Data-driven discovery of a formation prediction rule on high-entropy ceramics
ACTA MATERIALIA(2023)
摘要
The interest in high entropy ceramics (HECs) has increased steadily due to their superior properties. However, the prediction of their formation still poses challenges for the discovery of new systems. Here, we discover a rational rule for designing single-phase high entropy transition metal diborides (HEBs) using data-driven approach. The machine learning (ML) model is trained on data collected via high-throughput experiments (HTEs). K nearest neighbors (KNN) model shows an experimental validation accuracy of 93.75%. By imple-menting interpretable ML method, we demonstrate that a mismatch of the bonds between boron and transition metals (delta B_TM) dominates the formation of HEBs. We propose an empirical rule that HEBs favor forming a single phase when delta B_TM < 3.66; otherwise, multiphase. The rule has a high accuracy of 93.33% for new HEBs pre-dictions. In addition, we contribute 165 high quality HEBs data in total, which can promote the development of materials informatics in HEBs. Moreover, this data-driven strategy can be expanded to accelerate the search for new HECs, paving a pathway to design novel HECs with superior properties rapidly.
更多查看译文
关键词
formation prediction rule,ceramics,discovery,data-driven,high-entropy
AI 理解论文
溯源树
样例
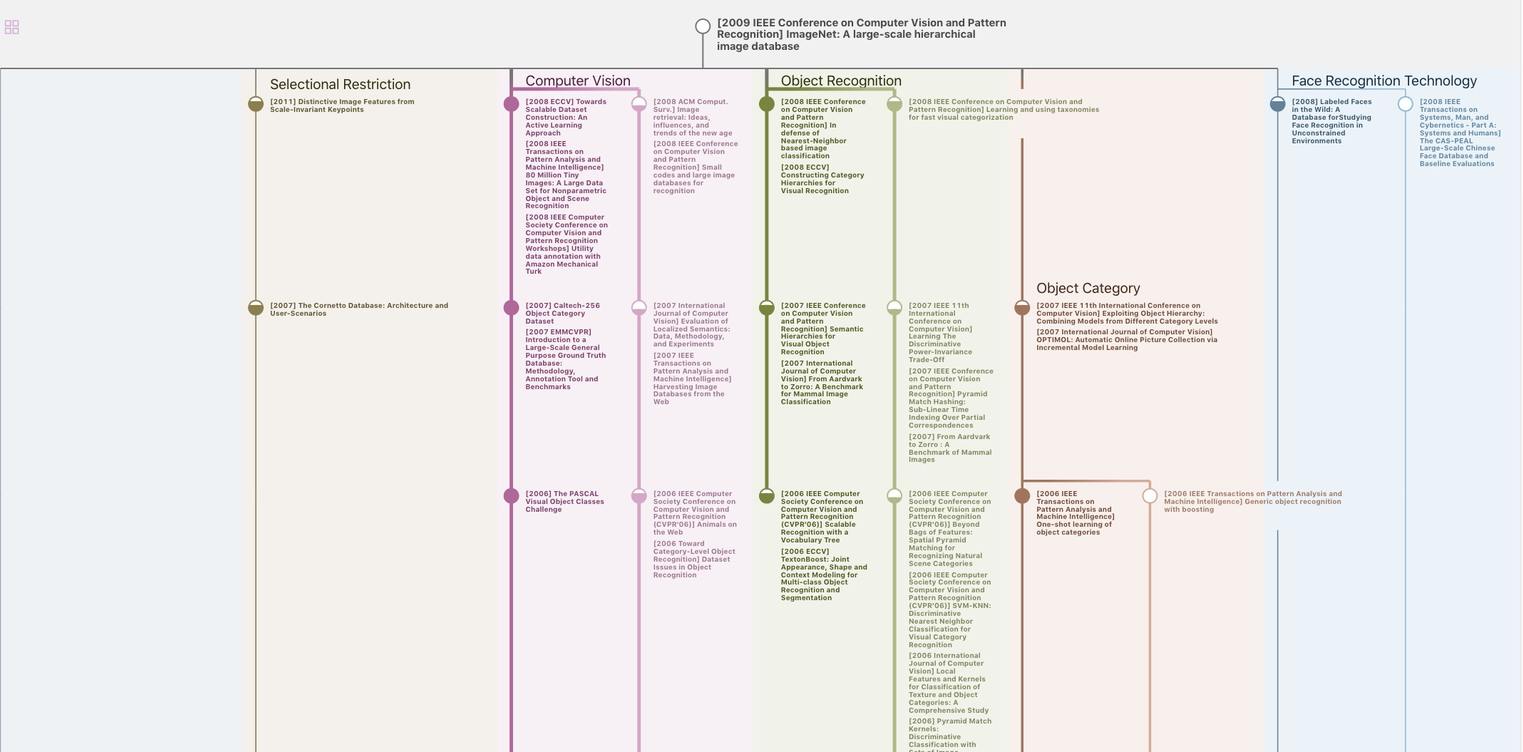
生成溯源树,研究论文发展脉络
Chat Paper
正在生成论文摘要