Class-Imbalanced-Aware Distantly Supervised Named Entity Recognition
IEEE TRANSACTIONS ON NEURAL NETWORKS AND LEARNING SYSTEMS(2024)
关键词
Estimation,Optimization,Training,Dictionaries,Learning systems,Labeling,Electronic mail,Class imbalance,class prior estimation,named entity recognition (NER),positive unlabeled (PU) learning
AI 理解论文
溯源树
样例
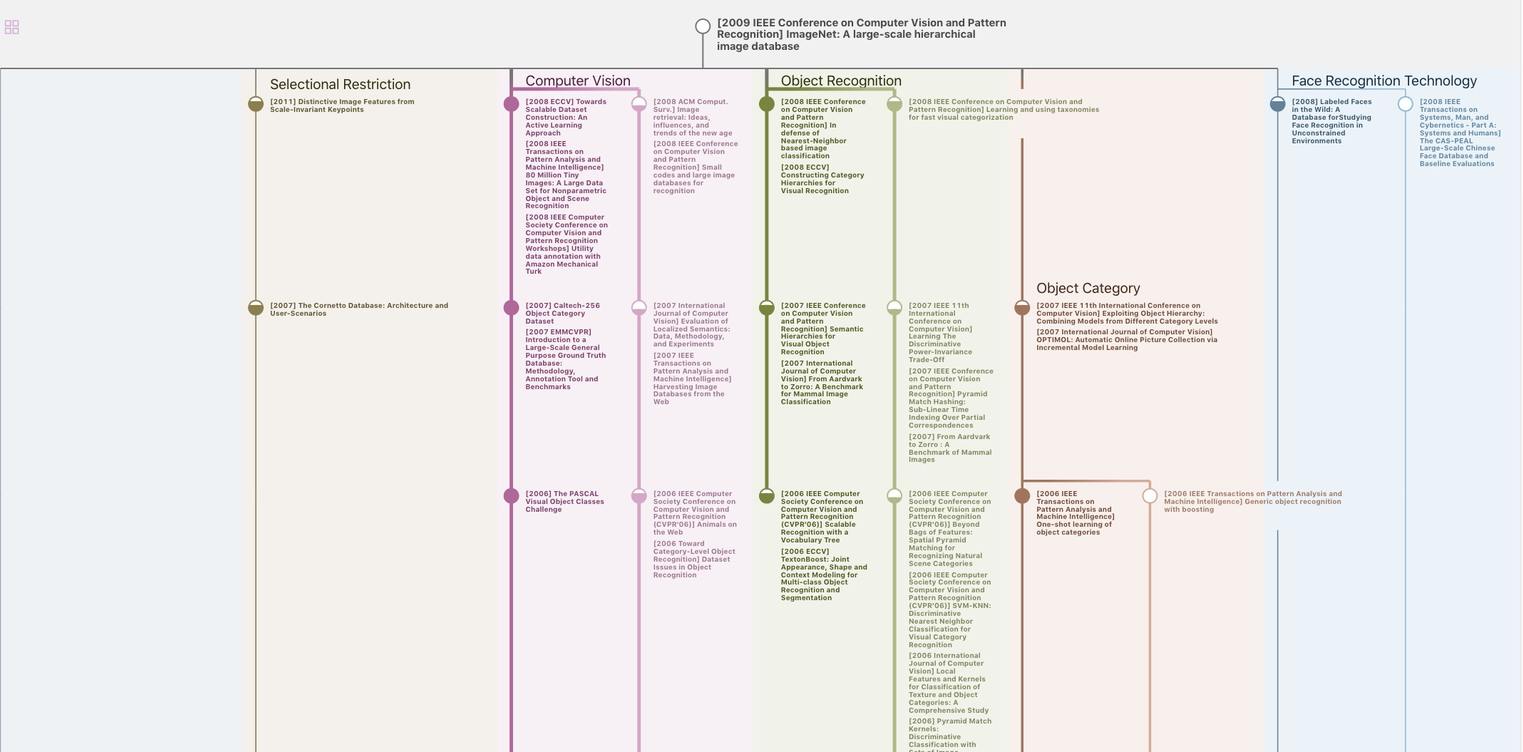
生成溯源树,研究论文发展脉络
Chat Paper
正在生成论文摘要