An unsupervised cyberattack detection scheme for AC microgrids using Gaussian process regression and one-class support vector machine anomaly detection
IET RENEWABLE POWER GENERATION(2023)
摘要
This paper addresses the cybersecurity of hierarchical control of AC microgrids with distributed secondary control. The false data injection (FDI) cyberattack is assumed to alter the operating frequency of inverter-based distributed generators (DGs) in an islanded microgrid. For the microgrids consisting of the grid-forming inverters with the secondary control operating in a distributed manner, the attack on one DG deteriorates not only the corresponding DG but also the other DGs that receive the corrupted information via the distributed communication network. To this end, an FDI attack detection algorithm based on a combination of Gaussian process regression and one-class support vector machine (OC-SVM) anomaly detection is introduced. This algorithm is unsupervised in the sense that it does not require labelled abnormal data for training which is difficult to collect. The Gaussian process model predicts the response of the DG, and its prediction error and estimated variances provide input to an OC-SVM anomaly detector. This algorithm returns enhanced detection performance than the standalone OC-SVM. The proposed cyberattack detector is trained and tested with the data collected from a 4 DG microgrid test model and is validated in both simulation and hardware-in-the-loop testbeds.
更多查看译文
关键词
cyber-physical systems,distributed control,Gaussian processes,microgrids,support vector machines
AI 理解论文
溯源树
样例
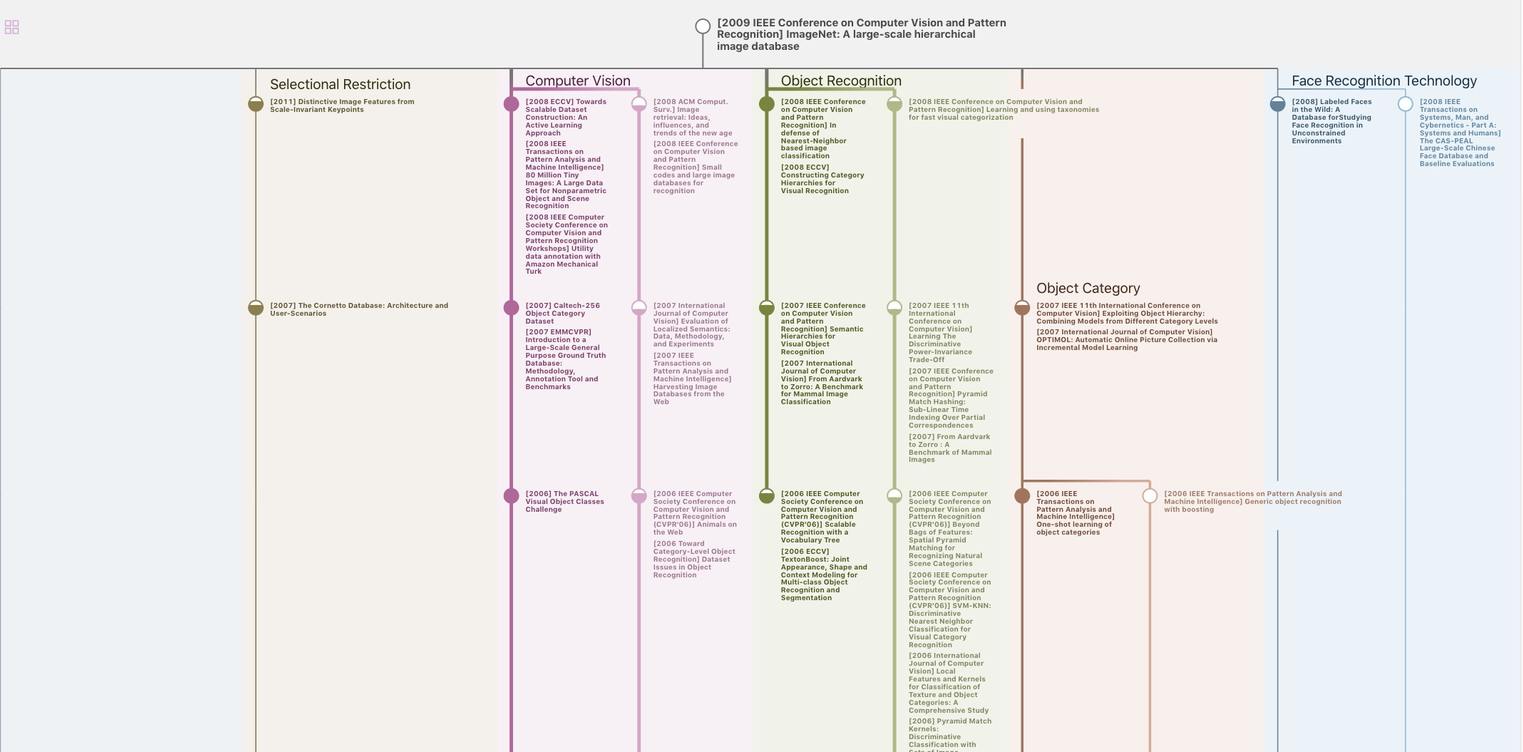
生成溯源树,研究论文发展脉络
Chat Paper
正在生成论文摘要