Stream Temperature Prediction in a Shifting Environment: Explaining the Influence of Deep Learning Architecture
WATER RESOURCES RESEARCH(2023)
摘要
Stream temperature is a fundamental control on ecosystem health. Recent efforts incorporating process guidance into deep learning models for predicting stream temperature have been shown to outperform existing statistical and physical models. This performance is in part because deep learning architectures can actively learn spatiotemporal relationships that govern how water and energy propagate through a river network. However, exploration of how spatiotemporal awareness and process guidance influence a model's generalizability under shifting environmental conditions such as climate change is limited. Here, we use Explainable Artificial Intelligence (XAI) to interrogate how differing deep learning architectures affect a model's learned spatial and temporal dependencies, and how those learned dependencies affect a model's ability to maintain high accuracy when applied to unseen environmental conditions. Using the Delaware River Basin in the northeastern United States as a test case, we compare two spatiotemporally aware process-guided deep learning models for predicting stream temperature (a recurrent graph convolution network-RGCN, and a temporal convolution graph model-Graph WaveNet). Both models achieve equally high predictive performance when testing data are well represented in the training data (test root mean squared errors of 1.64 degrees C and 1.65 degrees C); however, Graph WaveNet significantly outperforms RGCN in 4 out of 5 experiments where test partitions represent different types of unseen environmental conditions. XAI results show that the architecture of Graph WaveNet leads to learned spatial relationships with greater fidelity to physical processes, and that this fidelity improves the generalizability of the model when applied to shifting and/or unseen environmental conditions. Plain Language Summary In a river network, the temperature of the water at a given point depends on a suite of information that spans space and time. Downstream reaches are influenced by upstream reaches, and an event such as rain today will influence the water temperature tomorrow. Certain types of deep learning models are capable of learning how this type of information moves through a river network to influence stream temperature. Existing work shows that using models that incorporate this information improves overall model performance, but little is known about how the differences between deep learning models lead to differences in learned spatial and temporal relationships. Here, we apply a variety of techniques to inspect how two different models learn spatial and temporal relationships. Our results show that one model learns relationships that intuitively match how we would expect information to move through a river network. This more accurate representation of the river system leads to improved model results when using the model to predict stream temperature in new environmental conditions that were not seen during training.
更多查看译文
关键词
process guided deep learning, generalization, explainable AI (XAI), stream temperature, domain shift
AI 理解论文
溯源树
样例
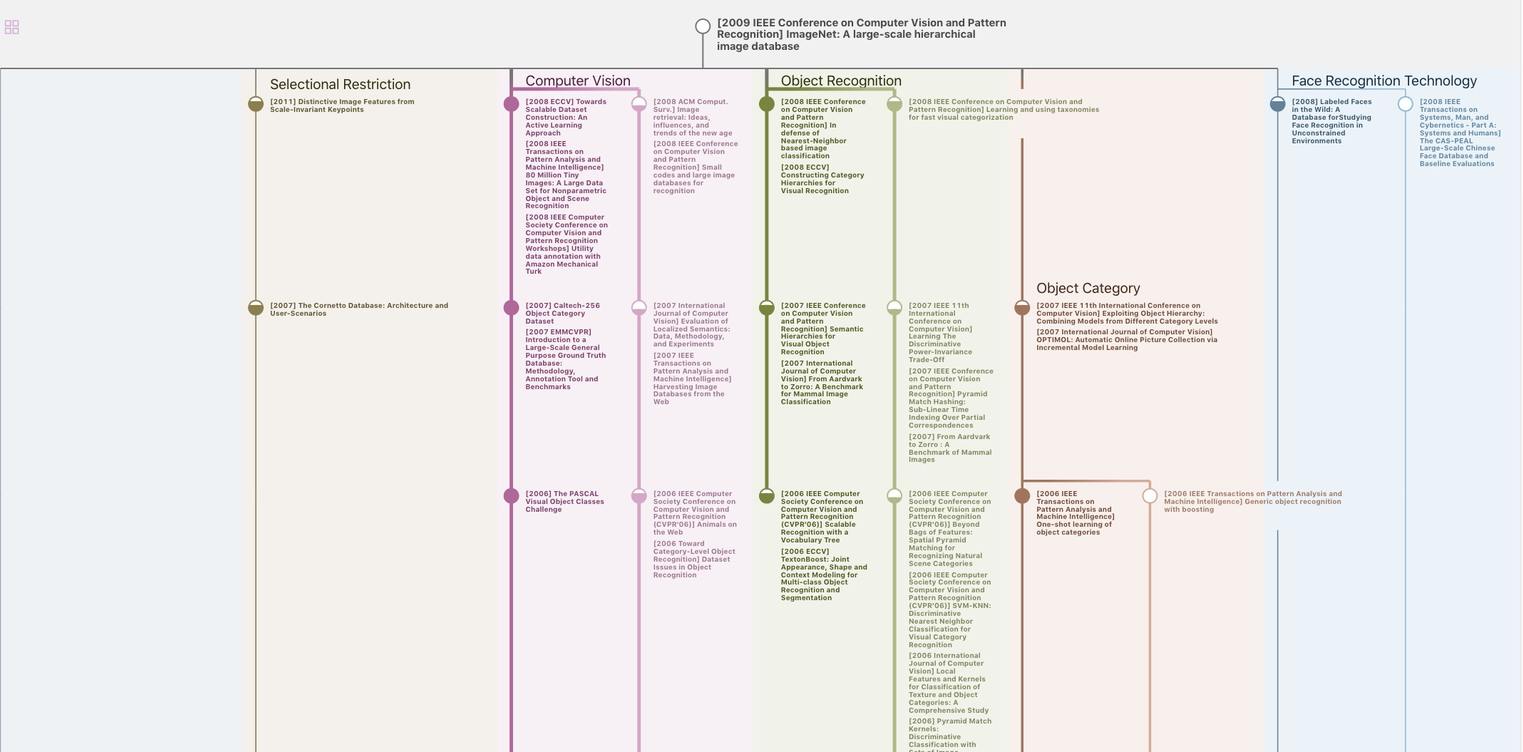
生成溯源树,研究论文发展脉络
Chat Paper
正在生成论文摘要