Characterizations on Knee Movement Estimation from Surface EMG Using Composited Approaches
JOURNAL OF ELECTRICAL ENGINEERING & TECHNOLOGY(2024)
摘要
This paper focuses on the relationship between surface electromyography (sEMG) signals and knee joint movement which would be applied in the extra assistant robotic legs (EARL). The characterizations of the sEMG data group for movement estimation were analyzed depending on the composited approaches, which were analyzed in situations of different sample sizes, different muscle groups, different time-domain features, and different algorithms. According to the analysis of knee movement estimation, it can be found that (1) Increasing the sample size, and the estimation accuracy becomes better. (2) The vastus lateralis and gastrocnemius lateralis have larger activation than the vastus medialis and gastrocnemius medialis, but the estimation accuracy is worse. (3) The estimation accuracy of time-domain features is related to the complexity of the feature group, the range of average AR 2 is 0.86 to 0.92. (4) The composited algorithms are better than a single back propagation neural network (BPNN), and principal component analysis is fitter than independent component analysis in conjunction with BPNN for estimation. This study reveals the hind factors that people cannot square up while extracting and processing the sEMG signals. This study’s results can help build new training data to improve the estimation accuracy of knee movement for sEMG.
更多查看译文
关键词
Surface electromyography, Knee movement estimation, Sample sizes, Muscle group, Time-domain feature
AI 理解论文
溯源树
样例
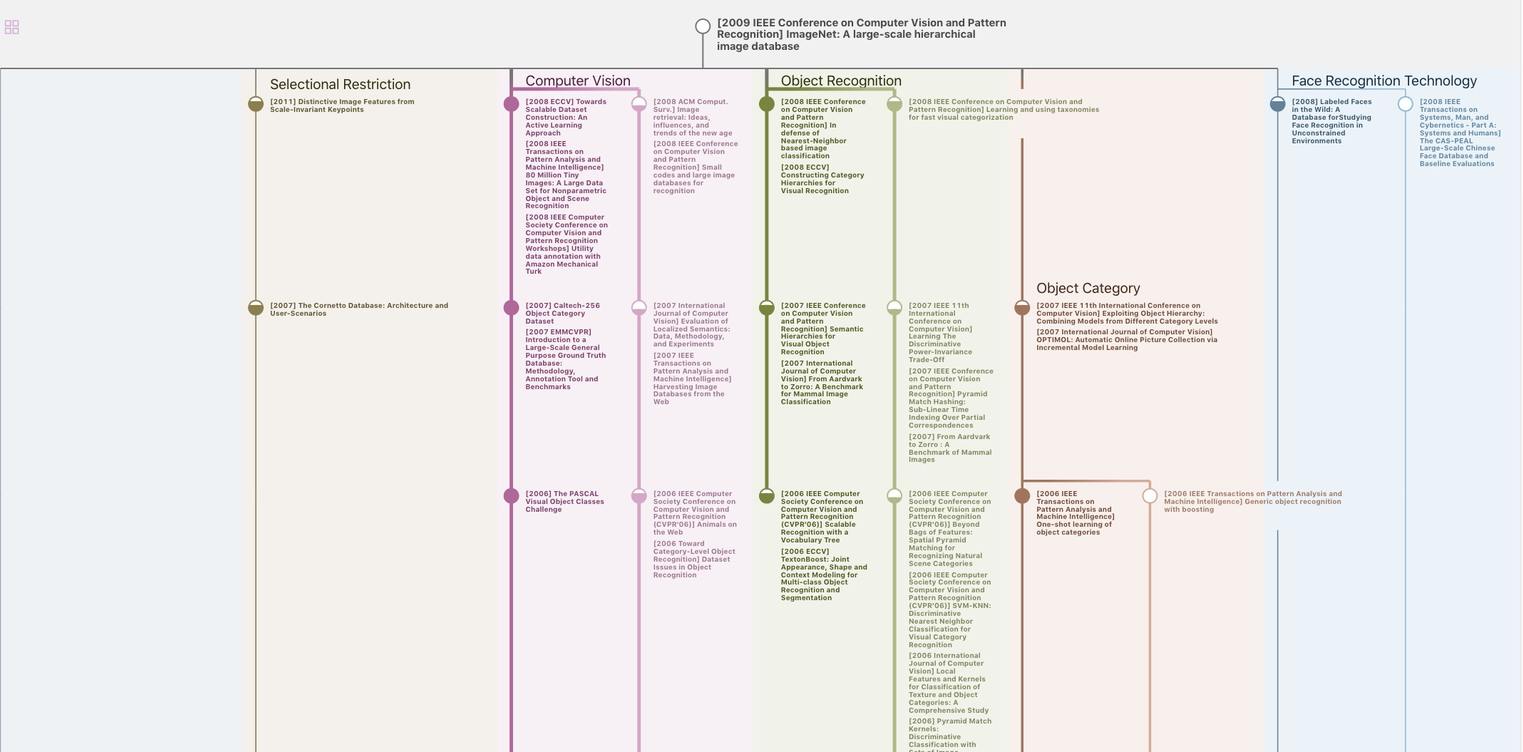
生成溯源树,研究论文发展脉络
Chat Paper
正在生成论文摘要