Low-rank matrix estimation via nonconvex optimization methods in multi-response errors-in-variables regression
JOURNAL OF GLOBAL OPTIMIZATION(2024)
摘要
Noisy and missing data cannot be avoided in real application, such as bioinformatics, economics and remote sensing. Existing methods mainly focus on linear errors-in-variables regression, while relatively little attention is paid for the case of multivariate responses, and how to achieve consistent estimation under corrupted covariates is still an open question. In this paper, a nonconvex error-corrected estimator is proposed for the matrix estimation problem in the multi-response errors-in-variables regression model. Statistical and computational properties for global solutions of the estimator are analysed. In the statistical aspect, the nonasymptotic recovery bound for all global solutions of the nonconvex estimator is established. In the computational aspect, the proximal gradient method is applied to solve the nonconvex optimization problem and proved to linearly converge to a near-global solution. Sufficient conditions are verified in order to obtain probabilistic consequences for specific types of measurement errors by virtue of random matrix analysis. Finally, simulation results on synthetic and real neuroimaging data demonstrate the theoretical properties and show nice consistency under high-dimensional scaling.
更多查看译文
关键词
Nonconvex optimization,Low-rank regularization,Recovery bound,Proximal gradient methods,Linear convergence
AI 理解论文
溯源树
样例
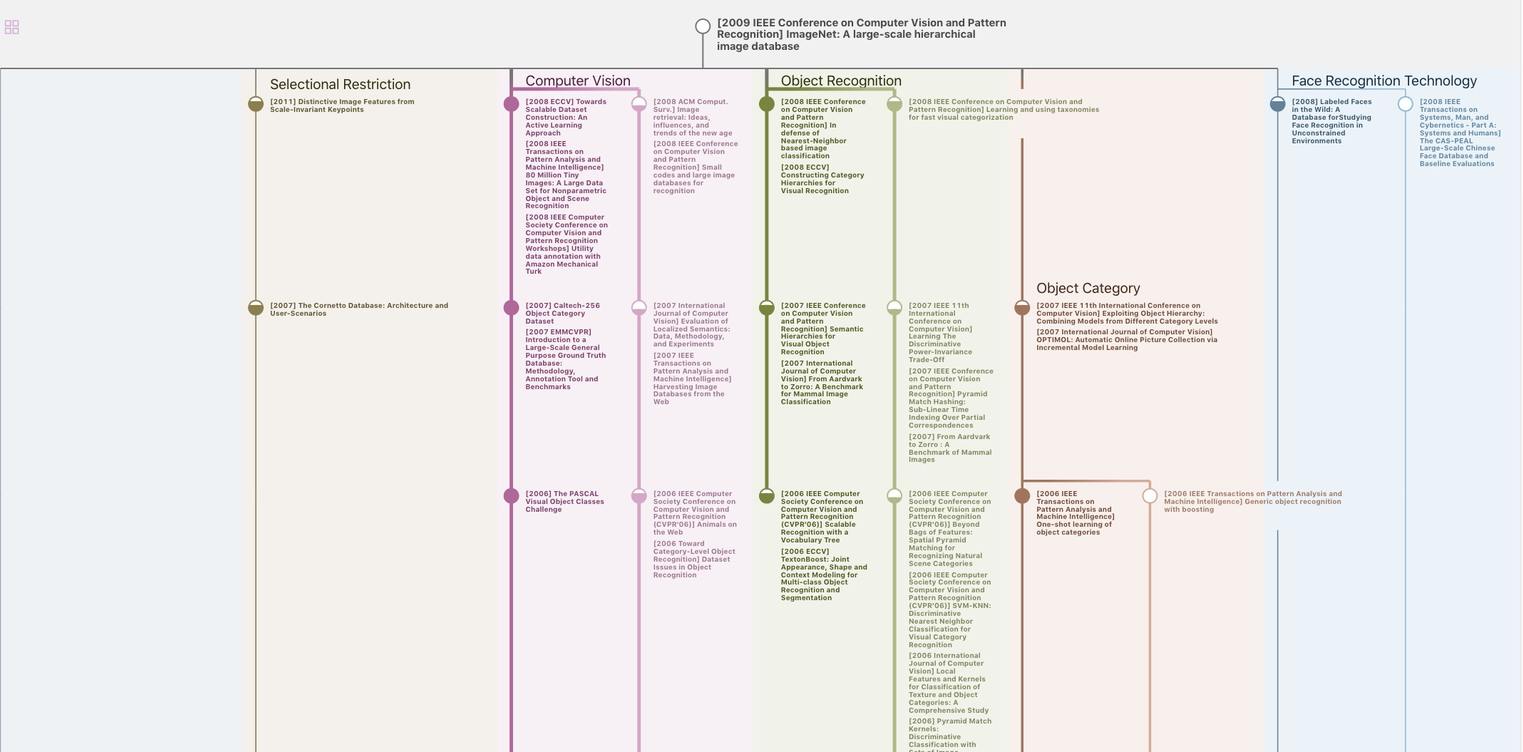
生成溯源树,研究论文发展脉络
Chat Paper
正在生成论文摘要