A LightGBM-based landslide susceptibility model considering the uncertainty of non-landslide samples
GEOMATICS NATURAL HAZARDS & RISK(2023)
Abstract
The quality of samples is crucial in constructing a data-driven landslide susceptibility model. This article aims to construct a data-driven landslide susceptibility model that takes into account the selection of non-landslide samples. First, 21 conditioning factors are selected, including four types of topography and landform, geological conditions, environmental conditions, and human activities. Grid units with 30 m resolution are established by combining 942 historical landslide events in study area. Second, non-landslide samples are selected using both the traditional method and the information quantity method. Two landslide susceptibility models are established using the Bayesian optimization-LightGBM model. The accuracy of the model is evaluated by significance test and the area under curve (AUC). Finally, the SHAP algorithm is used to analyse the internal mechanism of the model's decision-making. Based on the information quantity method, the LightGBM model identifies very high-high susceptibility areas that account for 77.92% of the total number of landslides. Additionally, the AUC of test set and the AUC of training set are 23.2% and 17.1% higher, respectively, compared to the traditional model. The selection of different sample data, whether landslide or non-landslide, impacts the factor rank, model accuracy, and the interal decision-making mechanism of the model. This finding provides valuable for the selection of sample data in the binary classification model.
MoreTranslated text
Key words
Information quantity method,Light Gradient Boosting Machine (LightGBM),Bayesian optimization,box plot diagram,SHapley Additive exPlanation (SHAP)
AI Read Science
Must-Reading Tree
Example
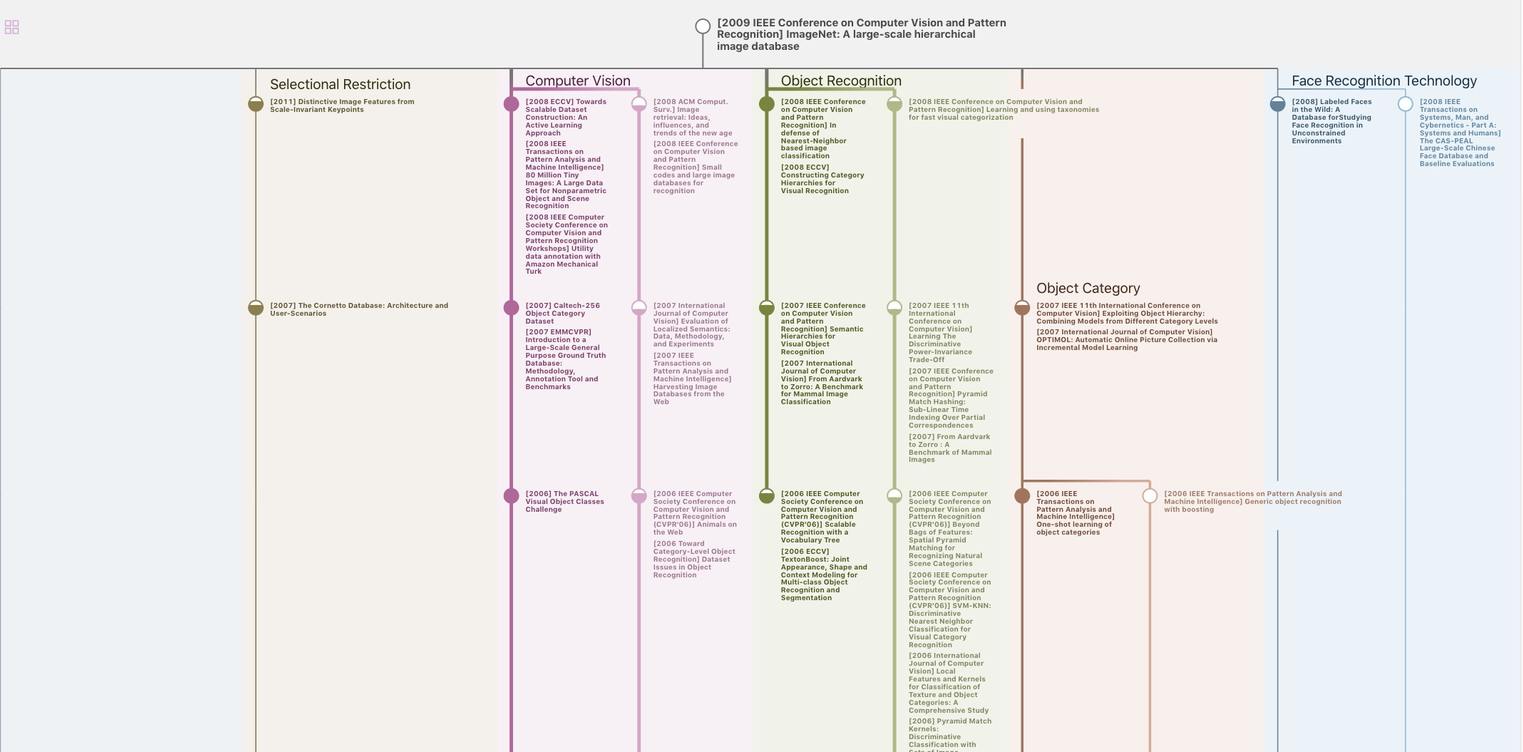
Generate MRT to find the research sequence of this paper
Chat Paper
Summary is being generated by the instructions you defined