Big-Data-Driven Machine Learning for Enhancing Spatiotemporal Air Pollution Pattern Analysis
ATMOSPHERE(2023)
摘要
Air pollution is an important problem for public health. The spatiotemporal analysis is a crucial step for understanding the complex characteristics of air pollution. Using many sensors and high-resolution time-step observations makes this task a big data challenge. In this study, unsupervised machine learning algorithms were applied to analyze spatiotemporal patterns of air pollution. The analysis was conducted using PM10 big data collected from almost 100 sensors located in Krakow, over a period of one year, with data being recorded at 1-h intervals. The analysis results using K-means and SKATER clustering revealed distinct differences between average and maximum values of pollutant concentrations. The study found that the K-means algorithm with Dynamic Time Warping (DTW) was more accurate in identifying yearly patterns and clustering in rapidly and spatially varying data, compared to the SKATER algorithm. Moreover, the clustering analysis of data after kriging greatly facilitated the interpretation of the results. These findings highlight the potential of machine learning techniques and big data analysis for identifying hot-spots, coldspots, and patterns of air pollution and informing policy decisions related to urban planning, traffic management, and public health interventions.
更多查看译文
关键词
machine learning,big-data-driven
AI 理解论文
溯源树
样例
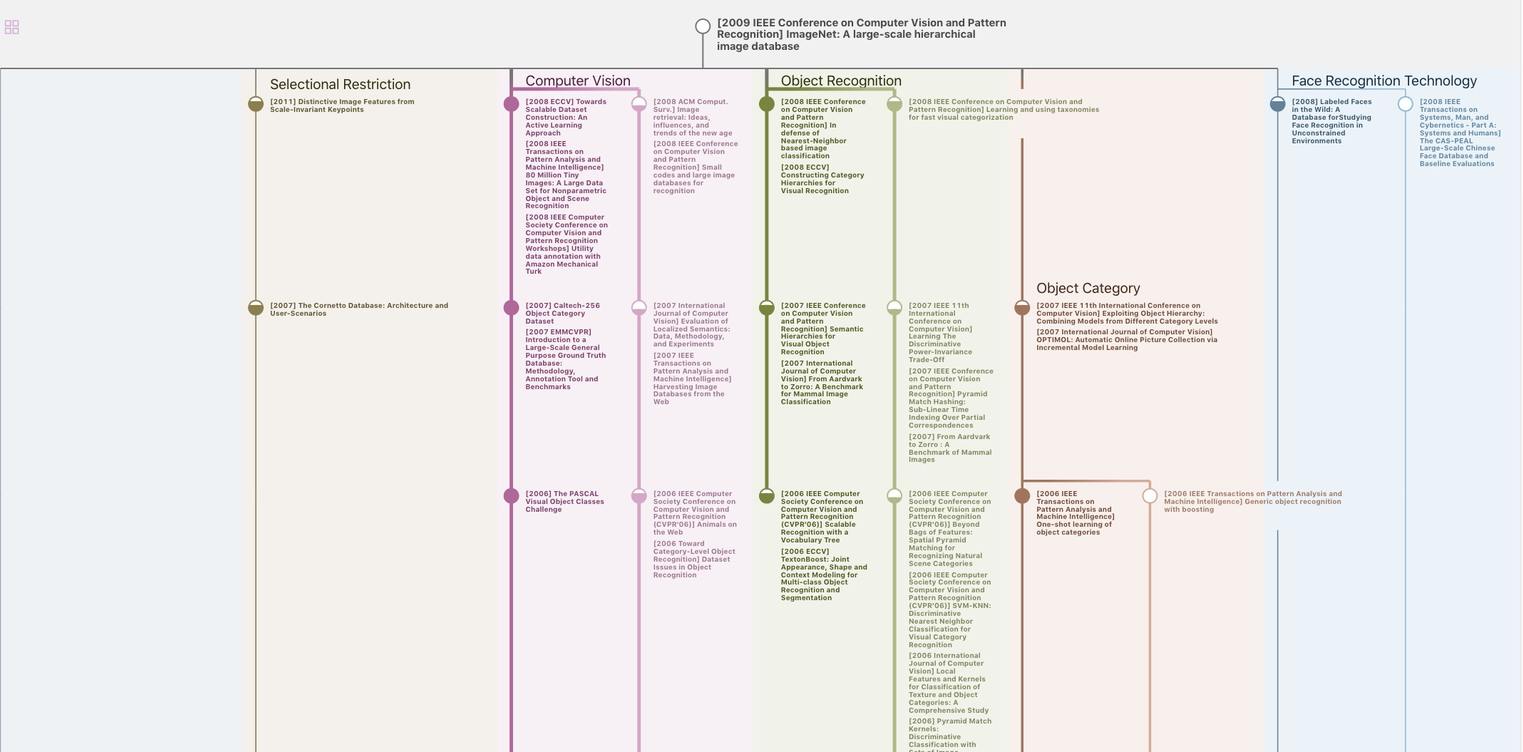
生成溯源树,研究论文发展脉络
Chat Paper
正在生成论文摘要