Fast CO2 saturation simulations on large-scale geomodels with artificial intelligence-based Wavelet Neural Operators
INTERNATIONAL JOURNAL OF GREENHOUSE GAS CONTROL(2023)
摘要
Carbon capture and storage (CCS) projects rely on numerical reservoir simulations for determining where and how much CO2 can safely be stored underground. Conventional simulations are computationally expensive and time consuming, thereby increasing the turn-around time of decision-making processes and projects. Recent research on artificial intelligence (AI) based approaches for solving the underlying two-phase flow equations has shown enormous promise, as the computational burden can be shifted to the training time, while simulations can be performed in fractions of a second at test time. Applications of AI methods for simulating CO2 flow and other differential equations have so far been limited to 2D or small 3D problems with relatively simple geometries. We introduce a cloud-native framework for parallel simulations of large-scale training data sets using the Open Porous Media (OPM) simulator and we propose a new AI architecture based on Fourier Neural Operators (FNOs) with 3D wavelet transforms. This architecture performs better than FNOs on data with shock fronts, such as simulating CO2 saturation. We also provide a new training dataset for CO2 flow based on the Sleipner CO2 storage geomodel from the Norwegian continental shelf and show that both FNOs and Wavelet Neural Operators (WNOs) can be trained on models with over 200,000 cells and lead to significant speed-ups of up to 50,000x (FNOs) and 100x (Wavelet NOs) over numerical simulations of CO2 saturation with OPM.
更多查看译文
关键词
Simulation,CO2,Deep learning,Cloud computing,Sleipner
AI 理解论文
溯源树
样例
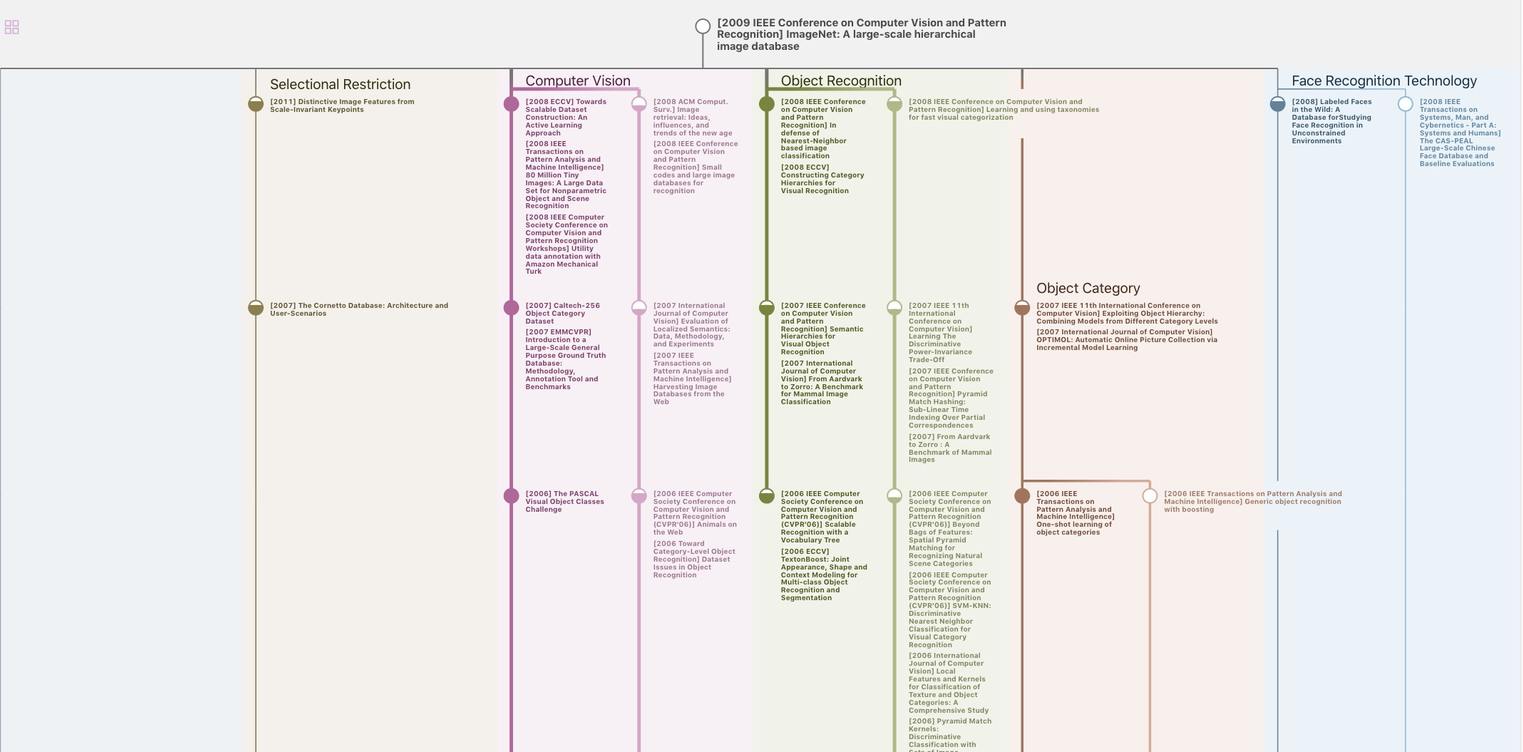
生成溯源树,研究论文发展脉络
Chat Paper
正在生成论文摘要