scRAA: the development of a robust and automatic annotation procedure for single-cell RNA sequencing data
JOURNAL OF BIOPHARMACEUTICAL STATISTICS(2023)
Abstract
A critical task in single-cell RNA sequencing (scRNA-Seq) data analysis is to identify cell types from heterogeneous tissues. While the majority of classification methods demonstrated high performance in scRNA-Seq annotation problems, a robust and accurate solution is desired to generate reliable outcomes for downstream analyses, for instance, marker genes identification, differentially expressed genes, and pathway analysis. It is hard to establish a universally good metric. Thus, a universally good classification method for all kinds of scenarios does not exist. In addition, reference and query data in cell classification are usually from different experimental batches, and failure to consider batch effects may result in misleading conclusions. To overcome this bottleneck, we propose a robust ensemble approach to classify cells and utilize a batch correction method between reference and query data. We simulated four scenarios that comprise simple to complex batch effect and account for varying cell-type proportions. We further tested our approach on both lung and pancreas data. We found improved prediction accuracy and robust performance across simulation scenarios and real data. The incorporation of batch effect correction between reference and query, and the ensemble approach improve cell-type prediction accuracy while maintaining robustness. We demonstrated these through simulated and real scRNA-Seq data.
MoreTranslated text
Key words
Batch effect correction, cell-type classification, ensembled method
AI Read Science
Must-Reading Tree
Example
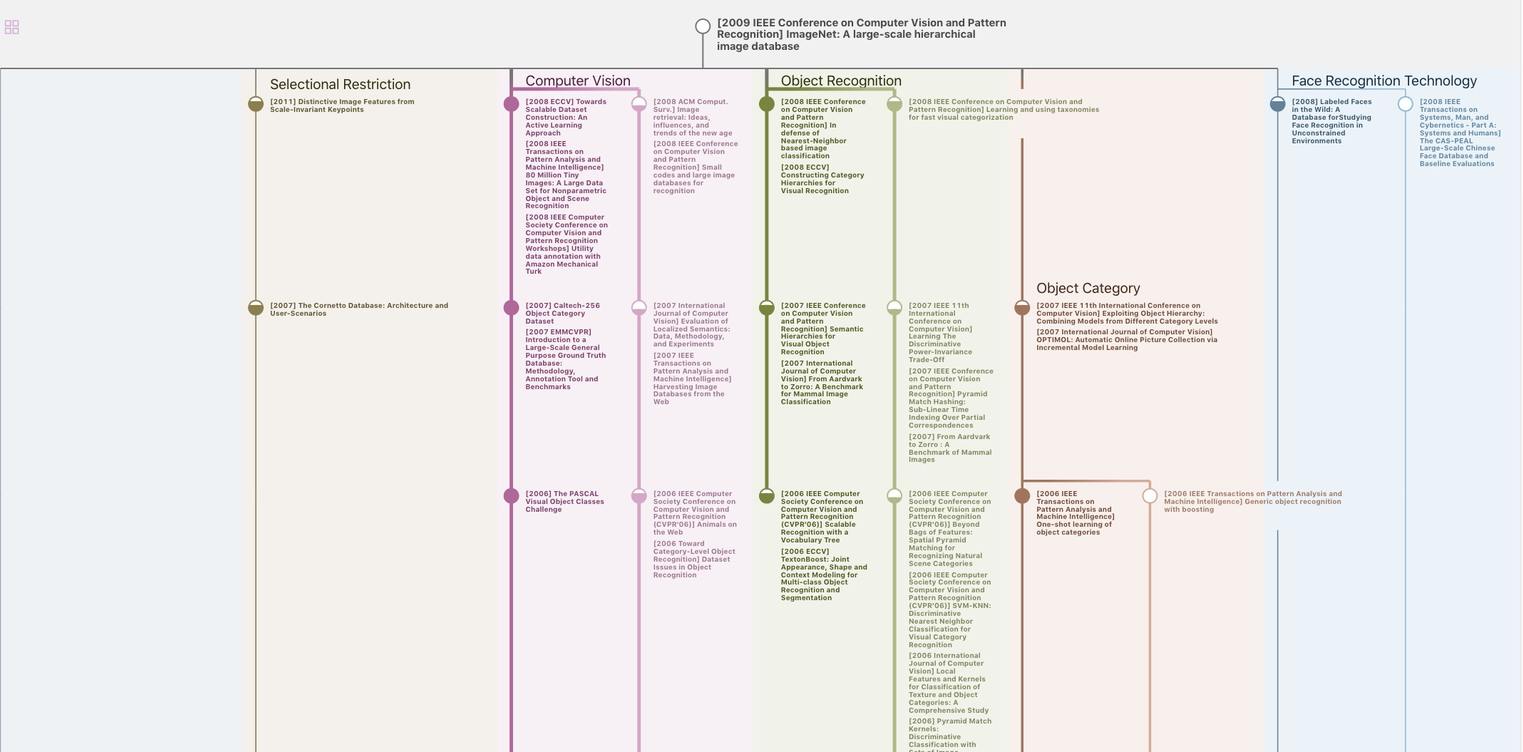
Generate MRT to find the research sequence of this paper
Chat Paper
Summary is being generated by the instructions you defined