End-cloud collaboration method enables accurate state of health and remaining useful life online estimation in lithium-ion batteries
JOURNAL OF ENERGY CHEMISTRY(2023)
摘要
Though the lithium-ion battery is universally applied, the reliability of lithium-ion batteries remains a challenge due to various physicochemical reactions, electrode material degradation, and even thermal runaway. Accurate estimation and prediction of battery health conditions are crucial for battery safety management. In this paper, an end-cloud collaboration method is proposed to approach the track of battery degradation process, integrating end-side empirical model with cloud-side data-driven model. Based on ensemble learning methods, the data-driven model is constructed by three base models to obtain cloud-side highly accurate results. The double exponential decay model is utilized as an empirical model to output highly real-time prediction results. With Kalman filter, the prediction results of end-side empirical model can be periodically updated by highly accurate results of cloud-side data-driven model to obtain highly accurate and real-time results. Subsequently, the whole framework can give an accurate prediction and tracking of battery degradation, with the mean absolute error maintained below 2%. And the execution time on the end side can reach 261 ls. The proposed end-cloud collaboration method has the potential to approach highly accurate and highly real-time estimation for battery health conditions during battery full life cycle in architecture of cyber hierarchy and interactional network. (c) 2023 Science Press and Dalian Institute of Chemical Physics, Chinese Academy of Sciences. Published by ELSEVIER B.V. and Science Press. All rights reserved.
更多查看译文
关键词
useful life online estimation,batteries,end-cloud,lithium-ion
AI 理解论文
溯源树
样例
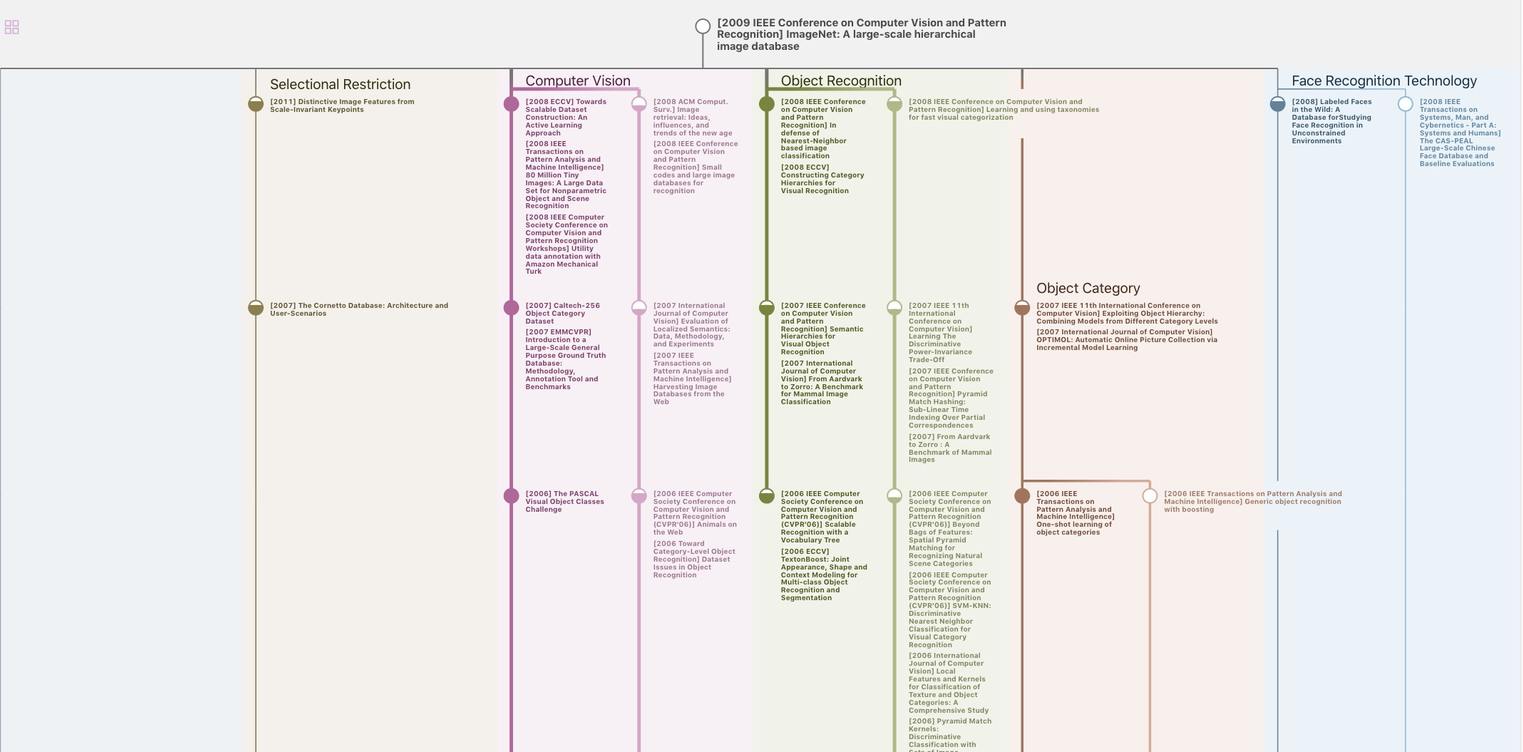
生成溯源树,研究论文发展脉络
Chat Paper
正在生成论文摘要