A probabilistic successor representation for context-dependent learning.
Psychological review(2023)
摘要
Two of the main impediments to learning complex tasks are that relationships between different stimuli, including rewards, can be uncertain and context-dependent. Reinforcement learning (RL) provides a framework for learning, by predicting total future reward directly (model-free RL), or via predictions of future states (model-based RL). Within this framework, "successor representation" (SR) predicts total future occupancy of all states. A recent theoretical proposal suggests that the hippocampus encodes the SR in order to facilitate prediction of future reward. However, this proposal does not take into account how learning should adapt under uncertainty and switches of context. Here, we introduce a theory of learning SRs using prediction errors which includes optimally balancing uncertainty in new observations versus existing knowledge. We then generalize that approach to a multicontext setting, allowing the model to learn and maintain multiple task-specific SRs and infer which one to use at any moment based on the accuracy of its predictions. Thus, the context used for predictions can be determined by both the contents of the states themselves and the distribution of transitions between them. This probabilistic SR model captures animal behavior in tasks which require contextual memory and generalization, and unifies previous SR theory with hippocampal-dependent contextual decision-making. (PsycInfo Database Record (c) 2023 APA, all rights reserved).
更多查看译文
关键词
reinforcement learning,successor representation,uncertainty,context
AI 理解论文
溯源树
样例
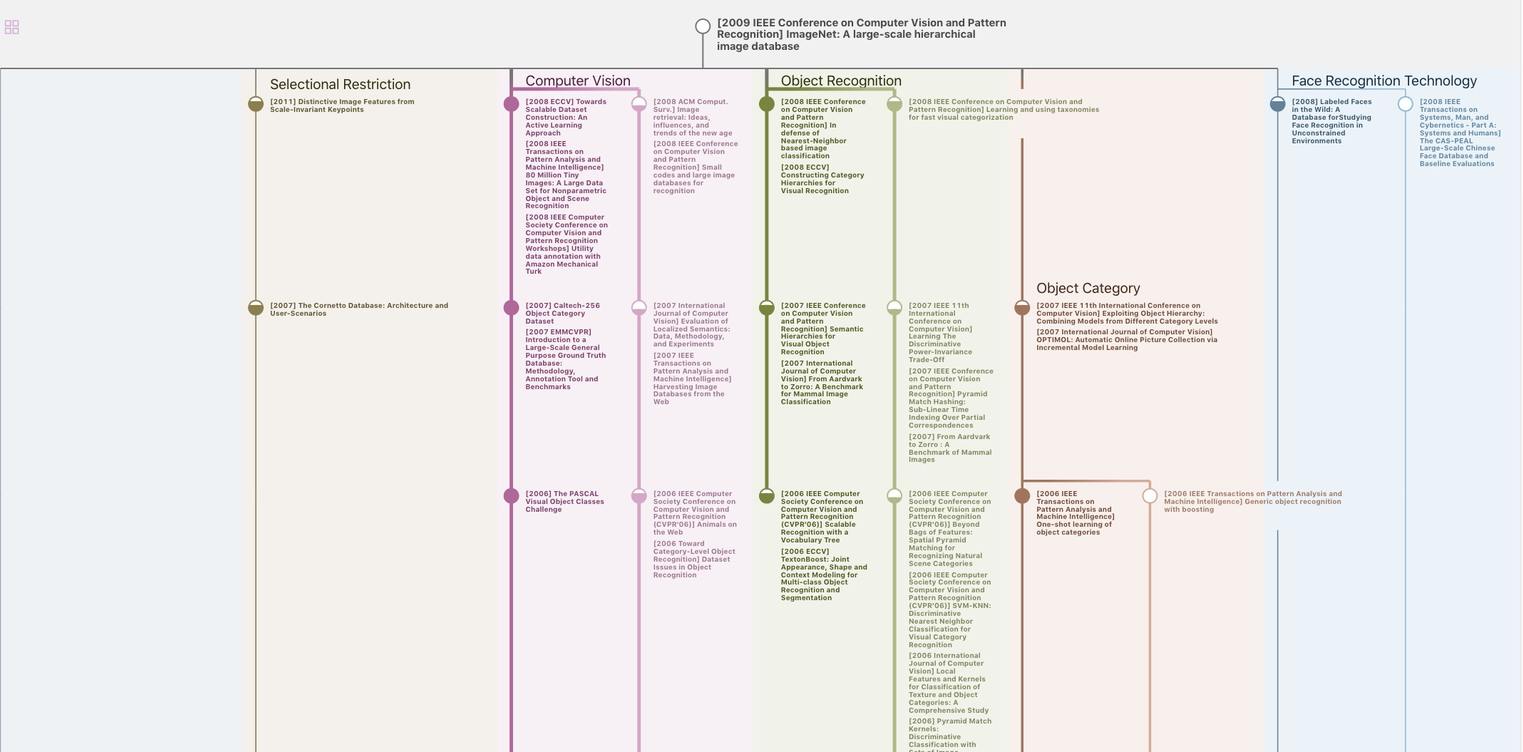
生成溯源树,研究论文发展脉络
Chat Paper
正在生成论文摘要