Bandgap energy prediction of senary zincblende III-V semiconductor compounds using machine learning
MATERIALS SCIENCE IN SEMICONDUCTOR PROCESSING(2023)
摘要
Two complimentary machine learning (ML) models are developed to estimate the bandgap energy (Eg) of senary zincblende (ZB) III-V semiconductor compounds Alx1 Gax2Inx3Py1 Asy2Sby3 from molar fractions. The models work - as well - for any compound with smaller number of constituent elements from binaries to quinaries. Some of these compounds exhibit transition between a direct and an indirect Eg as a function of composition. This is due to the fact that the bandgap for this class of materials is either the direct gap at f point (Egf) or the indirect one between the maximum valance band at f point and the minimum conduction band around X point (EgX ). Hence, to capture precisely the Eg and its state, the ML models estimate separately Egf EgX III- the and where the lowest energy gap is the effective Eg. For model training, 18 ternary ZB V semiconductor compounds are used, which contain all possible ternary configurations of III-type cations (Al, Ga, In) and V-type anions (P, As, Sb). The achieved testing accuracy using cubic regression with ridge regularization - in terms of root mean square error (RMSE) - are 7.583 and 0.021 meV for Egf and EgX, respectively. These correspond to R2 of 0.99995 and 1.00000 (8 nines), respectively. To validate the models, they are benchmarked against experimental measurements of four different quaternary ZB III-V semiconductor compounds. The agreement is very noticeable demonstrating the validity and the excellent accuracy of the developed models.
更多查看译文
关键词
Machine learning (ML),Bandgap(Eg),Zincblende (ZB),III-V semiconductor compound,Regression analysis
AI 理解论文
溯源树
样例
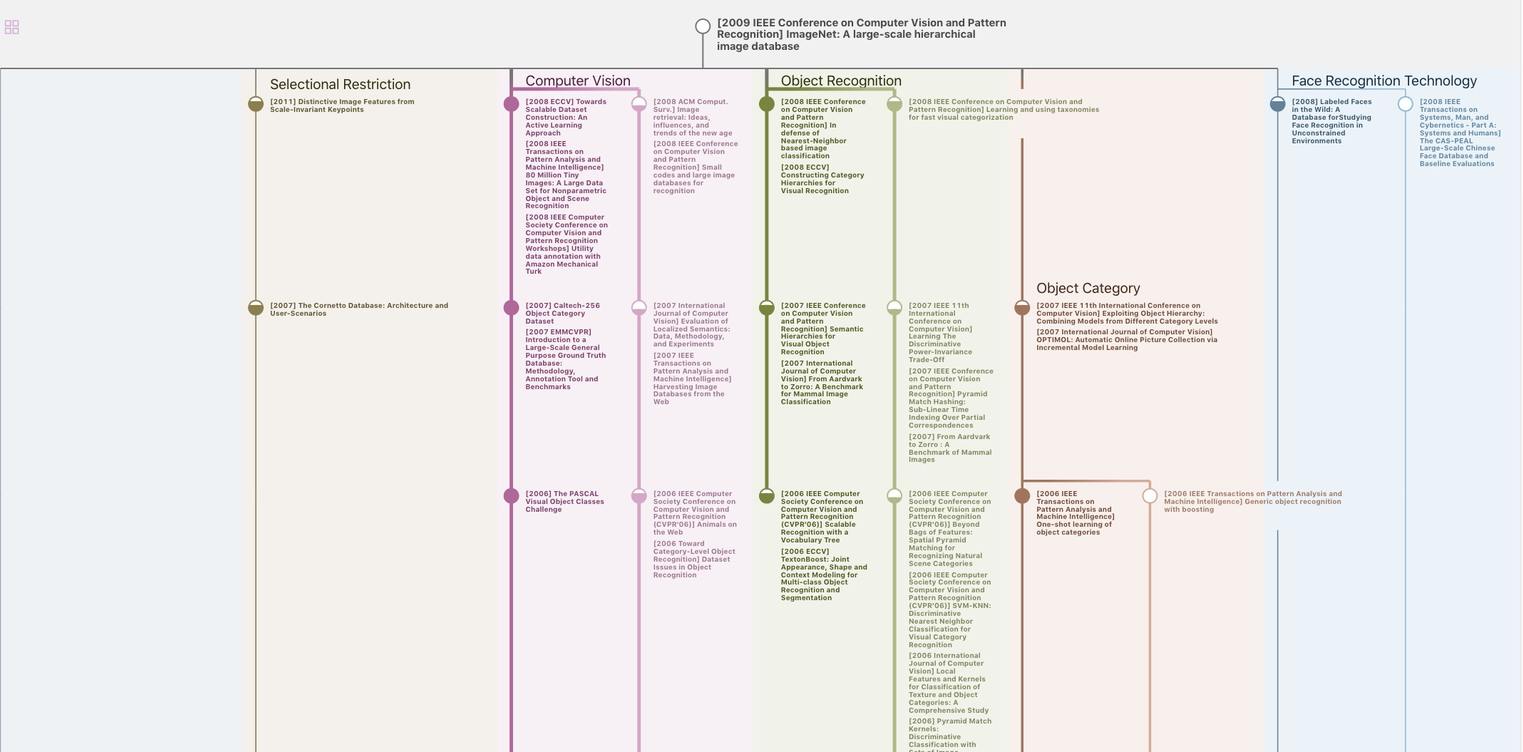
生成溯源树,研究论文发展脉络
Chat Paper
正在生成论文摘要