Predicting the COVID-19 Mortality among Iranian Patients Using Tree-Based Models: A Cross-Sectional Study
HEALTH SCIENCE REPORTS(2023)
关键词
COVID-19,gradient boosting,machine learning,prediction
AI 理解论文
溯源树
样例
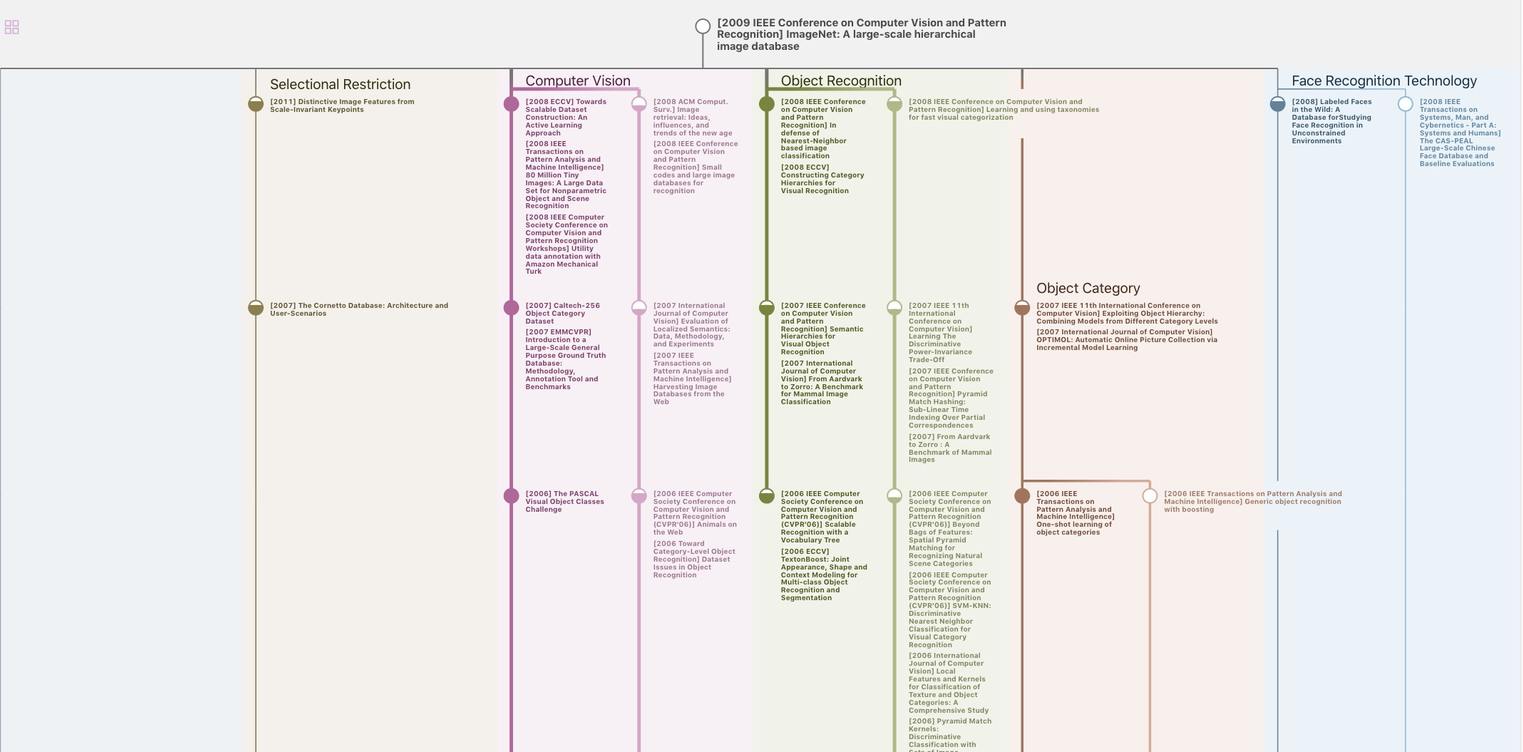
生成溯源树,研究论文发展脉络
Chat Paper
正在生成论文摘要
HEALTH SCIENCE REPORTS(2023)