Application of Deep Learning in Drainage Systems Monitoring Data Repair-A Case Study Using Con-GRU Model
WATER(2023)
摘要
Hydraulic monitoring data is critical for optimizing drainage system design and predicting system performance, particularly in the establishment of data-driven hydraulic models. However, anomalies in monitoring data, caused by sensor failures and network fluctuations, can severely impact their practical application. Such anomalies can persist for long periods, and existing data repair methods are primarily designed for short-term time series data, with limited effectiveness in repairing long-term monitoring data. This research introduces the DSMDR, a deep learning framework designed for repairing monitored data in drainage systems. Within this framework, a deep learning model named Con-GRU is proposed for repairing water level monitoring data with long-term anomalies (i.e., 288 consecutive time points) in the pump station forebay. The model iteratively predicts 36 time points at each iteration and uses an iterative approach to achieve the repair process for long-term abnormal monitoring data. The Con-GRU model integrates analysis of forebay water levels, pump status, and rainfall features related to repair, and captures both long-term and local time-dependent features via one-dimensional convolution (Conv1D) and gated recurrent units (GRU). The proposed model improves the accuracy and authenticity of repaired water level data. The results indicate that, compared to existing long short-term memory neural network (LSTM) and artificial neural network (ANN) models, the Con-GRU model has significantly better performance in repairing water level data.
更多查看译文
关键词
deep learning,drainage,con-gru
AI 理解论文
溯源树
样例
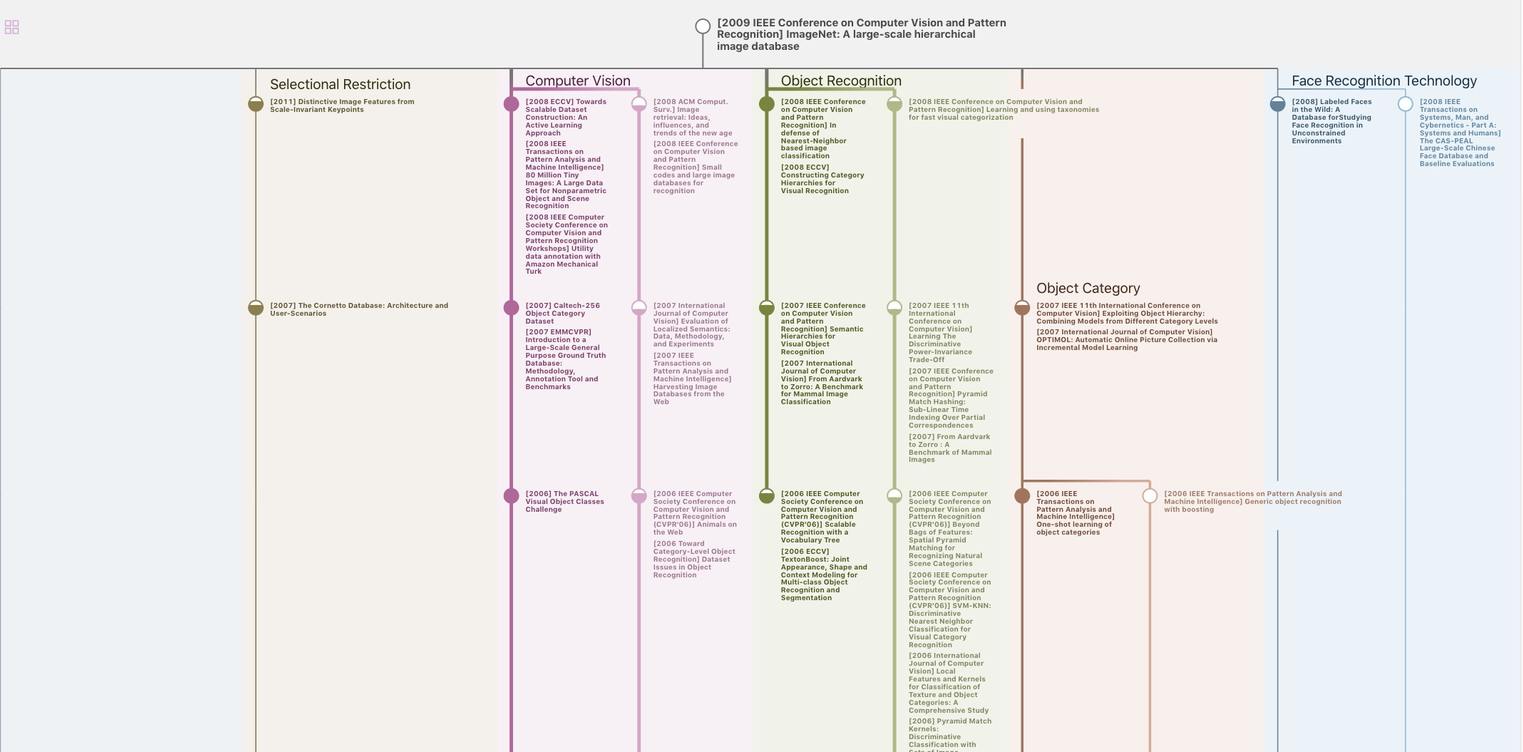
生成溯源树,研究论文发展脉络
Chat Paper
正在生成论文摘要