learning,feature extraction
AI 理解论文
溯源树
样例
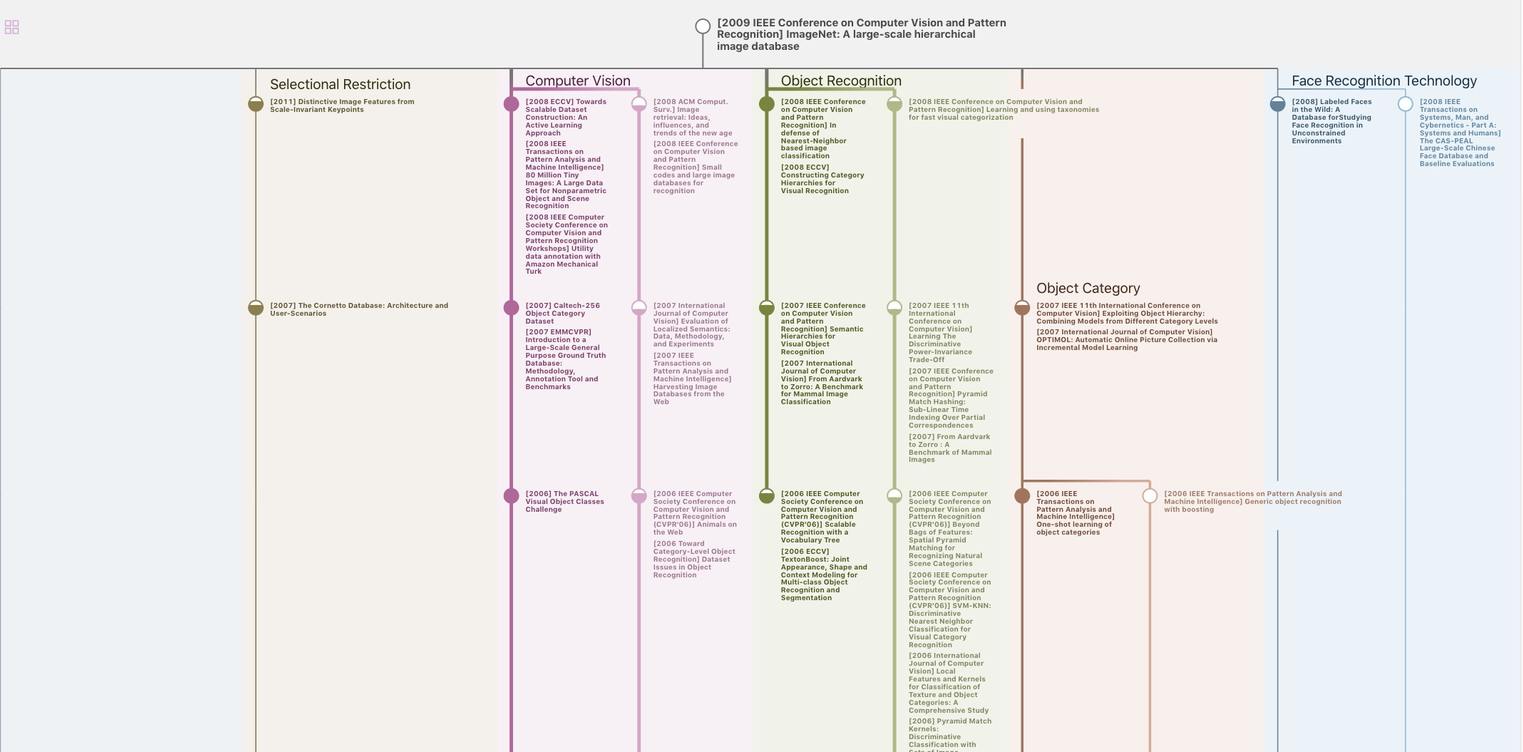
生成溯源树,研究论文发展脉络
Chat Paper
正在生成论文摘要