High temporal resolution urban flood prediction using attention-based LSTM models
JOURNAL OF HYDROLOGY(2023)
摘要
Rapid and accurate urban flood forecasting with high temporal resolution is critical to address future flood risks under urbanization and climate change. Machine learning models are increasingly used for flood forecasting, however, they are limited in their ability to extract effective features from rainfall data and capture dynamic runoff processes. This study proposes an attention mechanism-based Long Short-Term Memory (LSTM) network, named as ALSTM-DW, which uses double time sliding windows (DTSW), and a weighted mean square error (WMSE) loss function. The ALSTM-DW model was applied to three urban flooding hotspots in Shenzhen, China, and its effectiveness was verified through a series of comparative experiments. The results obtained show that: 1) the proposed model performs well, with a coefficient of determination larger than 0.85, peak flow error smaller than 0.015 m, and time to peak error smaller than 2 min on average in testing periods; 2) the use of DTSW helps extract effective rainfall features and mitigate excessive fluctuations in forecasted hydrographs caused by overresponse to rainfall; 3) adding the attention mechanism to the LSTM network helps consider the varying contribution of each input factor over time; and 4) the WMSE improves the ability of the network to predict flood peaks, with an average decrease of 88% and 54% in peak flow error and time to peak error, respectively. Therefore, the ALSTM-DW model shows promising potential for providing accurate flood forecasting, which can support effective flood emergency operations.
更多查看译文
关键词
urban flood prediction,high temporal resolution,attention-based
AI 理解论文
溯源树
样例
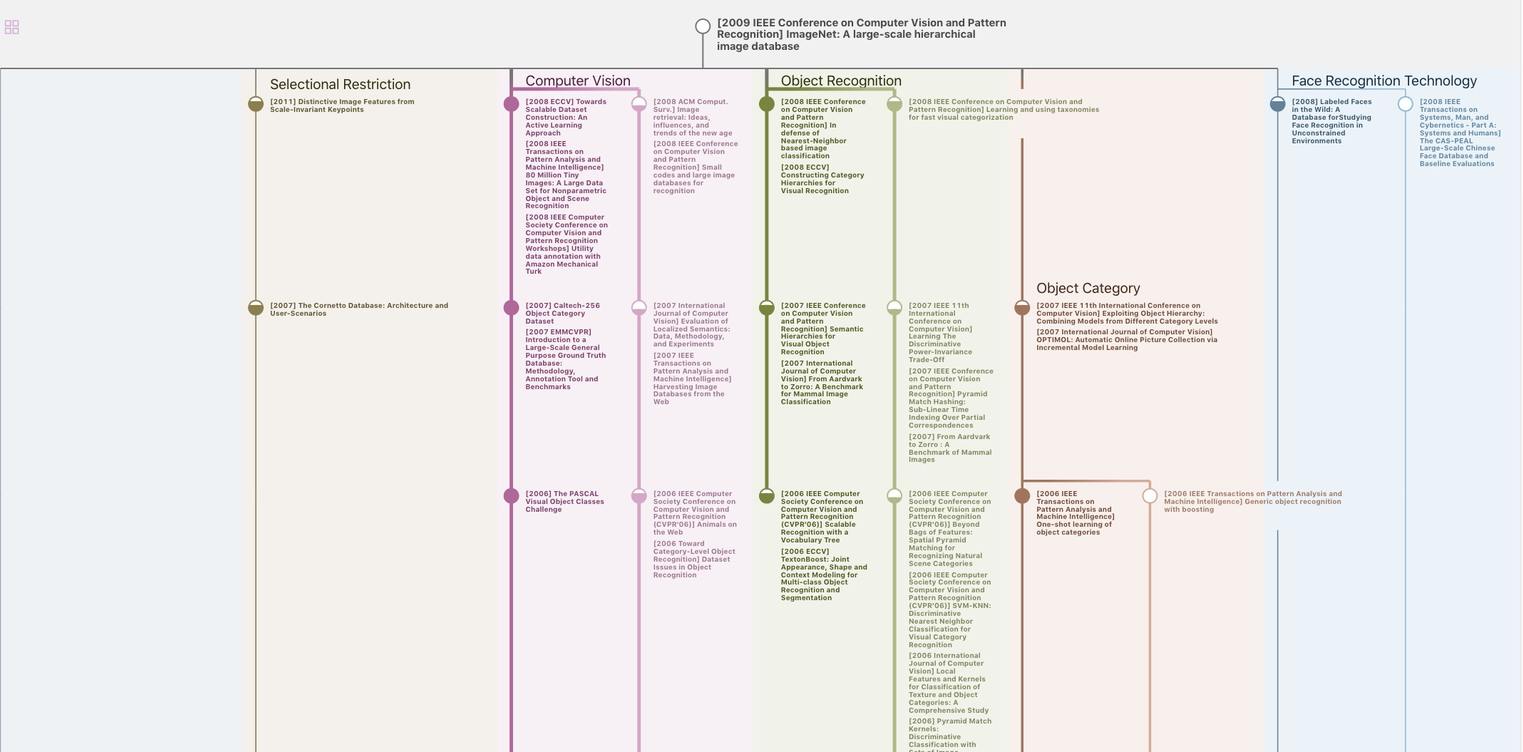
生成溯源树,研究论文发展脉络
Chat Paper
正在生成论文摘要