Unsupervised Multi-Scale-Stage Content-Aware Homography Estimation
ELECTRONICS(2023)
摘要
Homography estimation is a critical component in many computer-vision tasks. However, most deep homography methods focus on extracting local features and ignore global features or the corresponding relationship between features from two images or video frames. These methods are effective for alignment of image pairs with small displacement. In this paper, we propose an unsupervised Multi-Scale-Stage Content-Aware Homography Estimation Network (MS2CA-HENet). In the framework, we use multi-scale input images for different stages to cope with different scales of transformations. In each stage, we consider local and global features via our Self-Attention-augmented ConvNet (SAC). Furthermore, feature matching is explicitly enhanced using feature-matching modules. By shrinking the error residual of each stage, our network achieves coarse-to-fine results. Experiments show that our MS2CA-HENet achieves better results than other methods.
更多查看译文
关键词
unsupervised, multi-scale, multi-stage, self-attention-augmented ConvNet, feature matching
AI 理解论文
溯源树
样例
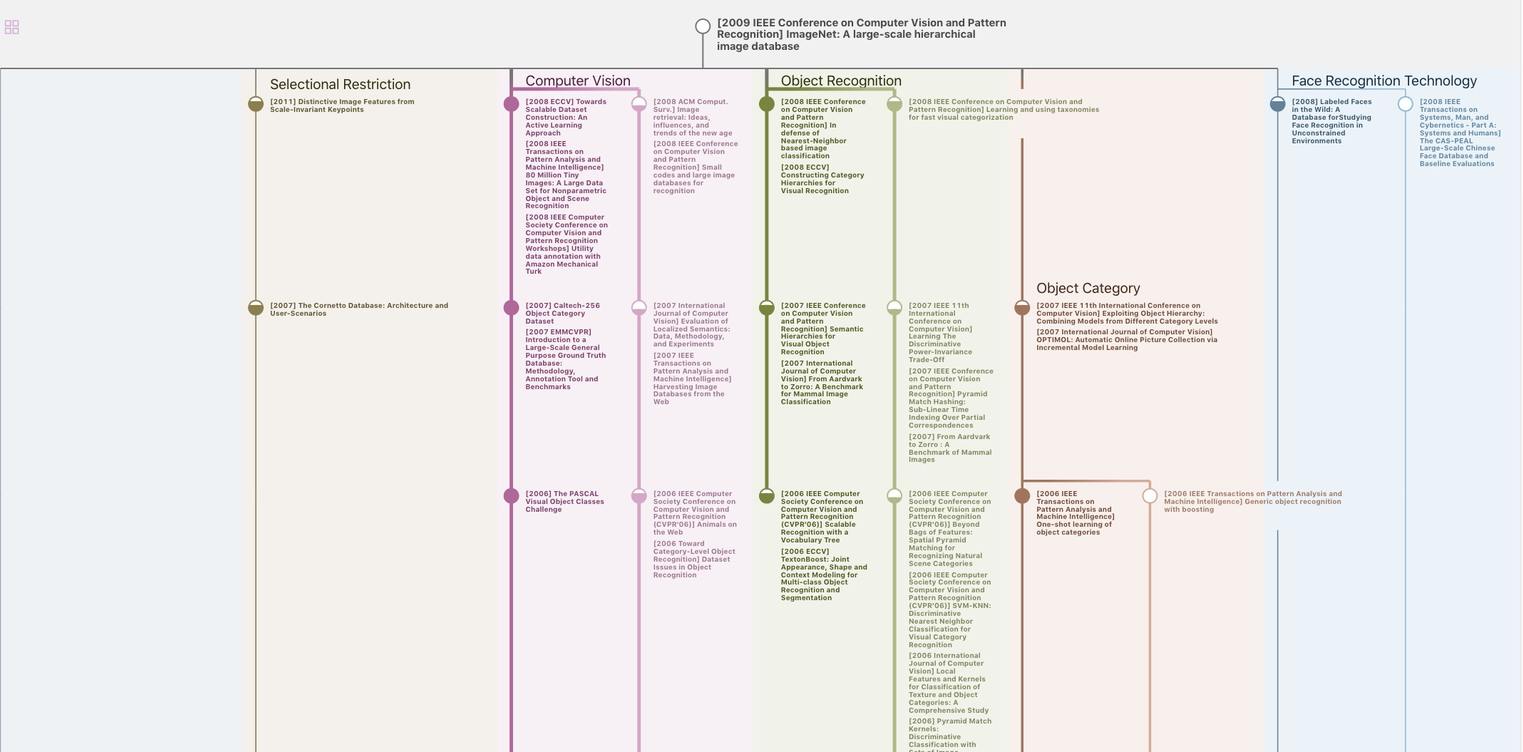
生成溯源树,研究论文发展脉络
Chat Paper
正在生成论文摘要