An Ensemble Learning Method Based on One-Class and Binary Classification for Credit Scoring
JOURNAL OF CIRCUITS SYSTEMS AND COMPUTERS(2023)
摘要
It is crucial to correctly assess whether a potential borrower can repay the loan in the credit scoring model. The credit loan data has a serious data imbalance because the number of defaulters is far less than the nondefaulters. However, most current methods for dealing with data imbalance are designed to improve the classification performance of minority data, which will reduce the performance of majority data. For a financial institution, the economic loss caused by the decrease in the classification performance of nondefaulters (majority data) cannot be ignored. This paper proposes an ensemble learning method based on one-class and binary classification (EMOBC) for credit scoring. The purpose is to improve the classification accuracy of the minority class while mitigating the loss of classification accuracy of the majority class as much as possible. EMOBC uses undersampling for the majority class (nondefault samples in credit scoring) and perform binary-class learning on the balanced data to improve the classification accuracy of the minority. To alleviate the decline in classification performance of the majority class, EMOBC uses one-class and binary collaborative classification to train classifiers. The classification result is determined by the average of one-class and binary-class classifiers. The experimental results show that EMOBC has good comprehensive performance compared with the existing methods.
更多查看译文
关键词
ensemble learning method,binary classification,one-class
AI 理解论文
溯源树
样例
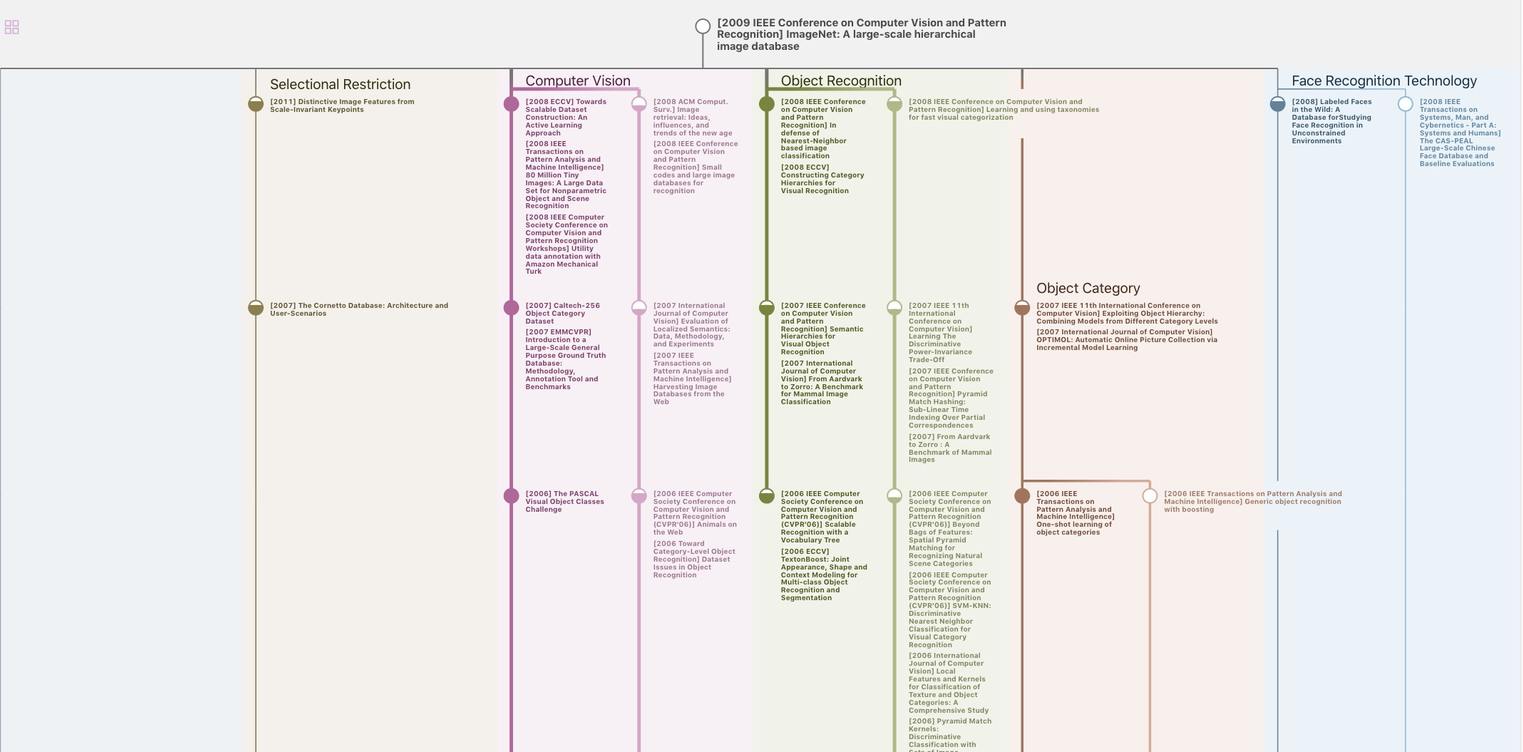
生成溯源树,研究论文发展脉络
Chat Paper
正在生成论文摘要