Pre-Attention Mechanism and Convolutional Neural Network Based Multivariate Load Prediction for Demand Response
ENERGIES(2023)
Abstract
The construction of smart grids has greatly changed the power grid pattern and power supply structure. For the power system, reasonable power planning and demand response is necessary to ensure the stable operation of a society. Accurate load prediction is the basis for realizing demand response for the power system. This paper proposes a Pre-Attention-CNN-GRU model (PreAttCG) which combines a convolutional neural network (CNN) and gate recurrent unit (GRU) and applies the attention mechanism in front of the whole model. The PreAttCG model accepts historical load data and more than nine other factors (including temperature, wind speed, humidity, etc.) as input. The attention layer and CNN layer effectively extract the features and weights of each factor. Load forecasting is then performed by the prediction layer, which consists of a stacked GRU. The model is verified by industrial load data from a German dataset and a Chinese dataset from the real world. The results show that the PreAttCG model has better performance (3 similar to 5% improvement in MAPE) than both LSTM with only load input and LSTM with all factors. Additionally, the experiments also show that the attention mechanism can effectively extract the weights of relevant factors affecting the load data.
MoreTranslated text
Key words
demand response,convolutional neural network,load,pre-attention
AI Read Science
Must-Reading Tree
Example
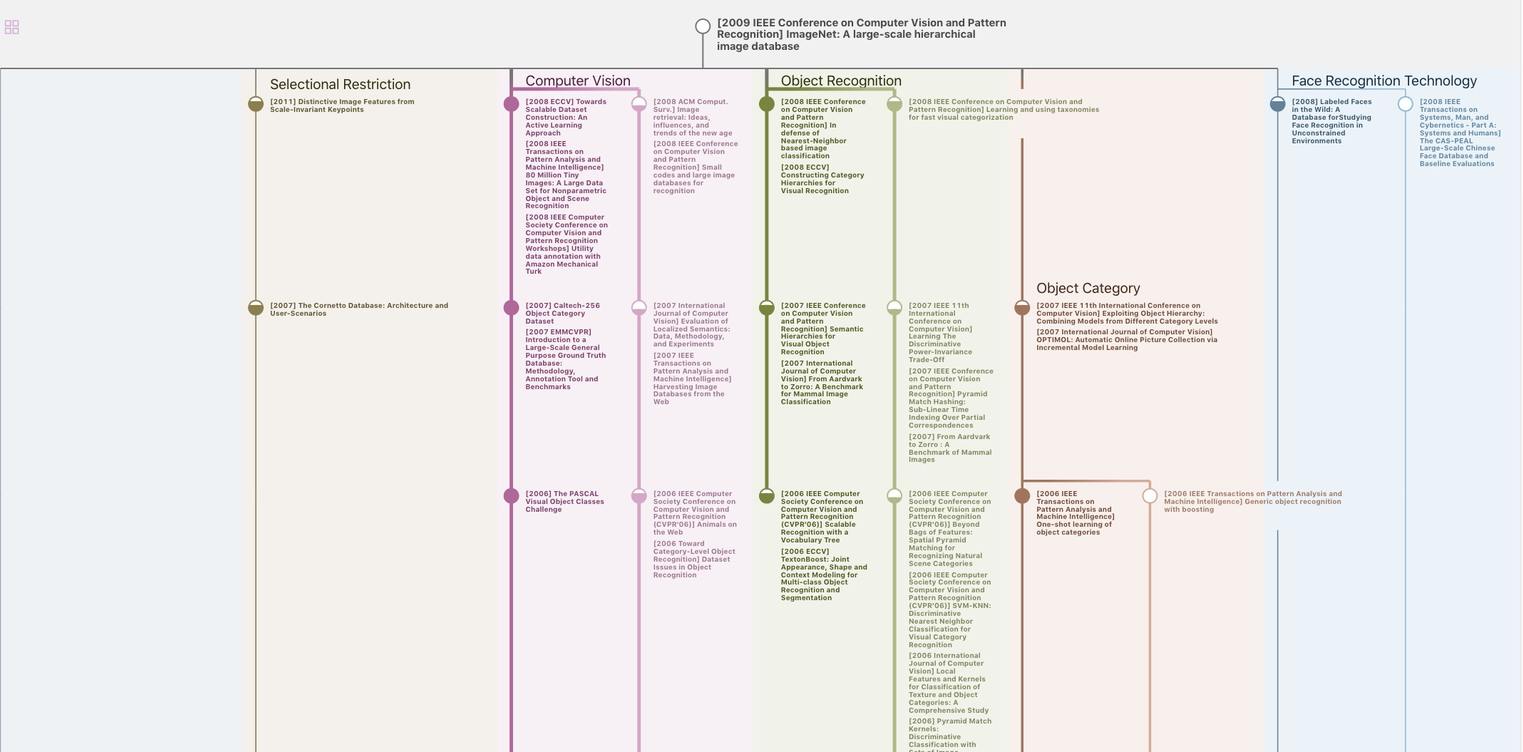
Generate MRT to find the research sequence of this paper
Chat Paper
Summary is being generated by the instructions you defined