A CNN-LSTM-Based Model to Fault Diagnosis for CPR1000
NUCLEAR TECHNOLOGY(2023)
Abstract
With the advancement of artificial intelligence technology, intelligent diagnostic technology has been gradually implemented across various industries. This study proposes the use of convolutional neural networks-long short-term memory (CNNs-LSTM) for diagnosing faults in CPR1000 nuclear power plants (NPPs). To automatically extract data related to different types and levels of faults in the PCTRAN program, the study utilizes a self-developed AutoPCTRAN software and selects several key nuclear parameters as feature quantities. The study uses random sampling to create the training, validation, and test sets in an 8:1:1 ratio and identifies acceptable parameters to build the CNN-LSTM model. Test results show that the CNN-LSTM-based model for diagnosing CPR1000 NPP faults achieves a problem recognition rate of 99.6%, which validates the efficacy of the CNN-LSTM-based nuclear power fault diagnosis model.
MoreTranslated text
Key words
fault diagnosis,cnn-lstm
AI Read Science
Must-Reading Tree
Example
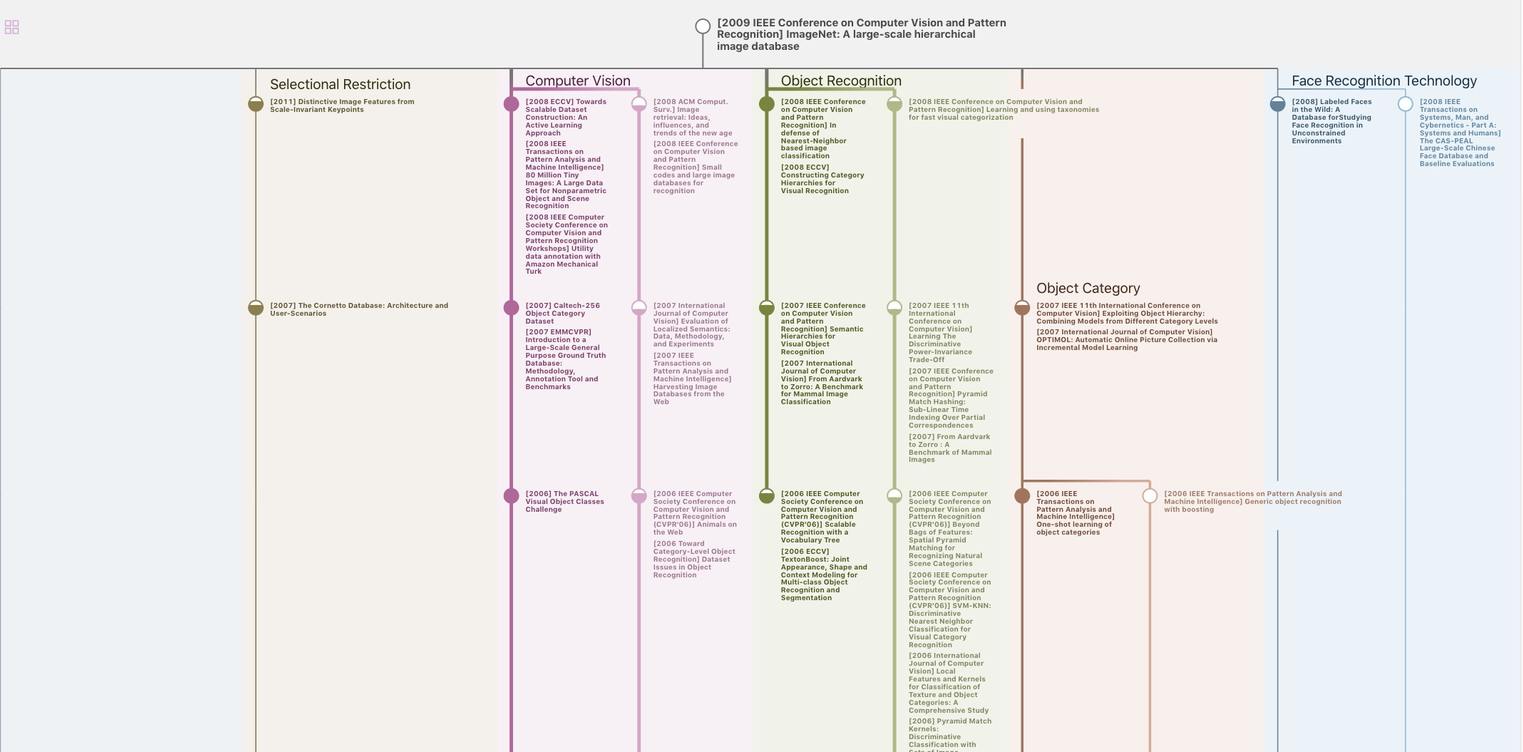
Generate MRT to find the research sequence of this paper
Chat Paper
Summary is being generated by the instructions you defined