A deep natural language processing-based method for ontology learning of project-specific properties from building information models
COMPUTER-AIDED CIVIL AND INFRASTRUCTURE ENGINEERING(2024)
摘要
Element property is a crucial aspect of building information modeling (BIM) for almost all BIM-based engineering tasks. Since there are limited properties predefined in Industry Foundation Classes (IFC) specifications, a vast number of property concepts were customized and stored in BIM models, which lack labor-intensive data modeling and alignment for effective information management and reuse. To tackle the challenge, this study presents a natural language understanding (NLU)-based method for the automatic ontological knowledge modeling of project-specific property concepts from BIM models. A soft pattern matching model was used to acquire contextual definitions of concepts from a domain corpus before applying deep NLU models to transform the concept names and definitions into dense vector representations. These outputs were then fed into two stacking ensemble learning models to carry out two tasks: (a) classifying whether an unseen concept overlaps with the IFC ontology, and (b) aligning the repetitive concepts with the most relevant concepts in the ontology. Finally, all fresh properties were appended to an IFC ontology, either as new objects or new synonyms. The performance was evaluated based on 327 property concepts from real-life BIM models. The results show that the proposed approach incorporating reading comprehension of definitions outperforms the existing name similarity-based methods. Finally, a case study on a renovation project demonstrates the effectiveness of this study in automatic ontology modeling of property concepts.
更多查看译文
AI 理解论文
溯源树
样例
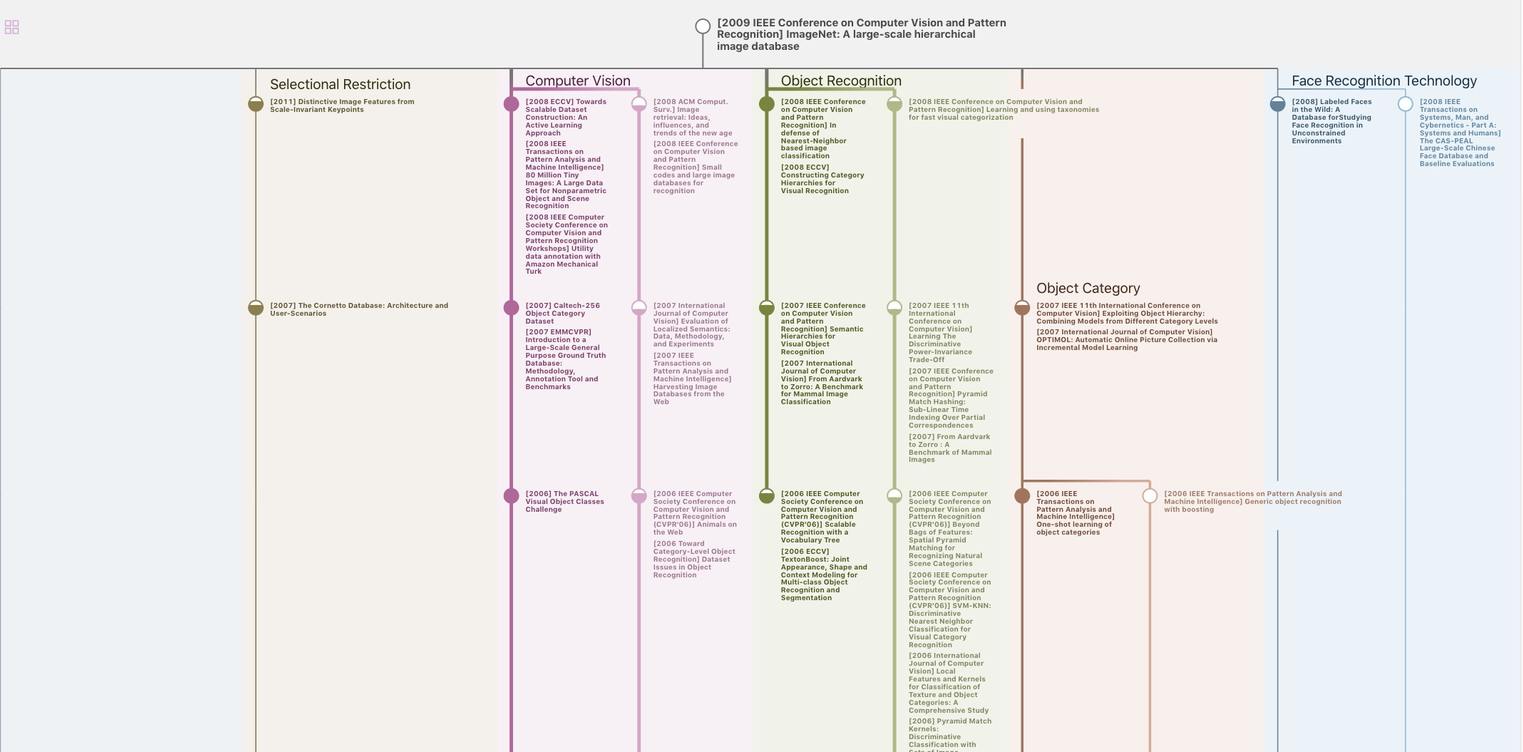
生成溯源树,研究论文发展脉络
Chat Paper
正在生成论文摘要