Implicit function-based continuous representation for meticulous segmentation of cracks from high-resolution images
COMPUTER-AIDED CIVIL AND INFRASTRUCTURE ENGINEERING(2024)
摘要
High-resolution (HR) crack images offer more detailed information for evaluating the structural condition and formulating effective maintenance or rehabilitation plans. However, the meticulous segmentation of HR crack images has been a challenge due to the limitations of mainstream deep learning algorithms that extract features in a discrete manner, as well as the constraints of computing resources. To address this issue, a novel implicit function-integrated architecture, called the crack continuous refinement network (CCRN), was proposed for meticulous segmentation of cracks from HR images using a continuous representation manner. First, a crack continuous alignment module with a position encoding function was proposed to encode the tiny crack pixels that are easily lost in the sampling process. Then, a lightweight decoder embedded with implicit functions was customized to recover crack details from the aligned latent features and continuous position encoding information. Afterward, the gap between low-resolution training images and HR inference results was bridged by the proposed continuous inference strategy. Finally, the robustness and practicability of the well-trained CCRN were demonstrated by a parallel comparison and an unmanned aerial vehicle-based field experiment.
更多查看译文
AI 理解论文
溯源树
样例
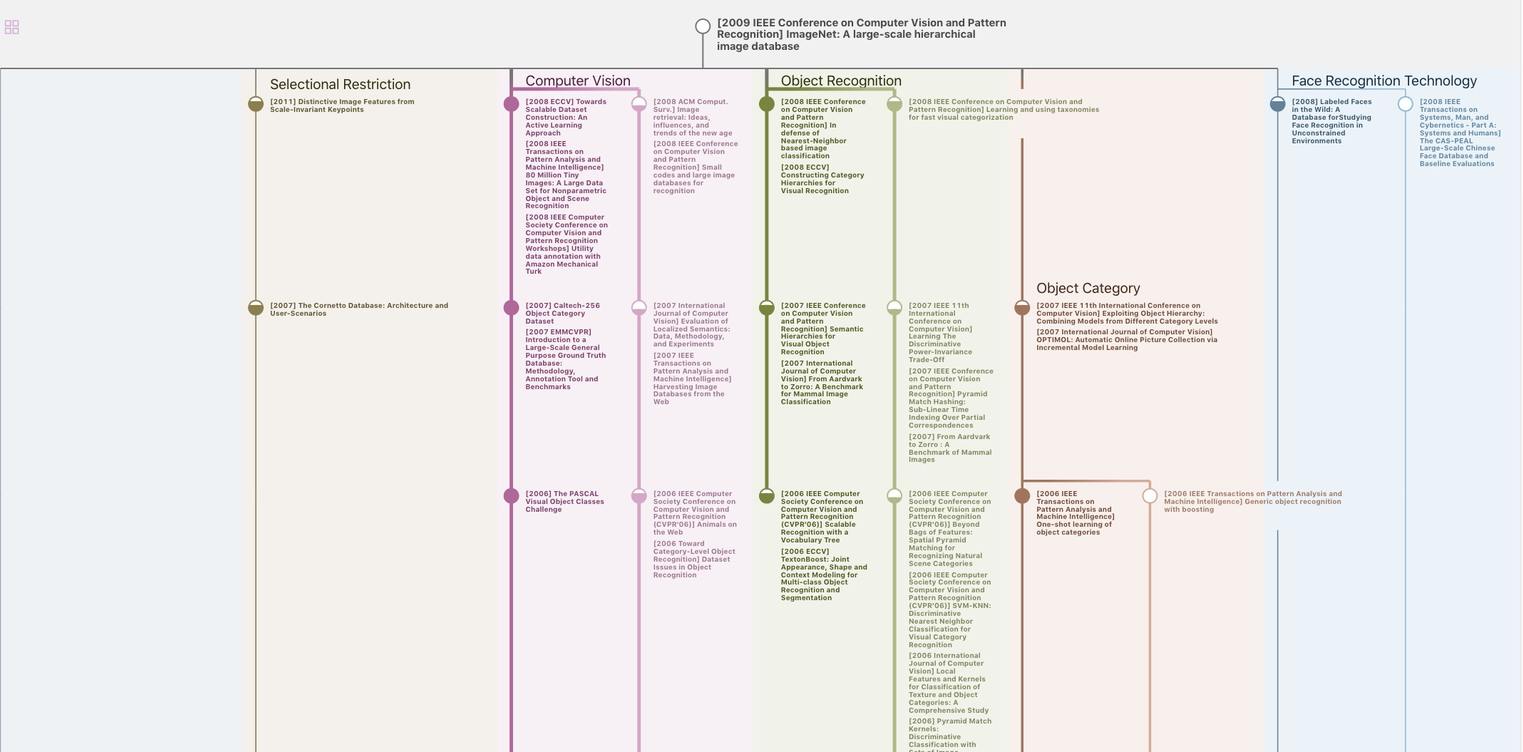
生成溯源树,研究论文发展脉络
Chat Paper
正在生成论文摘要