Cube-Evo: A Query-Efficient Black-Box Attack on Video Classification System
IEEE TRANSACTIONS ON RELIABILITY(2023)
摘要
The current progressive research in the domain of black-box adversarial attack enhances the reliability of deep neural network (DNN)-based video systems. Recent works mainly carry out black-box adversarial attacks on video systems by query-based parameter dimension reduction. However, the additional temporal dimension of video data leads to massive query consumption and low attack success rate. In this article, we embark on our efforts to design an effective adversarial attack on popular video classification systems. We deeply root the observations that the DNN-based systems are sensitive to adversarial perturbations with high frequency and reconstructed shape. Specifically, we propose a systematic attack pipeline Cube-Evo, aiming to reduce the search space dimension and obtain the effective adversarial perturbation via the optimal parameter group updating. We evaluate the proposed attack pipeline on two popular datasets: UCF101 and JESTER. Our attack pipeline reduces query consumption and achieves a high success rate on various DNN-based video classification systems. Compared with the state-of-the-art method Geo-Trap-Att, our pipeline averagely reduces 1.6x query consumption in untargeted attacks and 2.9x in targeted attacks. Besides, Cube-Evo improves 13% attack success rate on average, achieving new state-of-the-art results over diverse video classification systems.
更多查看译文
关键词
Adversarial examples,black-box attack,deep learning,system testing,video classification
AI 理解论文
溯源树
样例
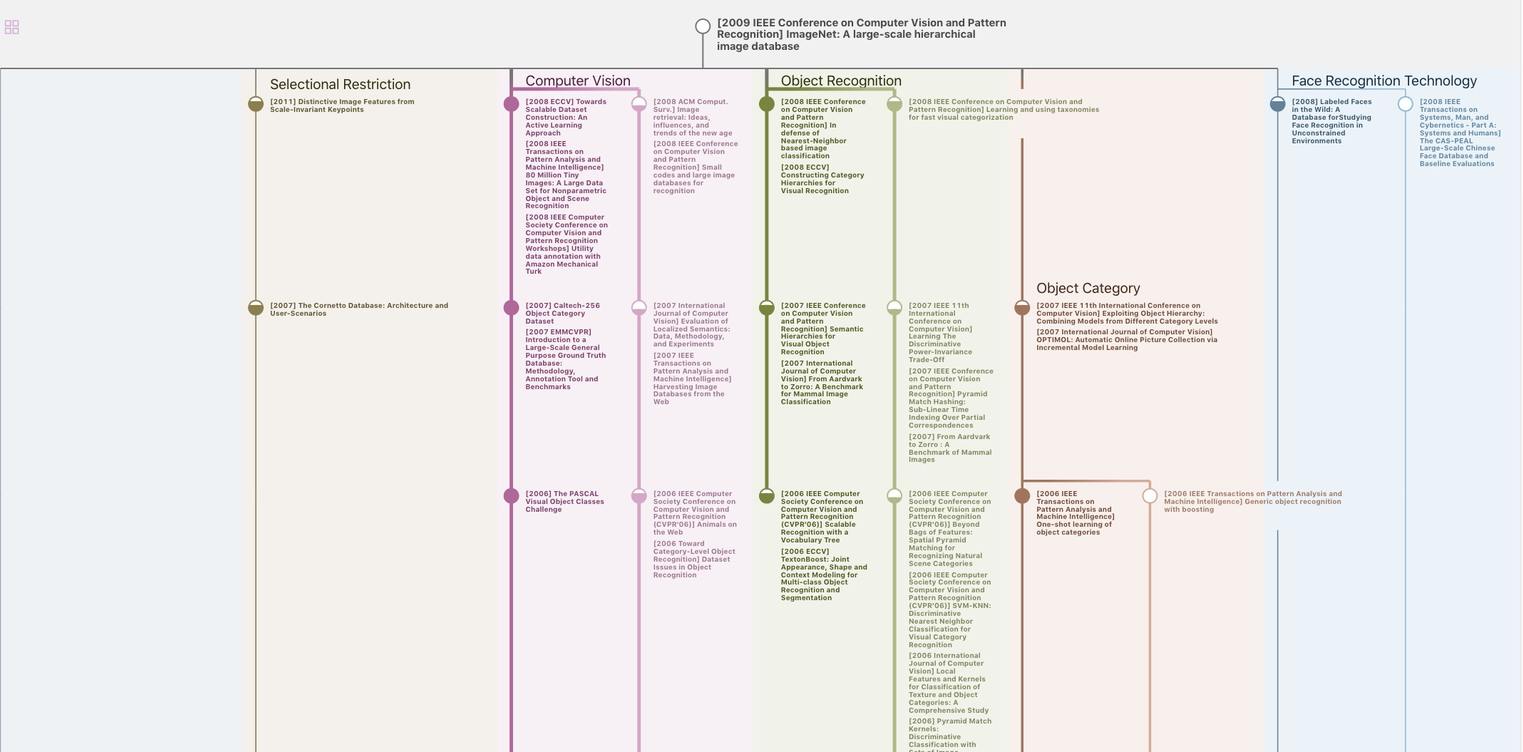
生成溯源树,研究论文发展脉络
Chat Paper
正在生成论文摘要