Multifuzzy b-Covering Approximation Spaces and Their Information Measures
IEEE TRANSACTIONS ON FUZZY SYSTEMS(2023)
摘要
Fuzzy b-covering rough sets, as an effective extension of covering-based rough sets, have been concerned by many re-searchers. All fuzzy ,3-covering rough set models are constructed under a corresponding fuzzy b-covering approximation space. However, fuzzy b-covering is difficult to find directly from the real data. Fortunately, fuzzy information granulation provides a reasonable and effective way to obtain fuzzy b-coverings from the real data. Since fuzzy information granulation is capable of generating multiple fuzzy b-coverings, we introduce the notion of multifuzzy b-covering approximation spaces. Fuzzy b-covering approximation spaces are a special case of multifuzzy b-covering approximation spaces. Besides, we employ fuzzy b-neighborhood operators with reflexivity and symmetry to characterize the simi-larity between samples. In this article, we first present the definition of multifuzzy b-covering approximation spaces and investigate some useful properties about fuzzy b-covering. Second, several information measures are explored in the context of multifuzzy b-covering approximation spaces. On this basis, a novel heuristic fuzzy b-covering reduction method with the measure of monotone conditional entropy is proposed. Moreover, a general framework of attribute reduction based on fuzzy b-covering reduction is also de-signed. Finally, through the comparative and experimental analyses with other four state-of-the-art attribute reduction methods, the effectiveness and superiority of the proposed method are verified.
更多查看译文
关键词
Attribute reduction, fuzzy, b-covering, fuzzy rough sets, granular computing, uncertainty measure
AI 理解论文
溯源树
样例
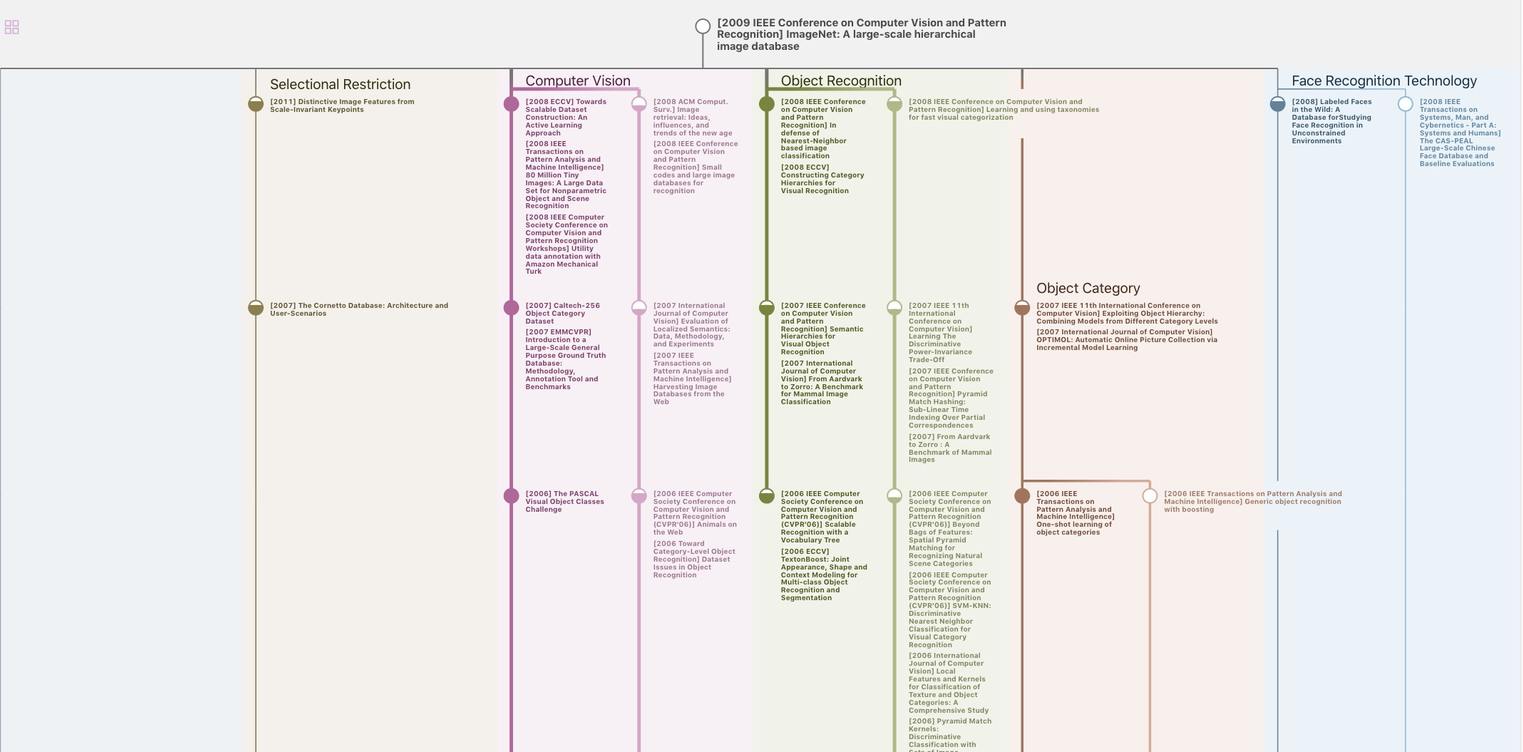
生成溯源树,研究论文发展脉络
Chat Paper
正在生成论文摘要