Particle Size Distribution at Ocean Station Papa from Nanometers to Millimeters Constrained with Intercomparison of Seven Methods
ELEMENTA-SCIENCE OF THE ANTHROPOCENE(2023)
Key words
Particle size distribution,Light scattering,ViewSizer,IFCB,UVP,Coulter Counter
AI Read Science
Must-Reading Tree
Example
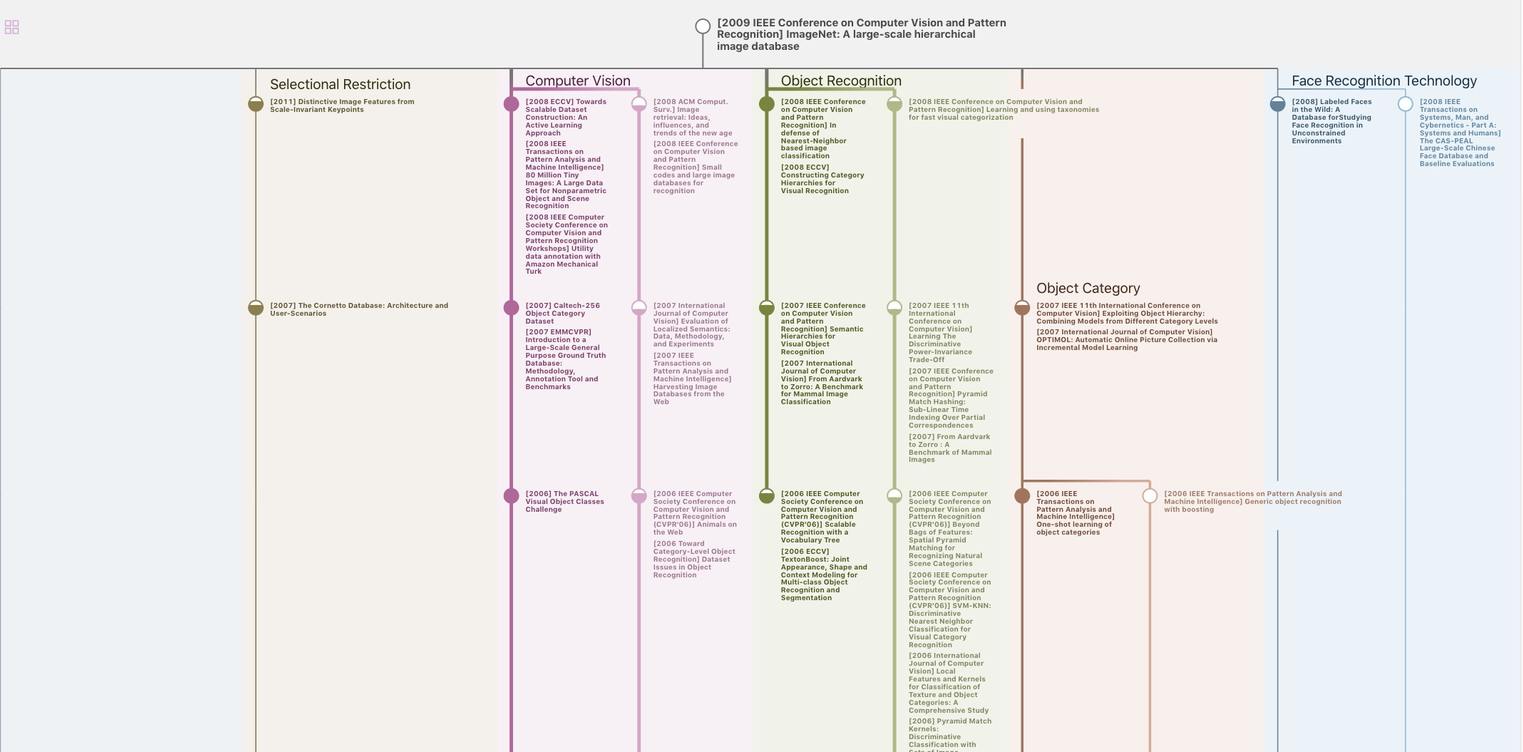
Generate MRT to find the research sequence of this paper
Chat Paper
Summary is being generated by the instructions you defined