A Generalizable Deepfake Detector based on Neural Conditional Distribution Modelling.
2020 International Conference of the Biometrics Special Interest Group (BIOSIG)(2020)
摘要
Photo- and video-realistic generation techniques have become a reality following the advent of deep neural networks. Consequently, there are immense concerns regarding the difficulty in differentiating what content is real from what is synthetic. An example of video-realistic generation techniques is the infamous Deepfakes, which exploit the main modality by which humans identify each other. Deepfakes are a category of synthetic face generation methods and are commonly based on generative adversarial networks. In this article, we propose a novel two-step synthetic face image detection method in which general-purpose features are extracted in a first step, trivializing the task of detecting synthetic images. The anomaly detector predicts the conditional probabilities for observing every individual pixel in the image and is trained on pristine data only. The extracted anomaly features demonstrate true generalization capacity across widely different unknown synthesis methods while showing a minimal loss in performance with regard to the detection of known synthetic samples.
更多查看译文
关键词
Deepfake,Video Forensics,Generative Adversarial Networks,PixelCNN,Universal Background Model
AI 理解论文
溯源树
样例
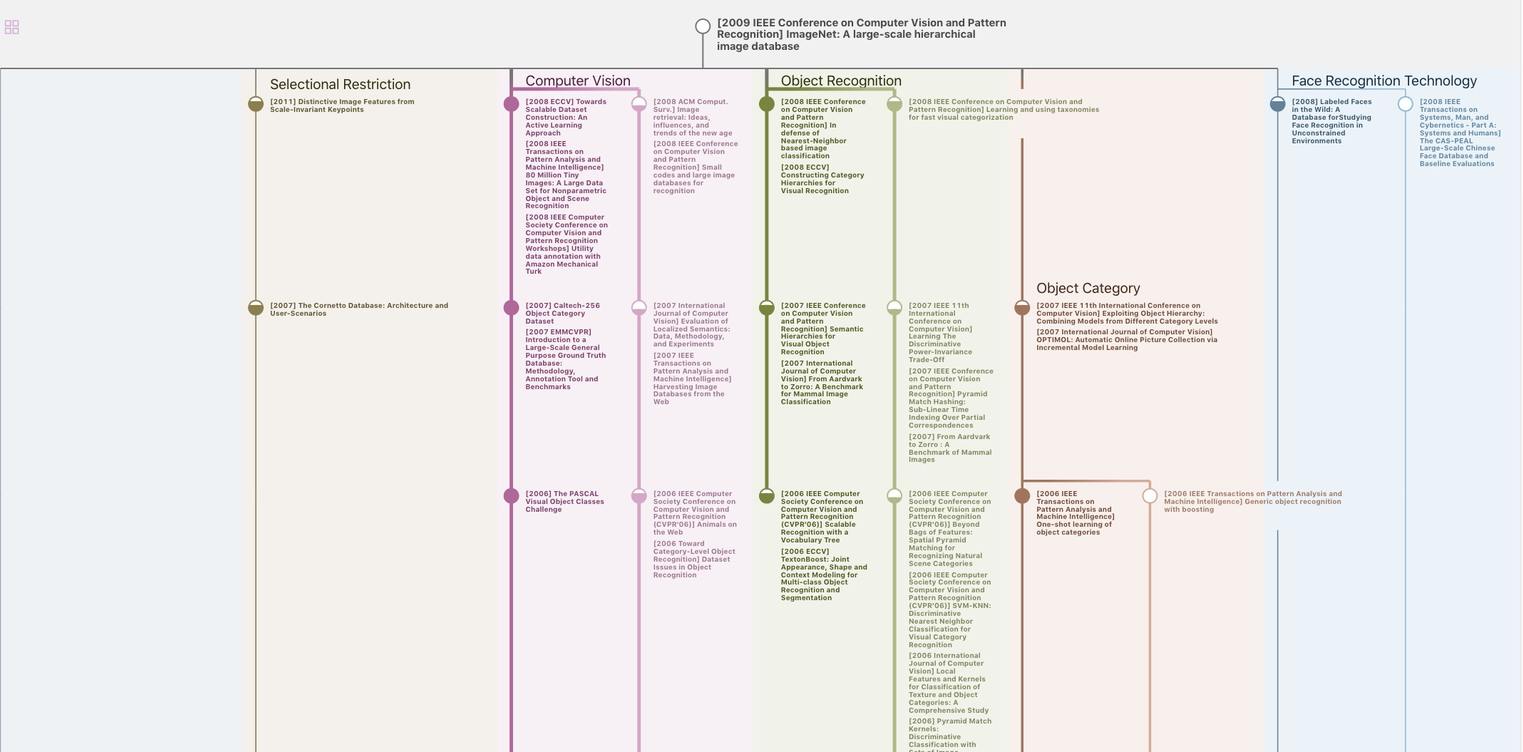
生成溯源树,研究论文发展脉络
Chat Paper
正在生成论文摘要