Optimized deep learning based single-phase broken fault type identification for active distribution networks
ENERGY REPORTS(2023)
摘要
Single-phase broken faults occur frequently, affecting the reliability of distribution network. In order to effectively identify the fault type of single-phase broken fault, this paper proposes a new identification method, which is based on the combination of variational mode decomposition and stacked auto encoder with double optimization (AO-VMD-PSO-SAE). Firstly, the zero sequence voltage, which collected in line, is decomposed into a set of variational modal components. Nextly, the stack automatic encoder is used to conduct unsupervised training on the denoised data to establish a depth learning model, and the AO optimization algorithm and the PSO optimization algorithm are used to determine the super parameters in the model. Finally, simulation results supported and the validity of the method was verified. What the results show is that the proposed model named AO-VMD-PSO-SAE can accurately predict the types of single-phase broken fault under noise interference. (c) 2023 The Author(s). Published by Elsevier Ltd. This is an open access article under the CC BY-NC-ND license (http://creativecommons.org/licenses/by-nc-nd/4.0/). Peer-review under responsibility of the scientific committee of the 2022 The 3rd International Conference on Power Engineering, ICPE, 2022.
更多查看译文
关键词
Single-phase broken fault, Variational mode decomposition, Stacked auto encoder, Distribution network
AI 理解论文
溯源树
样例
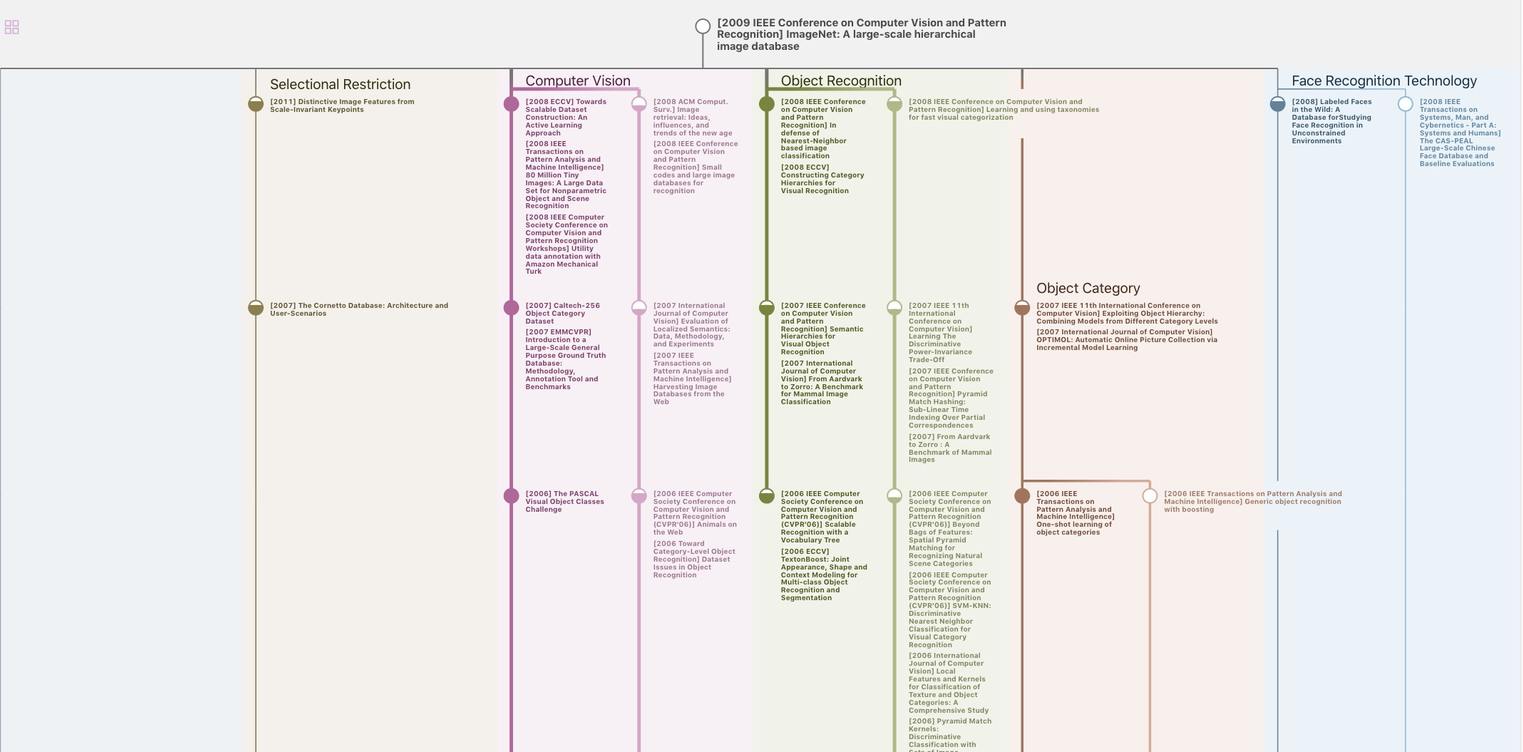
生成溯源树,研究论文发展脉络
Chat Paper
正在生成论文摘要