Multi-View Joint Learning and BEV Feature-Fusion Network for 3D Object Detection
APPLIED SCIENCES-BASEL(2023)
摘要
Traditional 3D object detectors use BEV (bird's eye view) feature maps to generate 3D object proposals, but in a single BEV feature map, there are inevitably the problems of high compression and information loss. To solve this problem, we propose a multi-view joint learning and BEV feature-fusion network. In this network, we mainly propose two fusion modules: the multi-view feature-fusion module and the multi-BEV feature-fusion module. The multi-view feature fusion module performs joint learning from multiple views, fusing features learned from multiple views, and supplementing missing information in the BEV feature map. The multi-BEV feature-fusion module fuses BEV feature map outputs from different feature extractors to further enrich the feature information in the BEV feature map, in order to generate better quality 3D object proposals. We conducted experiments on a widely used KITTI dataset. The results show that our method has significantly improved the detection accuracy of the cyclist category.For cyclist detection tasks at the easy, moderate, and hard levels of the KITTI test dataset, our method improves by 1.57%, 2.03%, and 0.67%, respectively, compared to PV-RCNN.
更多查看译文
关键词
automatic driving,point cloud-based 3D detection,multi-view feature,adaptive feature fusion
AI 理解论文
溯源树
样例
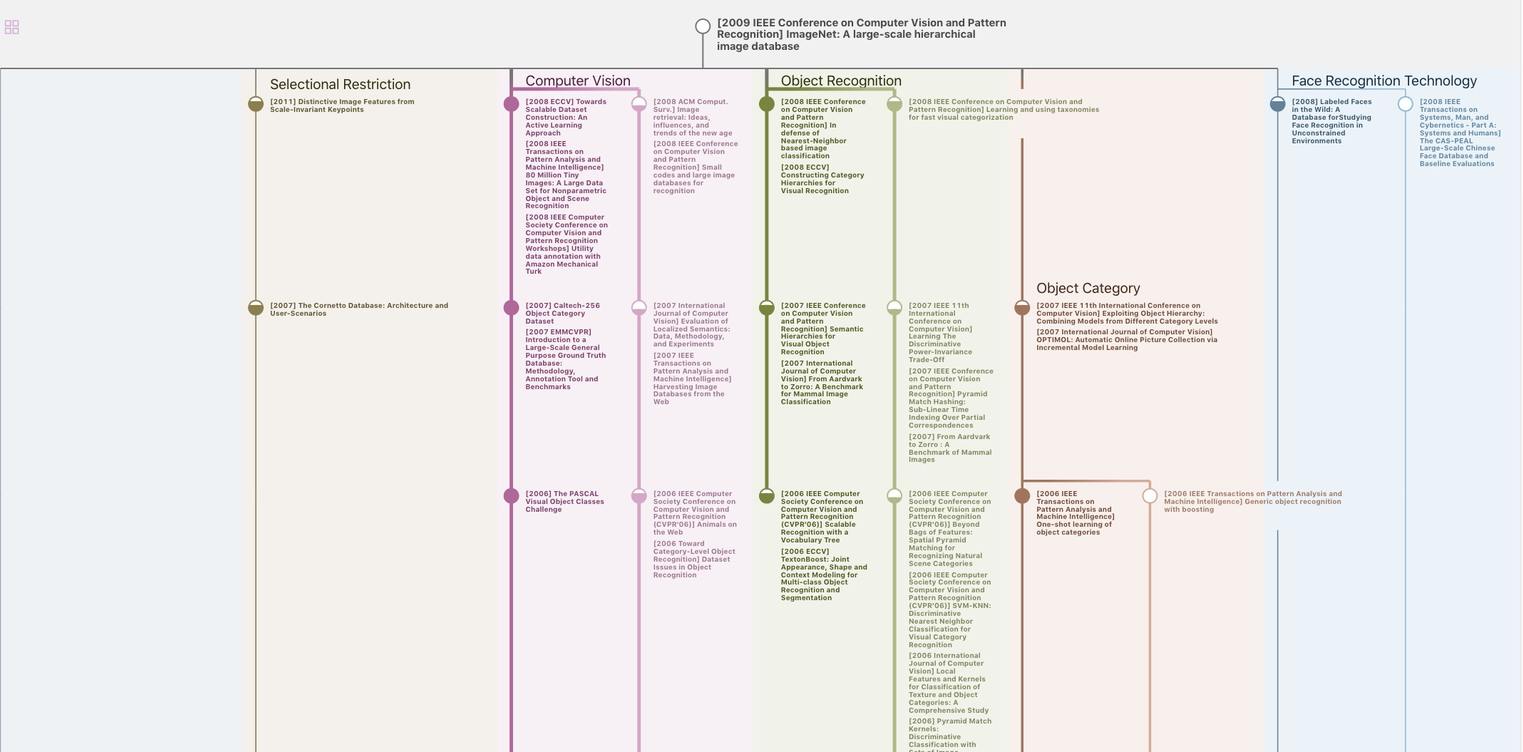
生成溯源树,研究论文发展脉络
Chat Paper
正在生成论文摘要