A collaborative quasi-Monte Carlo uncertainty propagation analysis method for multiple types of epistemic uncertainty quantified by probability boxes
STRUCTURAL AND MULTIDISCIPLINARY OPTIMIZATION(2023)
摘要
Various epistemic uncertainties arise in practical engineering problems. In this paper, probability boxes (p-boxes) are used to unify multiple types of epistemic uncertainty, which include intervals, p-boxes and evidence variables. A collaborative interval quasi-Monte Carlo method (CIMCM) is presented to calculate the probability bounds of a model. The interval-based quasi-random sampling technique is applied to sample a range of intervals from unified input p-boxes. An interval that includes all random intervals is constructed. Rosen’s gradient projection method (RGPM) is utilized to solve the extreme output values of the numerical model based on the enveloping interval. A collaborative optimization method is presented to calculate the extreme values of the numerical model, the input variables of which are a range of intervals. The probability bounds of the model are finally computed by a statistical method. The presented method reduces the number of repeated search iterations for a range of optimization problems that include many overlapping domains. Two numerical examples and two engineering cases are investigated to demonstrate the effectiveness of the CIMCM.
更多查看译文
关键词
epistemic uncertainty,propagation,quasi-monte
AI 理解论文
溯源树
样例
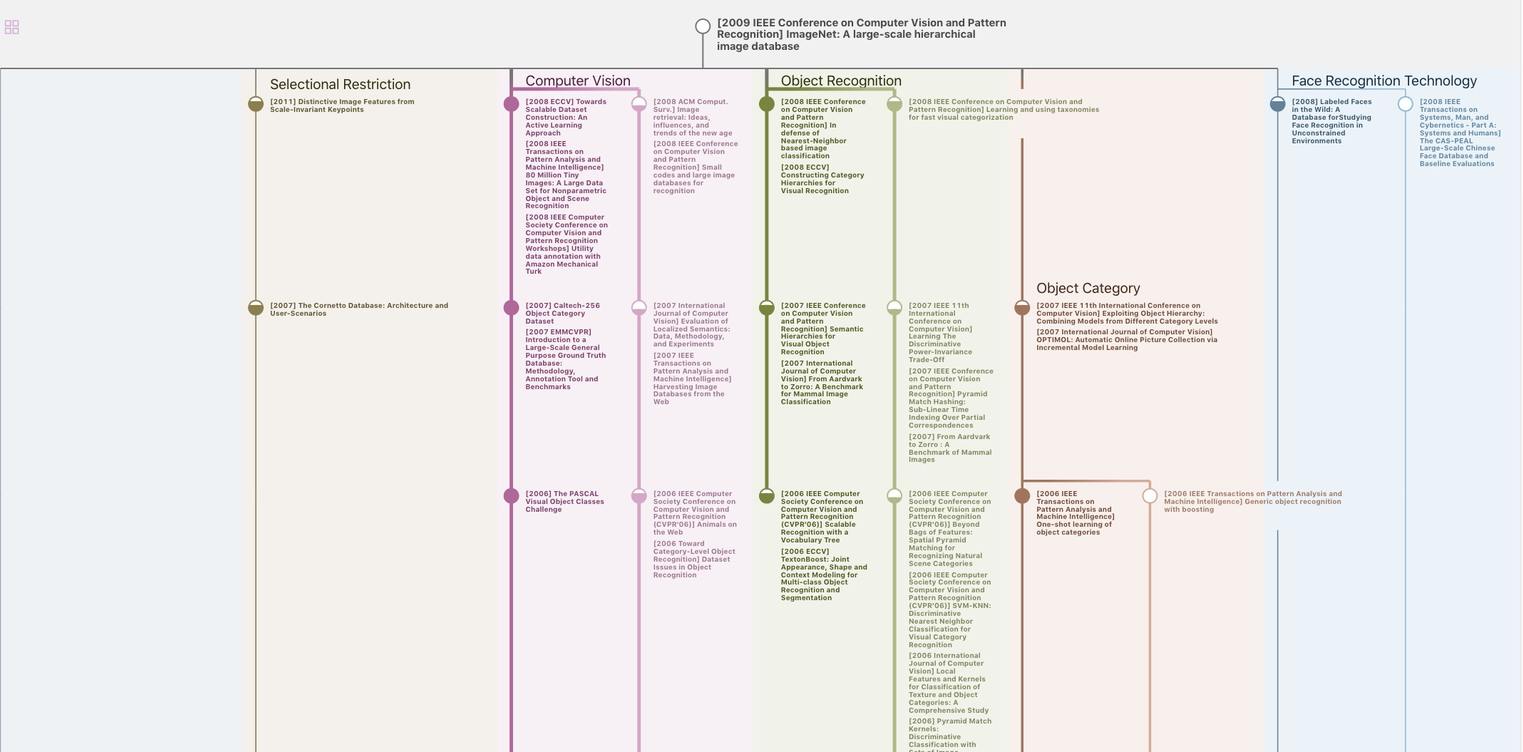
生成溯源树,研究论文发展脉络
Chat Paper
正在生成论文摘要