Reconstructive Spectrum Analyzer with High-Resolution and Large-Bandwidth Using Physical-Model and Data-Driven Model Combined Neural Network
LASER & PHOTONICS REVIEWS(2023)
摘要
Most neural networks (NNs) used for reconstructive spectrum analyzers (RSAs) rely on data-driven training strategies, which can be time-consuming due to the need for a large training dataset with a limited amount of output channels. Here, a specially designed NN is proposed for a reconstructive wavemeter based on temporal speckle obtained from a whispering gallery mode (WGM) resonator. By combining a physical model and data-driven model, it only takes 10 mu s to obtain a reference speckle for the generation of a training dataset. The WGM resonator-based wavemeter assisted by the NN uses only one photo-detector to obtain a temporal speckle, achieving a spectral resolution of 3.2 fm. The number of output channels reaches 2300, which is the largest dynamic range achieved by an NN in RSA without the need for re-training. It demonstrates that the proposed NN has capability to resolve unseen spectrum with multi-tone wavelengths. Moreover, the proposed network exhibits better robustness in long-time measurement compared to data-driven model based networks. This opens up new possibilities for NN design methods in RSA, without the need for a large training dataset, by incorporating a physical model to achieve high-resolution, high-dynamic-range, and fast-speed spectrum measurement.
更多查看译文
关键词
spectrum,physical‐model combined neural network,high‐resolution high‐resolution
AI 理解论文
溯源树
样例
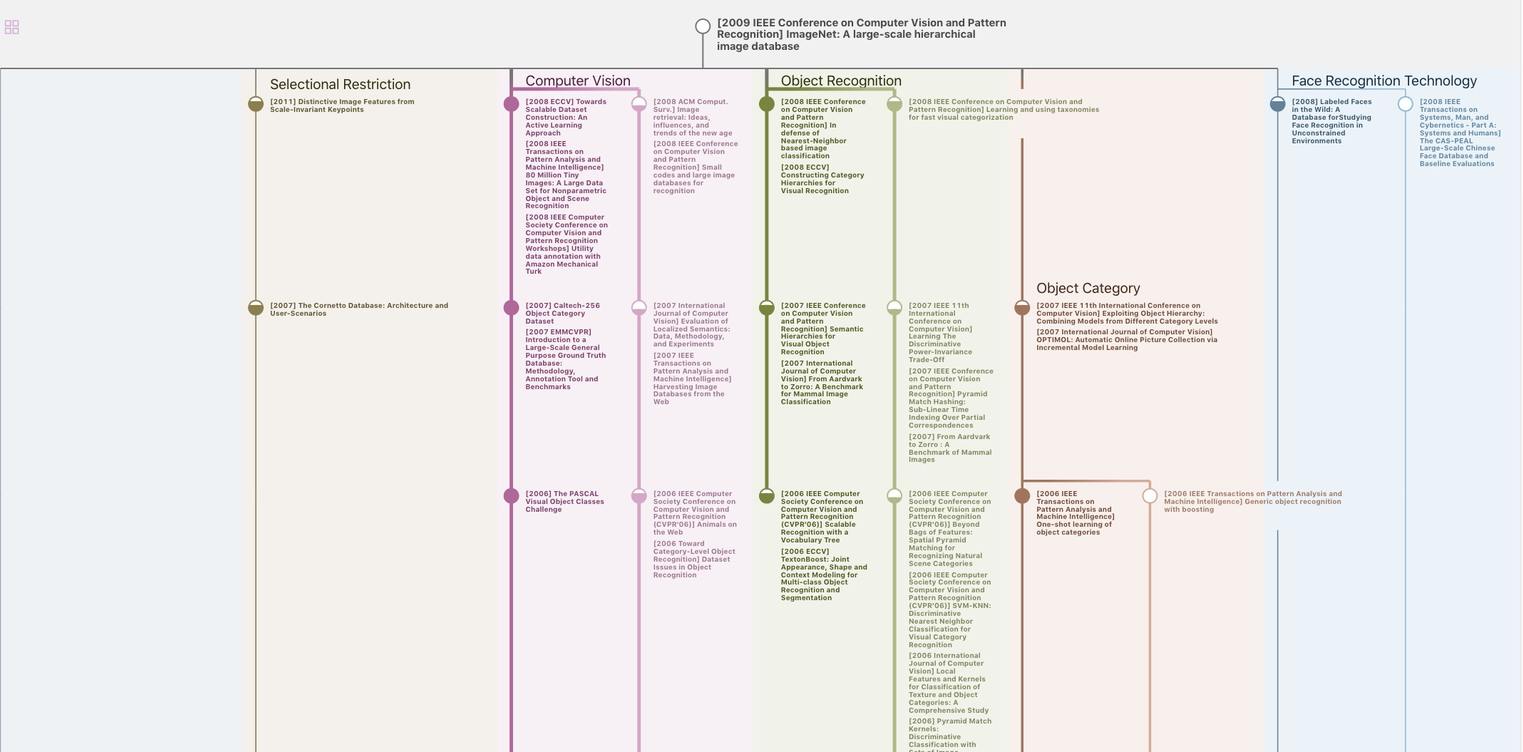
生成溯源树,研究论文发展脉络
Chat Paper
正在生成论文摘要