Machine learning for shock compression of solids using scarce data
JOURNAL OF APPLIED PHYSICS(2023)
Abstract
Data-driven machine learning techniques can be useful for the rapid evaluation of material properties in extreme environments, particularly in cases where direct access to the materials is not possible. Such problems occur in high-throughput material screening and material design approaches where many candidates may not be amenable to direct experimental examination. In this paper, we perform an exhaustive examination of the applicability of machine learning for the estimation of isothermal shock compression properties, specifically the shock Hugoniot, for diverse material systems. A comprehensive analysis is conducted where effects of scarce data, variances in source data, feature choices, and model choices are systematically explored. New modeling strategies are introduced based on feature engineering, including a feature augmentation approach, to mitigate the effects of scarce data. The findings show significant promise of machine learning techniques for design and discovery of materials suited for shock compression applications.
MoreTranslated text
Key words
shock compression,machine learning,solids
AI Read Science
Must-Reading Tree
Example
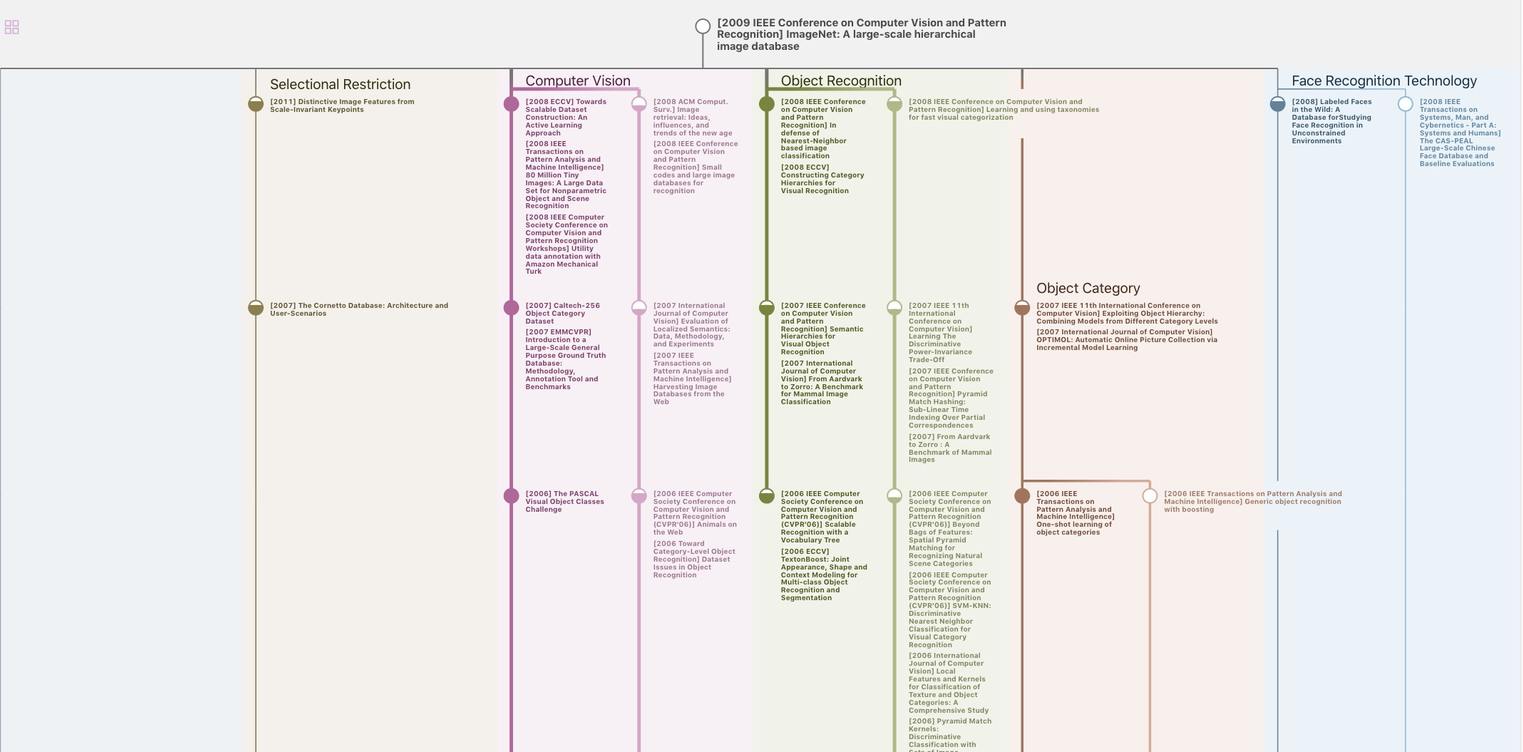
Generate MRT to find the research sequence of this paper
Chat Paper
Summary is being generated by the instructions you defined