Bridge seismic fragility model based on support vector machine and relevance vector machine
STRUCTURES(2023)
摘要
In the area of bridge seismic fragility studies, the fragility curve is commonly defined as a log-normal cumulative distribution function, and its parameters are usually estimated through two methods. However, the fragility curves derived by one of these two methods may intersect, whereas the other imposes significant restrictions on the shape of the curves. The purpose of this study is to derive fragility curves by utilizing machine learning tools (in particular, tools that are based on multi-classification probabilistic prediction), to address the limitations that are present in existing methodologies. To be more precise, support vector machine for classification (SVC) and relevance vector machine for classification (RVC) are utilized to construct fragility curves, while two other classic methodologies are chosen for comparison and validation. The four approaches were tested on a dataset acquired using numerical analysis, and their performance was measured using Brier -Score and Log-Loss. The results show that both SVC and RVC not only overcome the limitations and outperform the classical method, but also show robustness to arbitrarily partitioned training sets, whereas the classical method sometimes performs poorly.
更多查看译文
关键词
Bridge seismic fragility,Machine learning tools,Support vector machine,Relevance vector machine,Multiclass classification,Probabilistic outputs
AI 理解论文
溯源树
样例
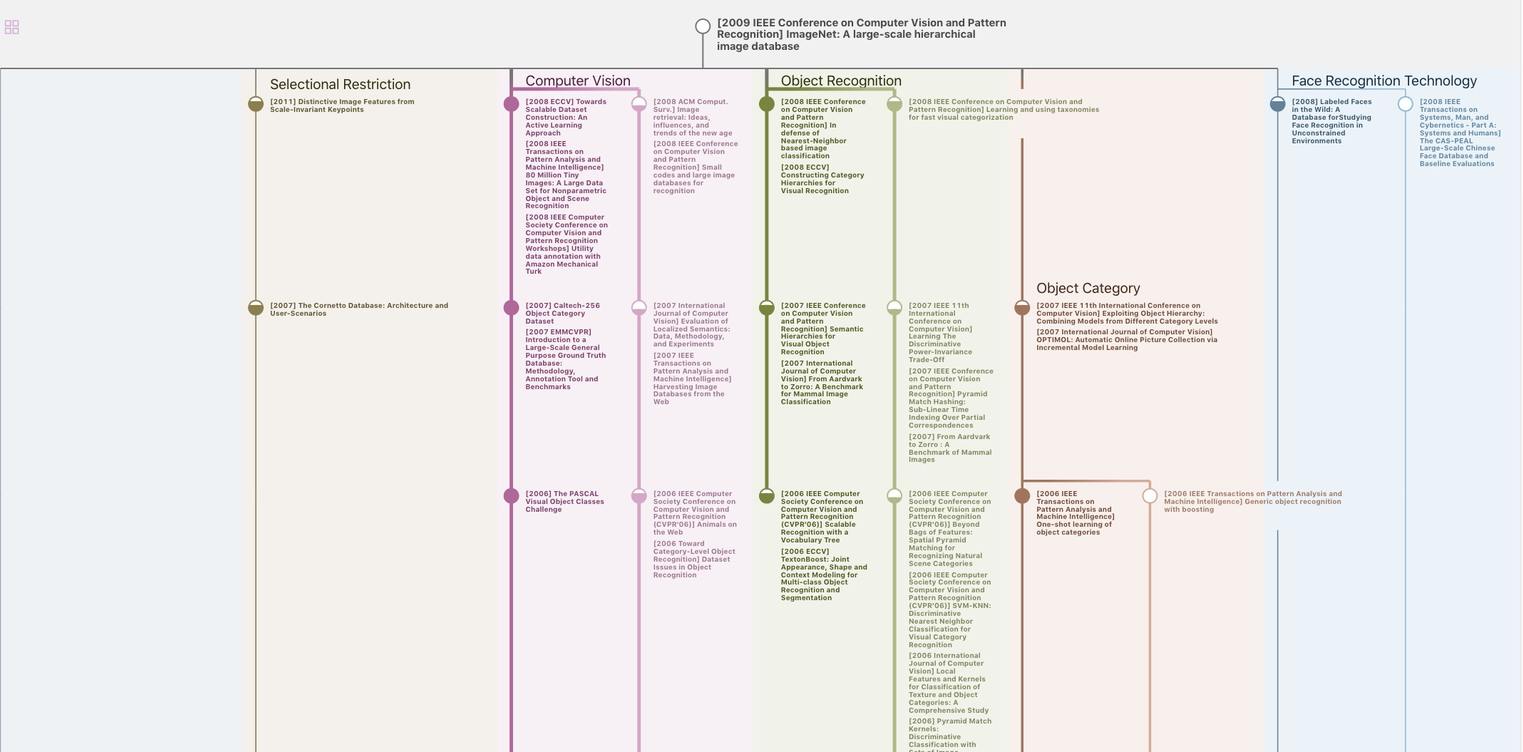
生成溯源树,研究论文发展脉络
Chat Paper
正在生成论文摘要